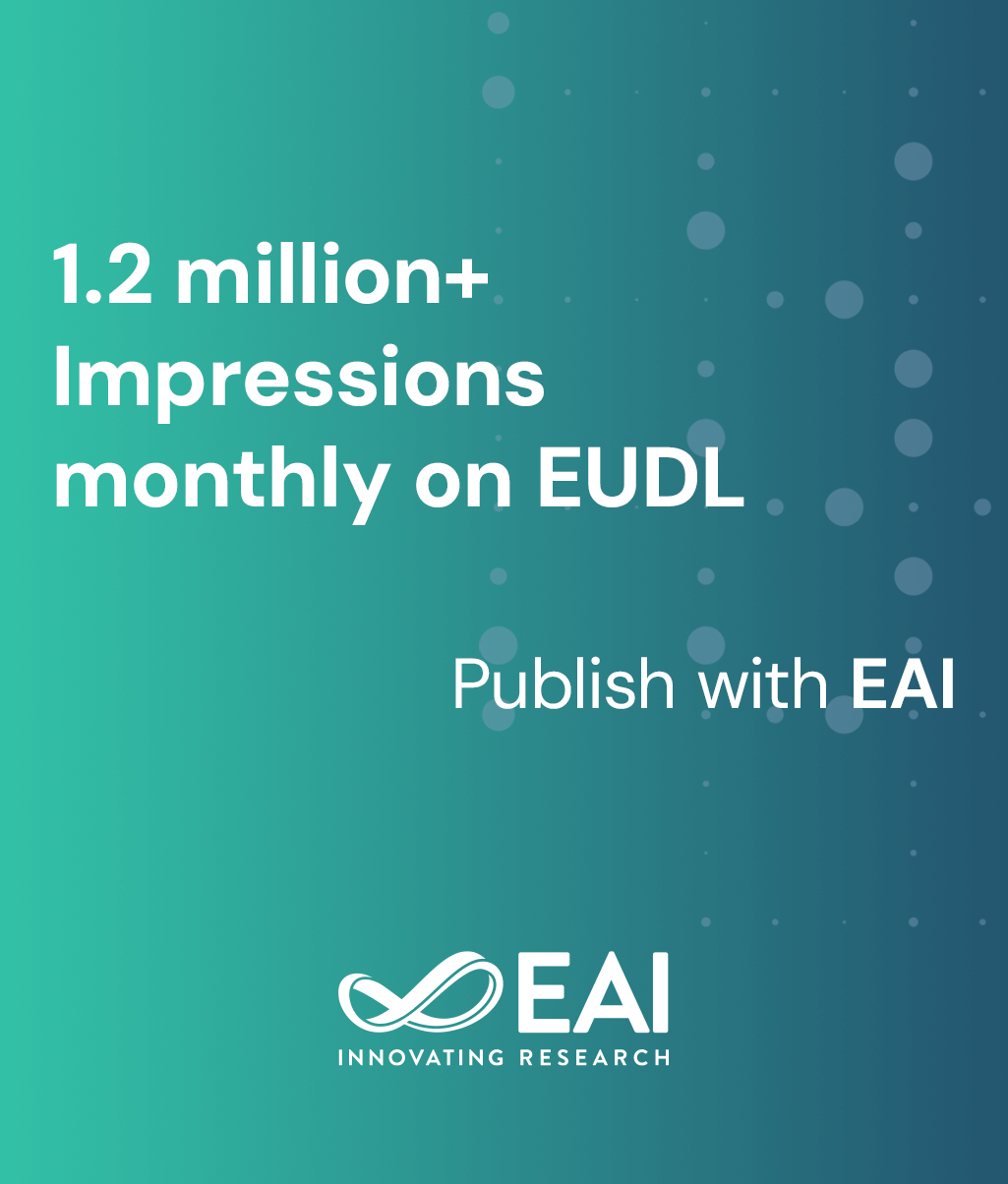
Research Article
Prediction of Emergency Mobility Under Diverse IoT Availability
@ARTICLE{10.4108/eetpht.v8i4.274, author={Bin Sun and Renkang Geng and Yuan Xu and Tao Shen}, title={Prediction of Emergency Mobility Under Diverse IoT Availability}, journal={EAI Endorsed Transactions on Pervasive Health and Technology}, volume={8}, number={4}, publisher={EAI}, journal_a={PHAT}, year={2022}, month={9}, keywords={Emergency Traffic, IoT Time Series, Data Quantity, Data Quality, Machine Learning Prediction}, doi={10.4108/eetpht.v8i4.274} }
- Bin Sun
Renkang Geng
Yuan Xu
Tao Shen
Year: 2022
Prediction of Emergency Mobility Under Diverse IoT Availability
PHAT
EAI
DOI: 10.4108/eetpht.v8i4.274
Abstract
INTRODUCTION: Prediction of emergency mobility needs to consider more scenarios as Internet of Things (IoT) develops at a high speed, which influences the quality and quantity of data, manageable resources and algorithms. OBJECTIVES: This work investigates differences in dynamic emergency mobility prediction when facing dynamic temporal IoT data with different quality and quantity considering diverse computing resources and algorithm availability. METHODS: A node construction scheme under a small range of traffic networks is adopted in this work, which can effectively convert the road to graph network structure data which has been proved to be feasible and used for the small-scale traffic network data here. Besides, two different datasets are formed using public large scale traffic network data. Representative widely used and proven algorithms from typical types of methods are selected respectively with different datasets to conduct experiments. RESULTS: The experimental results show that the graphed data and neural network algorithm can deal with the dynamic time series data with complex nodes and edges in a better way, while the non-neural network algorithm can predict the with a simple graph network structure. CONCLUSION: Our proposed graph construction with graph neural network improves dynamic emergency mobility prediction. The prediction should consider the scenarios of availability of computing resources, quantity and quality of data among other IoT features to improve the results. Later, automation and data enrichment should be improved.
Copyright © 2022 Bin Sun et al., licensed to EAI. This is an open access article distributed under the terms of the CC BY-NC-SA 4.0, which permits copying, redistributing, remixing, transformation, and building upon the material in any medium so long as the original work is properly cited.