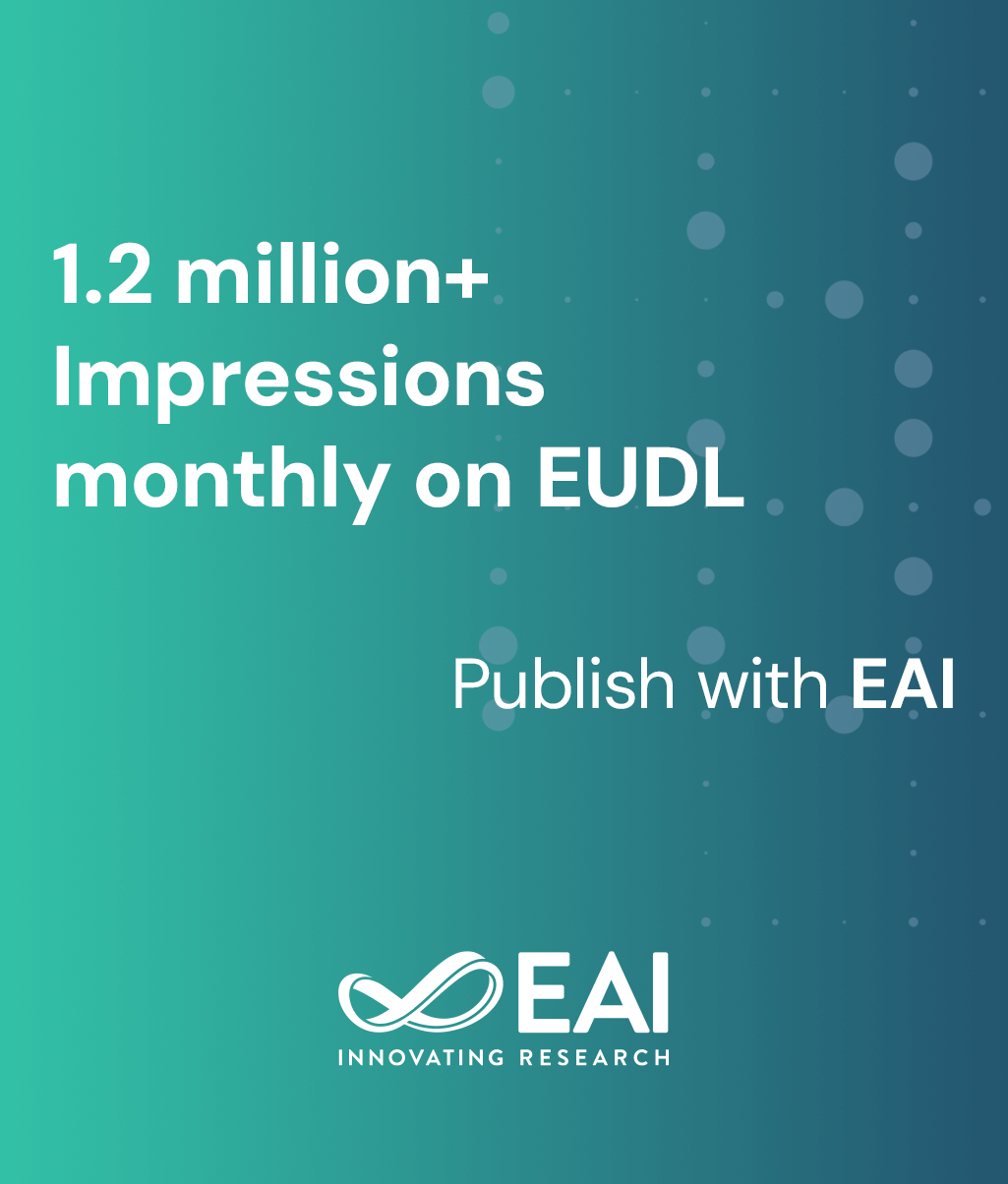
Research Article
An Empirical Study on Classification of Monkeypox Skin Lesion Detection
@ARTICLE{10.4108/eetpht.v8i5.3352, author={B. V. CHANDRAHAAS and Sachi Nandan Mohanty and Sujit Kumar Panda and Michael G.}, title={An Empirical Study on Classification of Monkeypox Skin Lesion Detection}, journal={EAI Endorsed Transactions on Pervasive Health and Technology}, volume={9}, number={1}, publisher={EAI}, journal_a={PHAT}, year={2023}, month={5}, keywords={Deep learning, Disease diagnosis, Image processing, Monkeypox virus, Machine learning, Transfer learning, CNN models, Computer vision architectures}, doi={10.4108/eetpht.v8i5.3352} }
- B. V. CHANDRAHAAS
Sachi Nandan Mohanty
Sujit Kumar Panda
Michael G.
Year: 2023
An Empirical Study on Classification of Monkeypox Skin Lesion Detection
PHAT
EAI
DOI: 10.4108/eetpht.v8i5.3352
Abstract
INTRODUCTION: After the covid-19 outbreak, Monkeypox has become a global pandemic putting people’s lives in jeopardy. Monkeypox has become a major concern in 40+ countries apart from Africa as scientists are struggling to clinically diagnose the virus as it looks similar with chickenpox and measles. As a part of our research, we found that to get the clinically tested result of monkey pox through polymerase chain reaction (PCR) test would take 3-4 days which is a lengthy process. OBJECTIVES: The objective of this paper is to provide a rapid identification solution which can instantly detect monkeypox virus with the help of computer vision architectures. This can be considered for preliminary examination of skin lesions and help the victim isolate themselves so that they would be cautious and can stop the spreading of virus. METHODS: Many studies have been conducted to identify the monkeypox with the help of Deep Learning models but in this study, we compare the test results obtained by deep learning CNN models AlexNet, GoogLeNet using transfer learning approach and determine the efficient model[2]. RESULTS: Testing the algorithms by changing the batch sizes and number of epochs we have obtained a highest accuracy of 83.61% for AlexNet and 82.64% for GoogLeNet. CONCLUSION: AlexNet was outperforming GoogLeNet architecture in terms of validation accuracy thus providing better results.
Copyright © 2023 B. V. Chandrahaas et al., licensed to EAI. This is an open access article distributed under the terms of the CC BYNC-SA 4.0, which permits copying, redistributing, remixing, transformation, and building upon the material in any medium so long as the original work is properly cited.