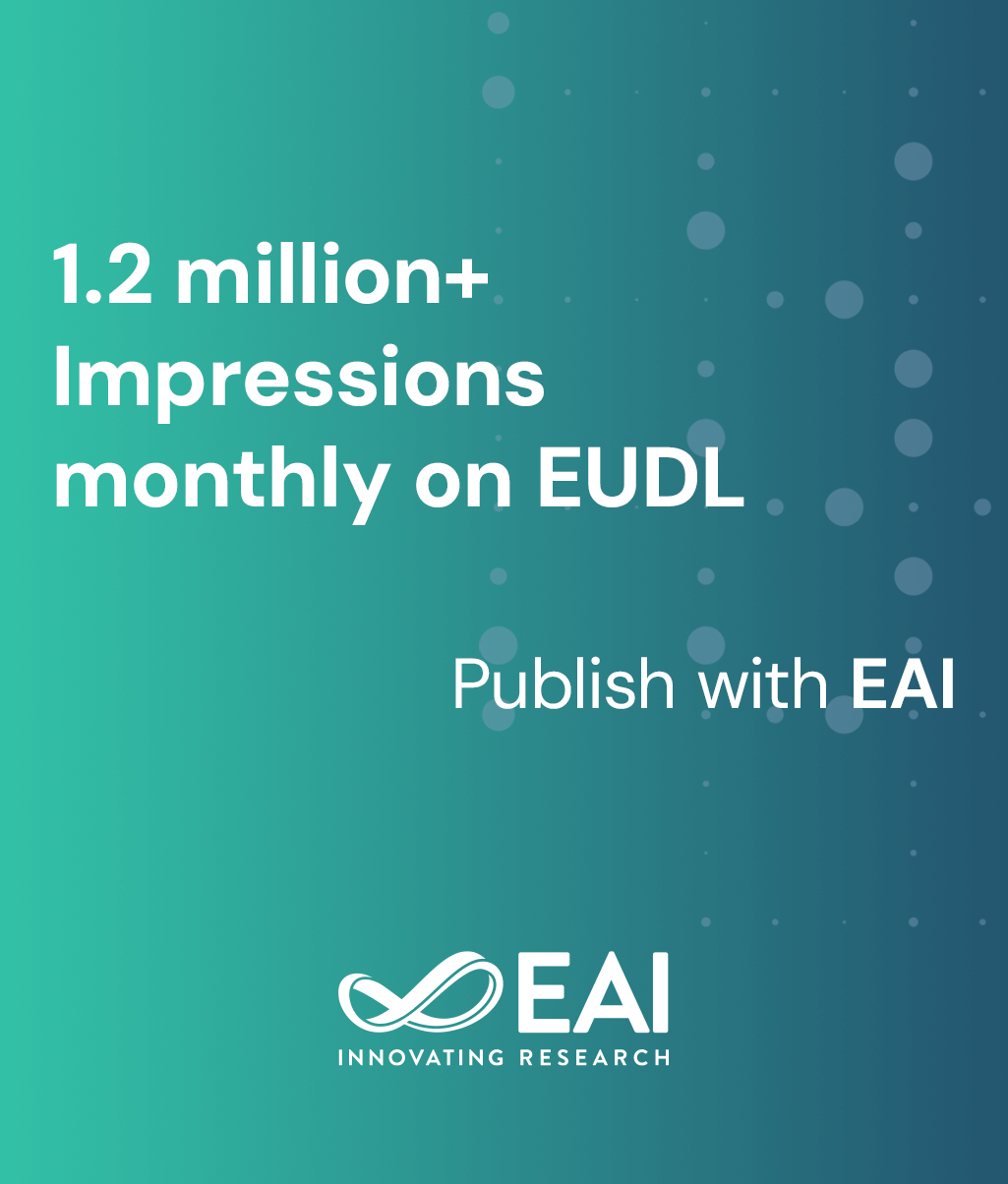
Research Article
Advanced Hybrid Model for Multi Paddy diseases detection using Deep Learning
@ARTICLE{10.4108/eetpht.9.4481, author={Atul Kumar Dixit and Rajat Verma}, title={Advanced Hybrid Model for Multi Paddy diseases detection using Deep Learning}, journal={EAI Endorsed Transactions on Pervasive Health and Technology}, volume={9}, number={1}, publisher={EAI}, journal_a={PHAT}, year={2023}, month={11}, keywords={Rice Plant, CNN, Leaf diseases, DCNN, DL, SVM, Transfer learning, Deep learning}, doi={10.4108/eetpht.9.4481} }
- Atul Kumar Dixit
Rajat Verma
Year: 2023
Advanced Hybrid Model for Multi Paddy diseases detection using Deep Learning
PHAT
EAI
DOI: 10.4108/eetpht.9.4481
Abstract
INTRODUCTION: Rapid developments in deep learning (DL) techniques have made it possible to find and recognize objects in pictures. To create a network that is significantly more successful than a single CNN, GAN, RNN, etc., we can mix various neural network models (CNN, GAN, RNN).this combination is known as hybrid model. Hybrid model of deep leaning is give more accurately result for detection and identification of paddy diseases. OBJECTIVES: I have studies outcome of hybrid model 1(DCNN+SVM) and Hybrid model 2 (DCNN + Transfer Learning) to increase accuracy of Rice plant disease detection and classification. The Researched model detects multiple rice plant diseases and it is giving same result in multiple data sets. METHODS: The Proposed System have used Deep Learning Image Processing algorithm and neural Network Like DCNN ,SVM and Transfer Learning .The brand new model is DST where D stands for DCNN, S stands for SVM and T stands for transfer learning. RESULTS: The Researched DST model achieved 95% Training accuracy and 85% validation Accuracy. The Researched model detect multiple rice plant diseases and it is giving same result in multiple data set. CONCLUSION: The proposed model combined 2 existing model and developed hybrid model that a detect various rice plant diseases with better accuracy from available existing model.
Copyright © 2023 A. K. Dixit et al., licensed to EAI. This is an open access article distributed under the terms of the CC BY-NC-SA 4.0, which permits copying, redistributing, remixing, transformation, and building upon the material in any medium so long as the original work is properly cited.