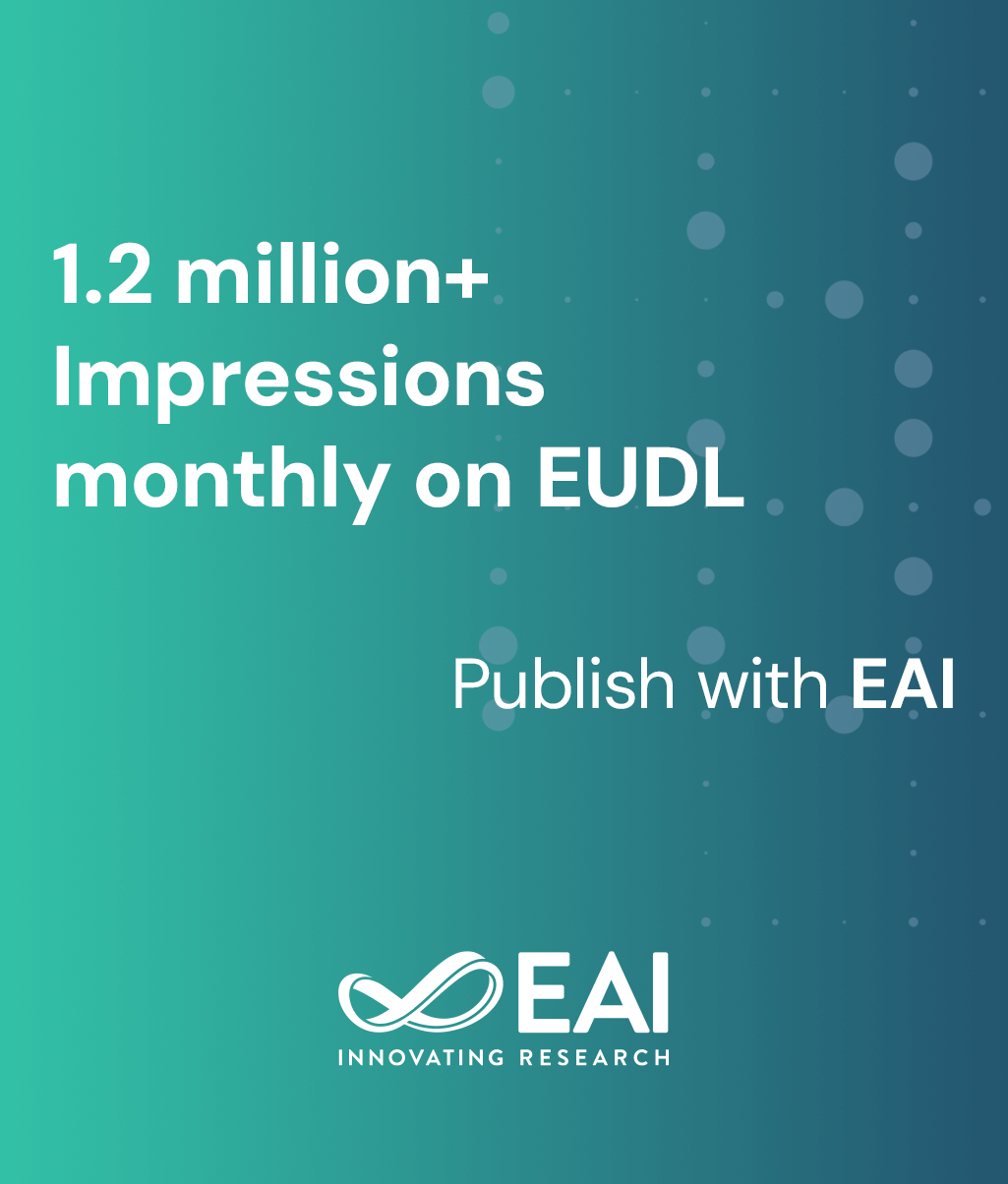
Research Article
Melanoma Skin Cancer Detection using SVM and CNN
@ARTICLE{10.4108/eetpht.9.4340, author={Sai Pranav Kothapalli and Panchumarthi Sri Hari Priya and Vempada Sagar Reddy and Botta Lahya and Prashanth Ragam}, title={Melanoma Skin Cancer Detection using SVM and CNN}, journal={EAI Endorsed Transactions on Pervasive Health and Technology}, volume={9}, number={1}, publisher={EAI}, journal_a={PHAT}, year={2023}, month={11}, keywords={Skin Cancer Detection, Machine Learning, Image Processing, Deep Learning, Convolutional Neural Networks, Support Vector Machine}, doi={10.4108/eetpht.9.4340} }
- Sai Pranav Kothapalli
Panchumarthi Sri Hari Priya
Vempada Sagar Reddy
Botta Lahya
Prashanth Ragam
Year: 2023
Melanoma Skin Cancer Detection using SVM and CNN
PHAT
EAI
DOI: 10.4108/eetpht.9.4340
Abstract
In the field of cancer detection and prevention, doctors and patients are facing numerous challenges when it comes to cancer prediction. Melanoma skin cancer is a deadly type of skin cancer with a multitude of variants spread across the world. Traditional methods involved manual inspection followed by various tests of samples. This time-consuming work and inaccurate predictions sometimes risk the overall health of the patient. The two aspects of solving skin cancer detection problems utilising both conventional image-processing techniques and methods based on machine learning and deep learning are elaborated in this article. It gives a review of current skin cancer detection techniques, weighs the benefits and drawbacks of those techniques, and introduces some relevant cancer datasets. The proposed method focuses mainly on Melanoma skin cancer detection and its previous stages (Common Nevus and Atypical Nevus). The methods being proposed employ a blend of colour, texture, and shape characteristics to derive distinguishing attributes from the images. Using CNN (convolutional neural networks) and SVM (support vector machine) algorithms to identify the type of skin cancer the patient is affected with and achieved an accuracy of 92% and 95% respectively.
Copyright © 2023 S. P. Kothapalli et al., licensed to EAI. This is an open access article distributed under the terms of the CC BY-NCSA 4.0, which permits copying, redistributing, remixing, transformation, and building upon the material in any medium so long as the original work is properly cited.