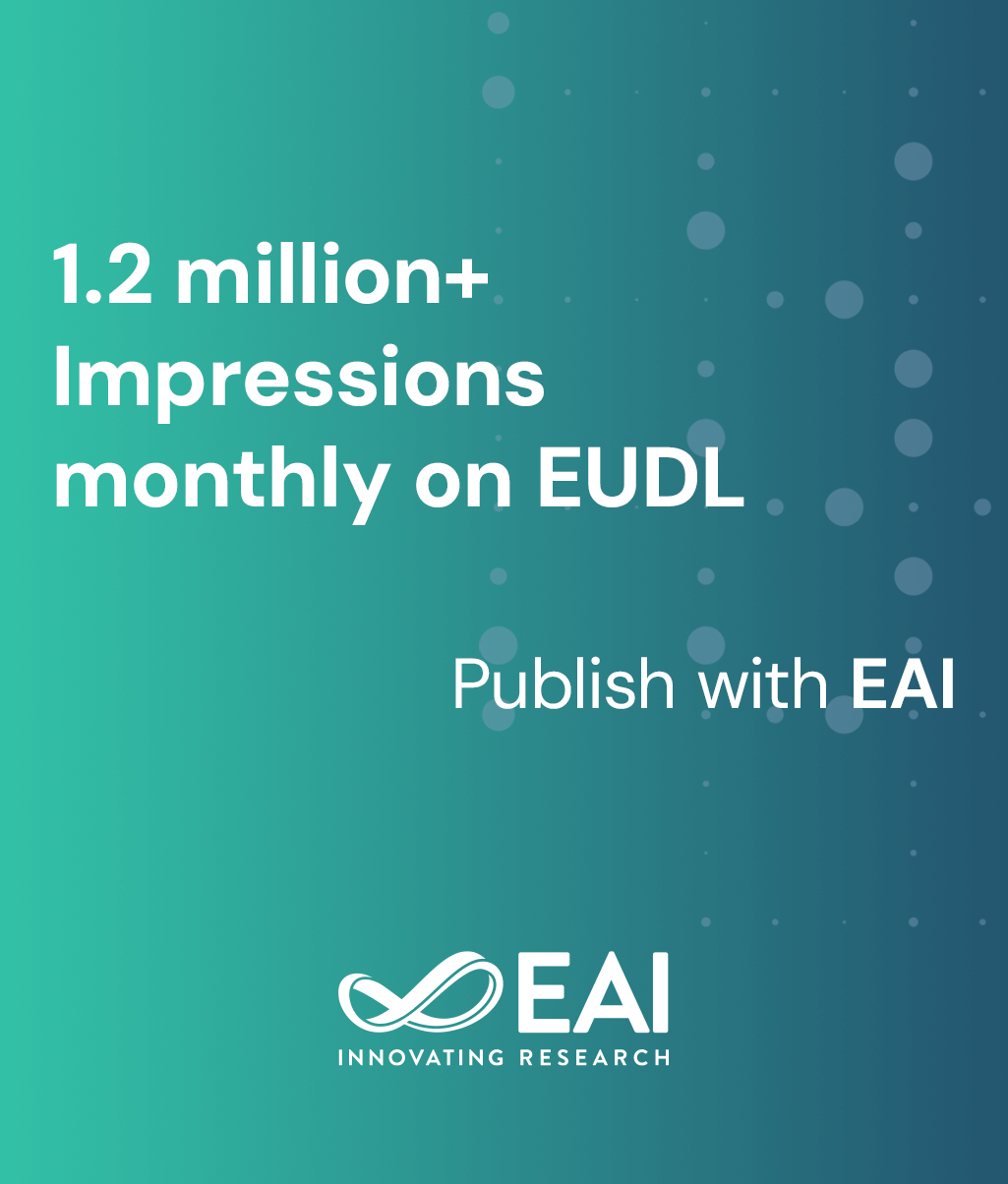
Research Article
Nonlinear Feature for Gait Speed Estimation using Inertial Sensors
@INPROCEEDINGS{10.4108/icst.bodynets.2013.253737, author={Shanshan Chen and John Lach}, title={Nonlinear Feature for Gait Speed Estimation using Inertial Sensors}, proceedings={8th International Conference on Body Area Networks}, publisher={ICST}, proceedings_a={BODYNETS}, year={2013}, month={10}, keywords={phase portrait; gait speed; feature selection; machine learning}, doi={10.4108/icst.bodynets.2013.253737} }
- Shanshan Chen
John Lach
Year: 2013
Nonlinear Feature for Gait Speed Estimation using Inertial Sensors
BODYNETS
ACM
DOI: 10.4108/icst.bodynets.2013.253737
Abstract
Gait speed is an important feature in many health applications. To obtain this information, a machine-learning approach is often preferred to first principle modeling for its generality in dealing with systematic errors. In this approach, extracting predictive features is critical to the estimation accuracy. Therefore, identifying and extracting highly correlated features for gait speed estimation become the first important steps for the machine-learning framework. This paper proposes a novel nonlinear feature for gait speed estimation using shank-mounted inertial sensors. Rooted in analytic mechanics, this nonlinear feature captures the dynamics of angular position and angular velocity – two interdependent variables for gait speed – simultaneously by examining the area of the phase portrait. Among all the features extracted, this nonlinear feature was ranked as the most important by an automatic feature selection algorithm given its highest correlation with gait speed among all features evaluated, and it improves the accuracy for gait speed estimation in intra-subject cross validation compared to using commonly extracted linear features alone.