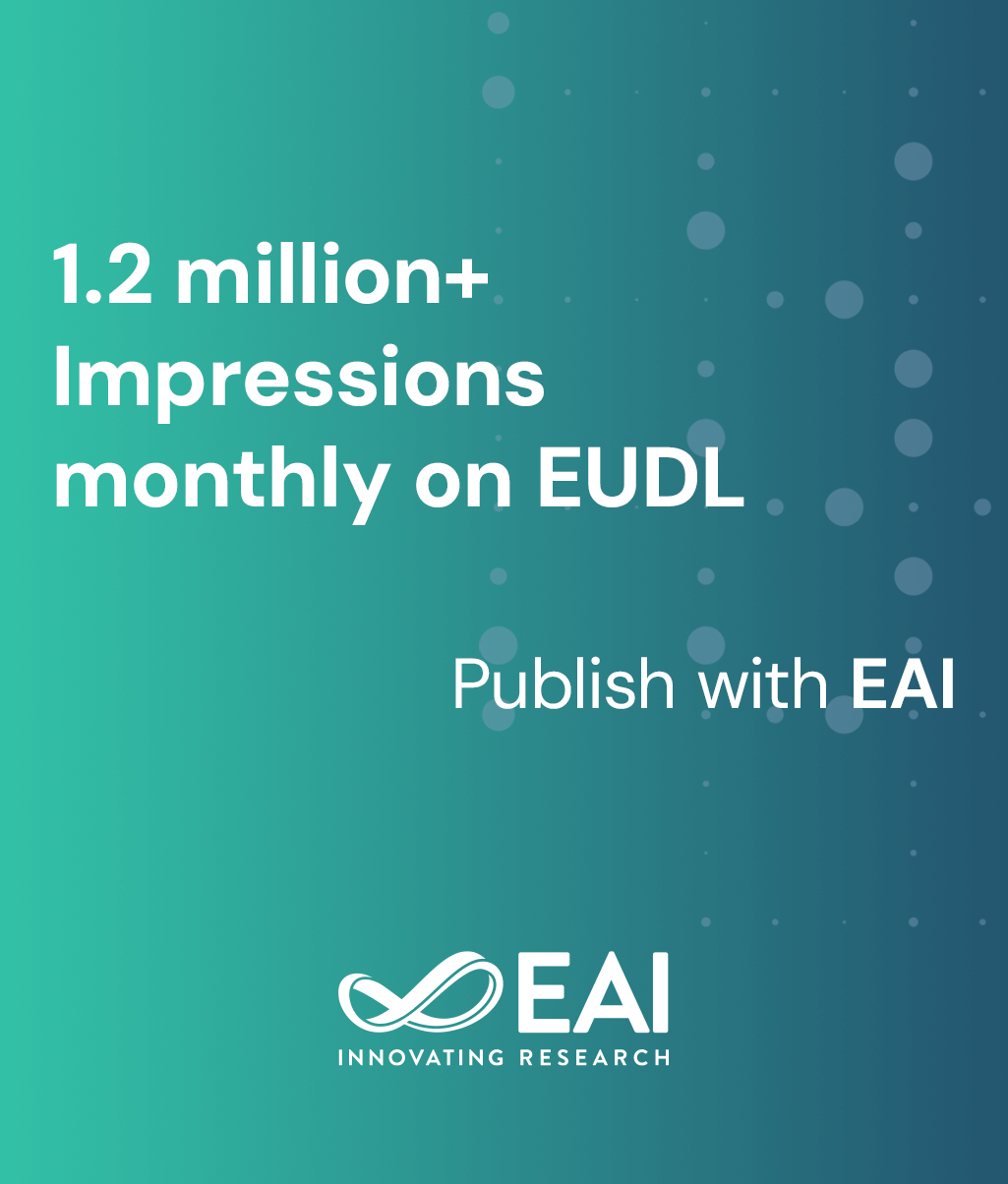
Research Article
Using Second Order Learning to Evolve Social Representation (Theory of Mind)
@INPROCEEDINGS{10.4108/icst.bict.2014.257889, author={Solvi Arnold and Reiji Suzuki and Takaya Arita}, title={Using Second Order Learning to Evolve Social Representation (Theory of Mind)}, proceedings={8th International Conference on Bio-inspired Information and Communications Technologies (formerly BIONETICS)}, publisher={ICST}, proceedings_a={BICT}, year={2015}, month={2}, keywords={evolution of mind representation theory of mind neural networks social evolution modularity}, doi={10.4108/icst.bict.2014.257889} }
- Solvi Arnold
Reiji Suzuki
Takaya Arita
Year: 2015
Using Second Order Learning to Evolve Social Representation (Theory of Mind)
BICT
ACM
DOI: 10.4108/icst.bict.2014.257889
Abstract
We demonstrate how basic social representation ability can be evolved in neural networks. Agents are evolved to perform a base behaviour and predict the actions of a partner performing the base behaviour. For the base behaviour agents pick actions in accordance with the environment and their mental state (a bit string). When predicting others' actions agents see the environment but not the mental state of the partner, so this task requires agents to observe the partner agent and learn. A neuromodulation mechanism is introduced to allow for evolution of the requisite learning ability. We run two sets of experiments. In the first, the mental state of the partner is constant over the duration of the interaction. This produces a first order learning task (essentially supervised learning). In the second, the mental state of the partner changes frequently, one bit at a time. This produces a second order learning task (unsolvable with supervised learning). Analysis shows that nets evolved using the second order task learn by decoding the partner's mental state from its behaviour. Connections in these nets specialize on individual bits of the partner's mental state, allowing us to read individual bits of knowledge about the other agents' minds. Such bit-level representation of mental states is not observed in nets evolved using the first order learning task. This supports the idea that evolutionary selection for second order learning is selection for representational cognition.