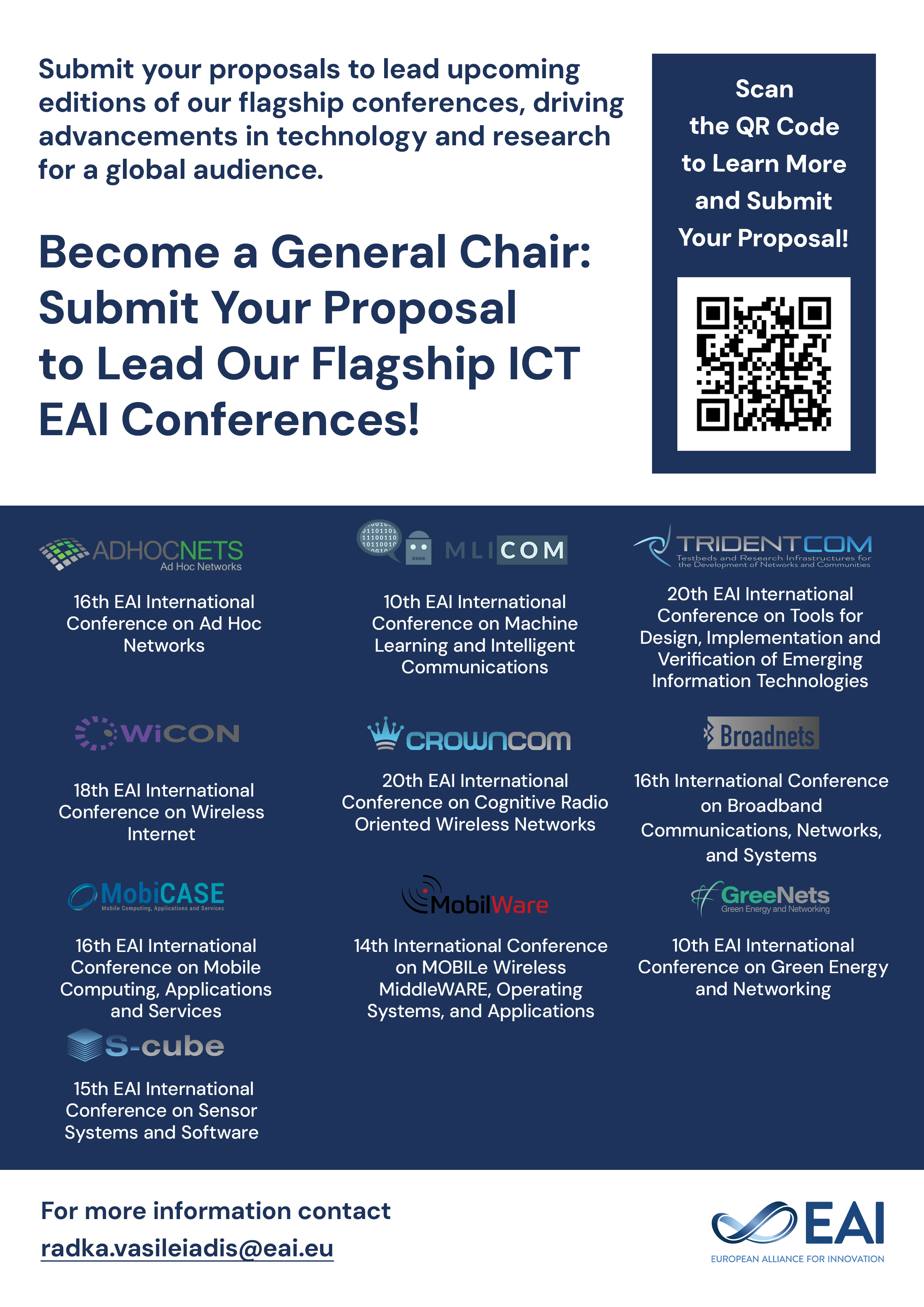
Research Article
Mining DNS for malicious domain registrations
@INPROCEEDINGS{10.4108/icst.collaboratecom.2010.5, author={Yuanchen He and Zhenyu Zhong and Sven Krasser and Yuchun Tang}, title={Mining DNS for malicious domain registrations}, proceedings={6th International ICST Conference on Collaborative Computing: Networking, Applications, Worksharing}, publisher={IEEE}, proceedings_a={COLLABORATECOM}, year={2011}, month={5}, keywords={Decision trees Helium Machine learning Manuals Markov processes Vegetation}, doi={10.4108/icst.collaboratecom.2010.5} }
- Yuanchen He
Zhenyu Zhong
Sven Krasser
Yuchun Tang
Year: 2011
Mining DNS for malicious domain registrations
COLLABORATECOM
ICST
DOI: 10.4108/icst.collaboratecom.2010.5
Abstract
Millions of new domains are registered every day and the many of them are malicious. It is challenging to keep track of malicious domains by only Web content analysis due to the large number of domains. One interesting pattern in legitimate domain names is that many of them consist of English words or look like meaningful English while many malicious domain names are randomly generated and do not include meaningful words. We show that it is possible to transform this intuitive observation into statistically informative features using second order Markov models. Four transition matrices are built from known legitimate domain names, known malicious domain names, English words in a dictionary, and based on a uniform distribution. The probabilities from these Markov models, as well as other features extracted from DNS data, are used to build a Random Forest classifier. The experimental results demonstrate that our system can quickly catch malicious domains with a low false positive rate.