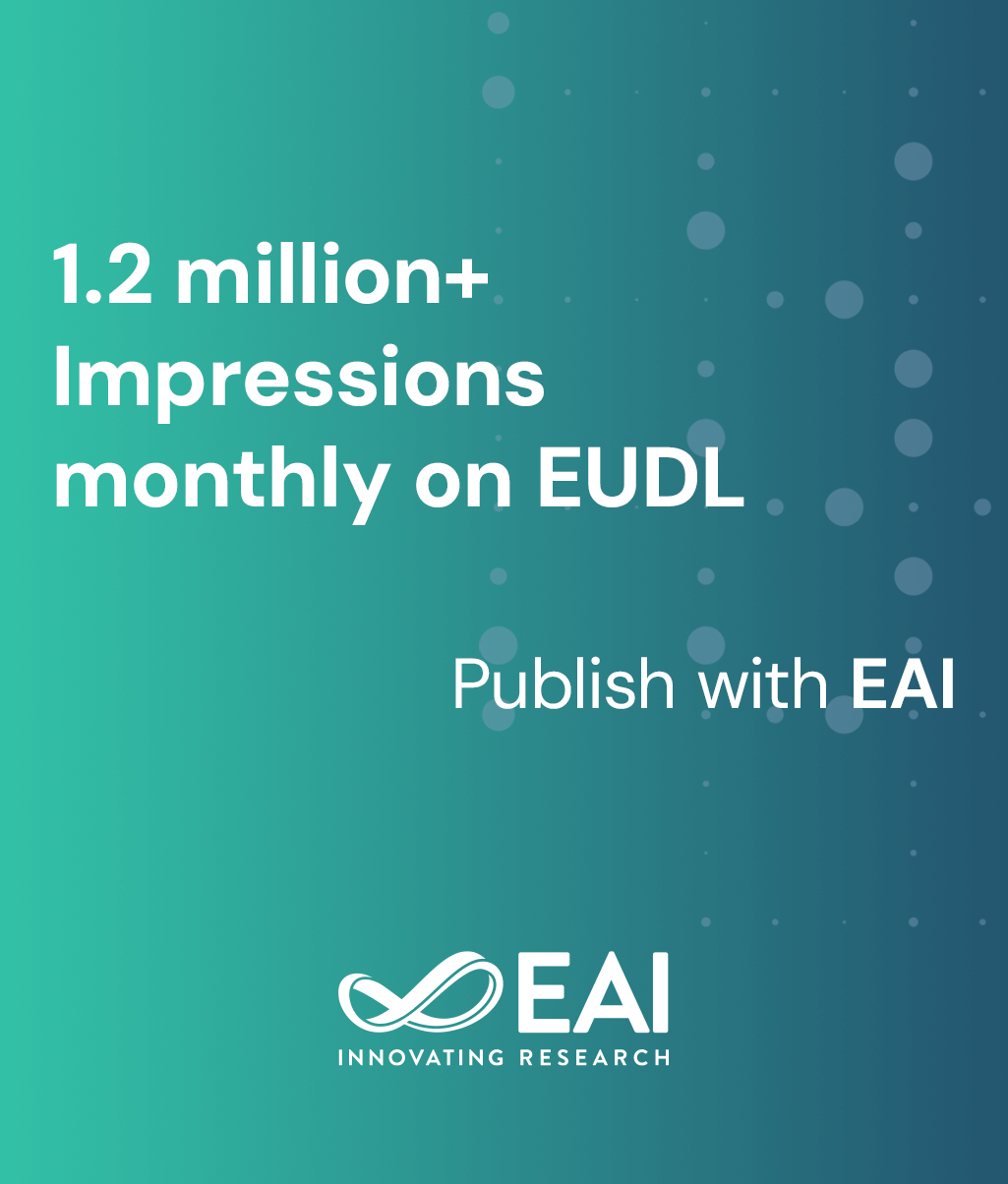
Research Article
Enhancing Image Recognition: Leveraging Machine Learning on Specialized Medical Datasets
@ARTICLE{10.4108/eetpht.9.4336, author={Nidhi Agarwal and Nitish Kumar and Anushka and Vrinda Abrol and Yashica Garg}, title={Enhancing Image Recognition: Leveraging Machine Learning on Specialized Medical Datasets}, journal={EAI Endorsed Transactions on Pervasive Health and Technology}, volume={9}, number={1}, publisher={EAI}, journal_a={PHAT}, year={2023}, month={11}, keywords={adversarial attacks, computational resources, Convolutional Neural Networks, CNNs, image recognition, machine learning}, doi={10.4108/eetpht.9.4336} }
- Nidhi Agarwal
Nitish Kumar
Anushka
Vrinda Abrol
Yashica Garg
Year: 2023
Enhancing Image Recognition: Leveraging Machine Learning on Specialized Medical Datasets
PHAT
EAI
DOI: 10.4108/eetpht.9.4336
Abstract
INTRODUCTION: Image recognition plays a pivotal role in numerous industries, ranging from healthcare to autonomous vehicles. Machine learning techniques, especially deep learning algorithms, have revolutionized the field of image recognition by enabling computers to identify and classify objects within images with high accuracy. OBJECTIVES: This research paper provides an in-depth exploration of the application of machine learning algorithms for image recognition tasks, including supervised learning, convolutional neural networks (CNNs), and transfer learning. METHODS: The paper discusses the challenges associated with image recognition, such as dataset size and quality, overfitting, and computational resources. RESULTS: It highlights emerging trends and future research directions, including explainability and interpretability, adversarial attacks and robustness, and real-time and edge-based recognition. CONCLUSION: In conclusion, the study emphasizes the transformative impact of deep learning algorithms, addressing challenges in image recognition. Ongoing focus on emerging trends is vital for enhancing accuracy and efficiency in diverse applications.
Copyright © 2023 N. Agarwal et al., licensed to EAI. This is an open access article distributed under the terms of the CC BY-NC-SA 4.0, which permits copying, redistributing, remixing, transformation, and building upon the material in any medium so long as the original work is properly cited.