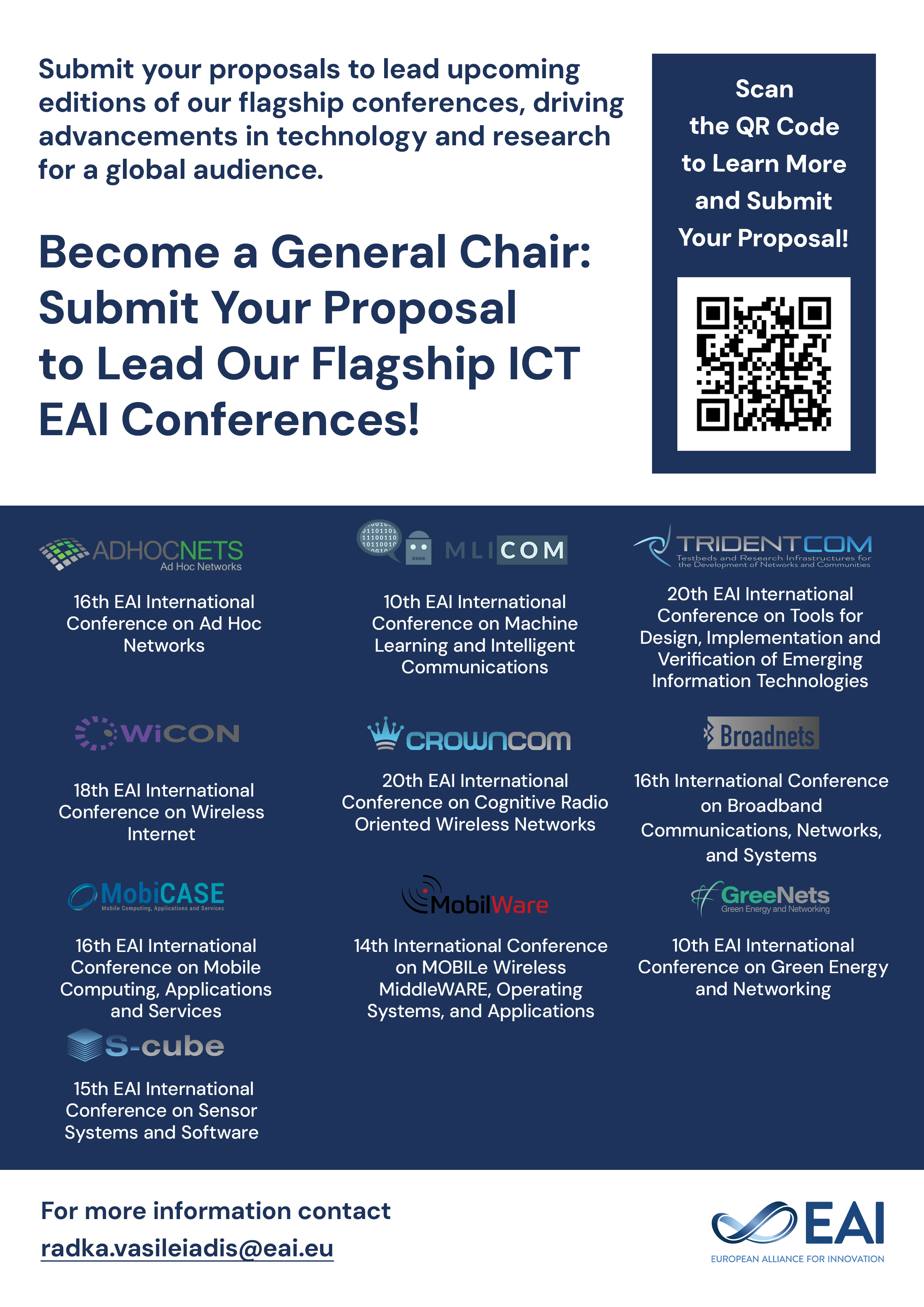
Research Article
Fusing On-Body Sensing with Local and Temporal Cues for Daily Activity Recognition
@INPROCEEDINGS{10.4108/icst.bodynets.2014.257014, author={Zack Zhu and Ulf Blanke and Alberto Calatroni and Oliver Brdiczka and Gerhard Tr\o{}ster}, title={Fusing On-Body Sensing with Local and Temporal Cues for Daily Activity Recognition}, proceedings={9th International Conference on Body Area Networks}, publisher={ICST}, proceedings_a={BODYNETS}, year={2014}, month={11}, keywords={activity routine recognition wearable sensors web repository exploitation crowd-sensing platform}, doi={10.4108/icst.bodynets.2014.257014} }
- Zack Zhu
Ulf Blanke
Alberto Calatroni
Oliver Brdiczka
Gerhard Tröster
Year: 2014
Fusing On-Body Sensing with Local and Temporal Cues for Daily Activity Recognition
BODYNETS
ACM
DOI: 10.4108/icst.bodynets.2014.257014
Abstract
Automatically recognizing people’s daily activities is essential for a variety of applications, such as just-in-time content delivery or quantified self-tracking. Towards this, researchers often use customized wearable motion sensors tailored to recognize a small set of handpicked activities in controlled environments. In this paper, we design and engineer a scalable, daily activity recognition framework, by leveraging two widely adopted commercial devices: Android smartphone and Pebble smartwatch. Deploying our system outside the laboratory, we collected a total of more than 72 days of data from 12 user study participants. We systematically show the usefulness of time, location, and wrist-based motion for automatically recognizing 10 standardized activities, as specified by the American Time Use Survey taxonomy. Overall, we achieve a recognition accuracy of 76.28% for personalized models and 69.80% for generic, interpersonal models.