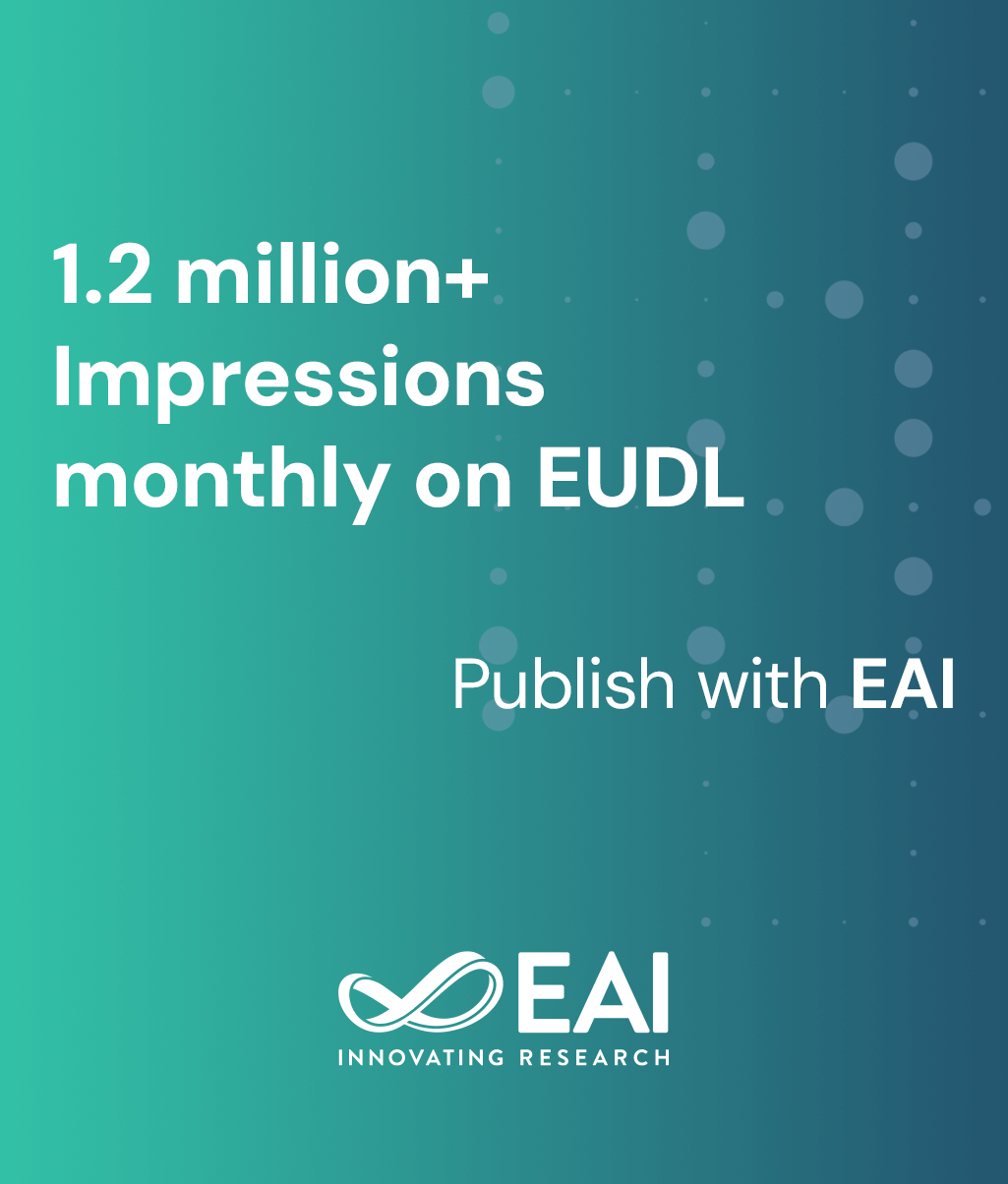
Research Article
Face Recognition Based on 4 Dimensions Local Binary Patterns
@INPROCEEDINGS{10.4108/icst.chinacom.2014.256439, author={Chao Wang and Jucheng Yang and Yarui Chen and Chao Wu and Song Dong and Xiaoyuan Zhang}, title={Face Recognition Based on 4 Dimensions Local Binary Patterns}, proceedings={9th International Conference on Communications and Networking in China}, publisher={IEEE}, proceedings_a={CHINACOM}, year={2015}, month={1}, keywords={lbp 3dlbp 4dlbp face recognition elm}, doi={10.4108/icst.chinacom.2014.256439} }
- Chao Wang
Jucheng Yang
Yarui Chen
Chao Wu
Song Dong
Xiaoyuan Zhang
Year: 2015
Face Recognition Based on 4 Dimensions Local Binary Patterns
CHINACOM
IEEE
DOI: 10.4108/icst.chinacom.2014.256439
Abstract
In face recognition, LBP (Local Binary Patterns) is a very popular method, which can solve the defects of the traditional local feature extraction methods with fixed scale and small extraction scale. However, the LBP operator only describes the relationship between the center pixel and its neighborhood pixels, it ignores the relationship among the operators. 3DLBP (3 Dimensions Local Binary Patterns) operator embodies this relationship to get a better local description. However, both of them neglect the center pixel value, which also reflects some properties of the image points. In this paper, we propose a novel face recognition method based on 4DLBP (4 Dimensions Local Binary Patterns), which adds the pixel value of the center point into the 3DLBP features for face recognition. We firstly partition the face image into small blocks, and then we extract the 4DLBP features of the blocked images, and combine the features to obtain the final facial features. Finally, we use the extreme learning machine (ELM) as classifier to train and classify the face images. The experimental results show the proposed method has better performances than the traditional methods.