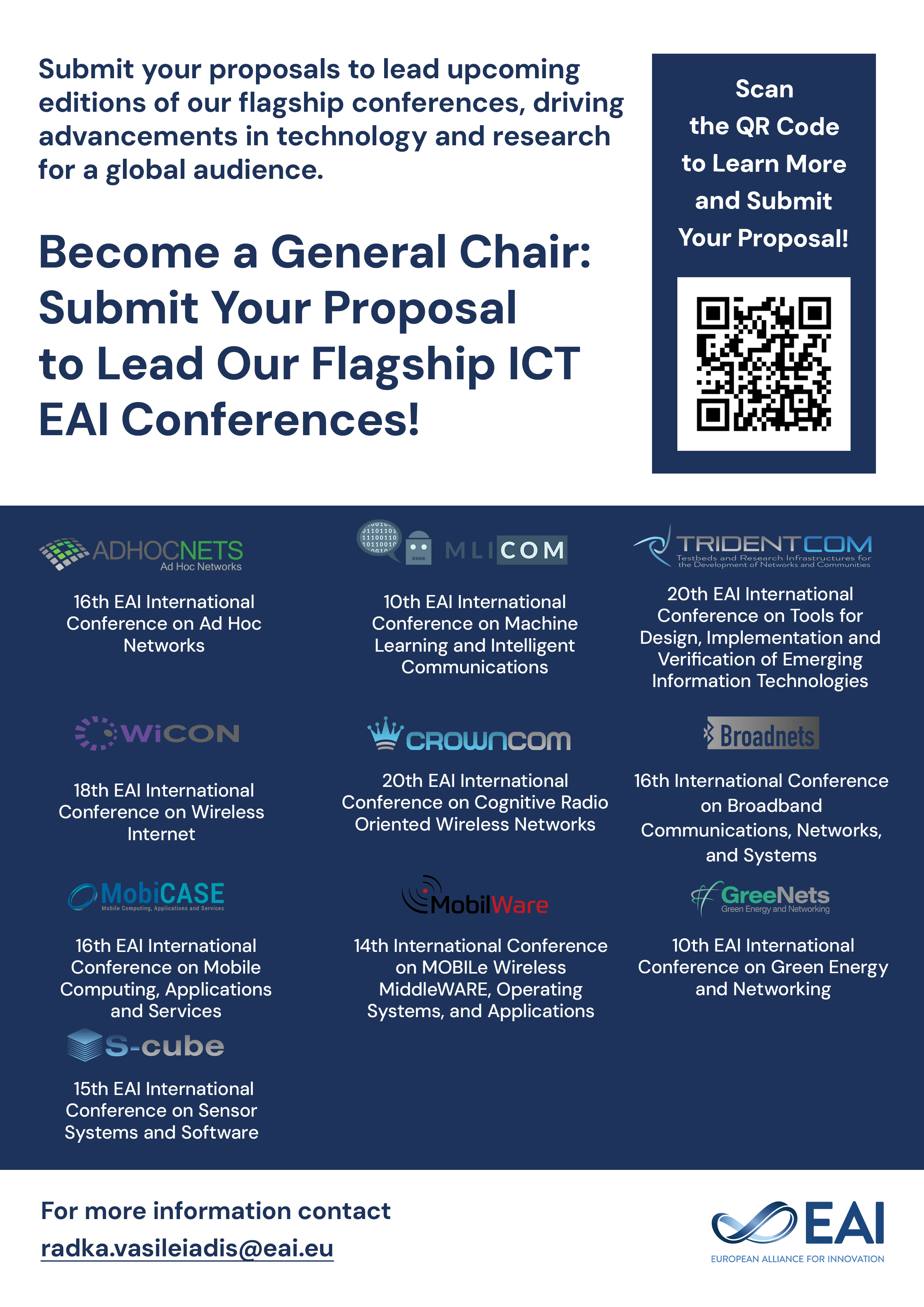
Research Article
Wireless Body Sensor for Objective Assessment of Surgical Performance on a Standardised FLS Task
@INPROCEEDINGS{10.4108/icst.bodynets.2014.257019, author={Georgina Kirby and Richard Kwasnicki and Caroline Hargrove and Jonathan Rees and Mikael Sodergren and Guang-Zhong Yang and Benny Lo}, title={Wireless Body Sensor for Objective Assessment of Surgical Performance on a Standardised FLS Task}, proceedings={9th International Conference on Body Area Networks}, publisher={ICST}, proceedings_a={BODYNETS}, year={2014}, month={11}, keywords={surgical dexterity machine learning body sensor networks inertial sensors}, doi={10.4108/icst.bodynets.2014.257019} }
- Georgina Kirby
Richard Kwasnicki
Caroline Hargrove
Jonathan Rees
Mikael Sodergren
Guang-Zhong Yang
Benny Lo
Year: 2014
Wireless Body Sensor for Objective Assessment of Surgical Performance on a Standardised FLS Task
BODYNETS
ACM
DOI: 10.4108/icst.bodynets.2014.257019
Abstract
Advances in Body Sensor Networks have prompted increasing numbers of low cost, miniaturised sensors being used in many applications with one being the capture of hand movement data for surgical skills assessment. Despite these advances, existing assessments are still predominantly subjective and resource demanding. Combining surgical training with a reliable objective assessment technique would ensure that trainees are correctly evaluated and credentialed as they progress through their training hence, ensuring competence and reducing critical medical errors. This paper proposes the use of wearable, wireless inertial sensors for capturing motion data and enabling objective assessment of trainee surgeons' performance in carrying out one of the FLS (Fundamentals of Laparoscopic surgery) tasks; the peg transfer. A novel approach has been developed for segmenting specific peg movements enabling performance to be measured entirely objectively. The features derived from the whole task and from segmented peg movements were analysed through unsupervised machine learning algorithms to look for useful measures of performance as well as patterns to identify differences between expert and trainee performance. Encouraging results in the peg transfer task, where a successful classification of expertise was obtained for participants against gold standard assessment, prompt further investigation into the development of advanced performance metrics for a wider range of surgical tasks.