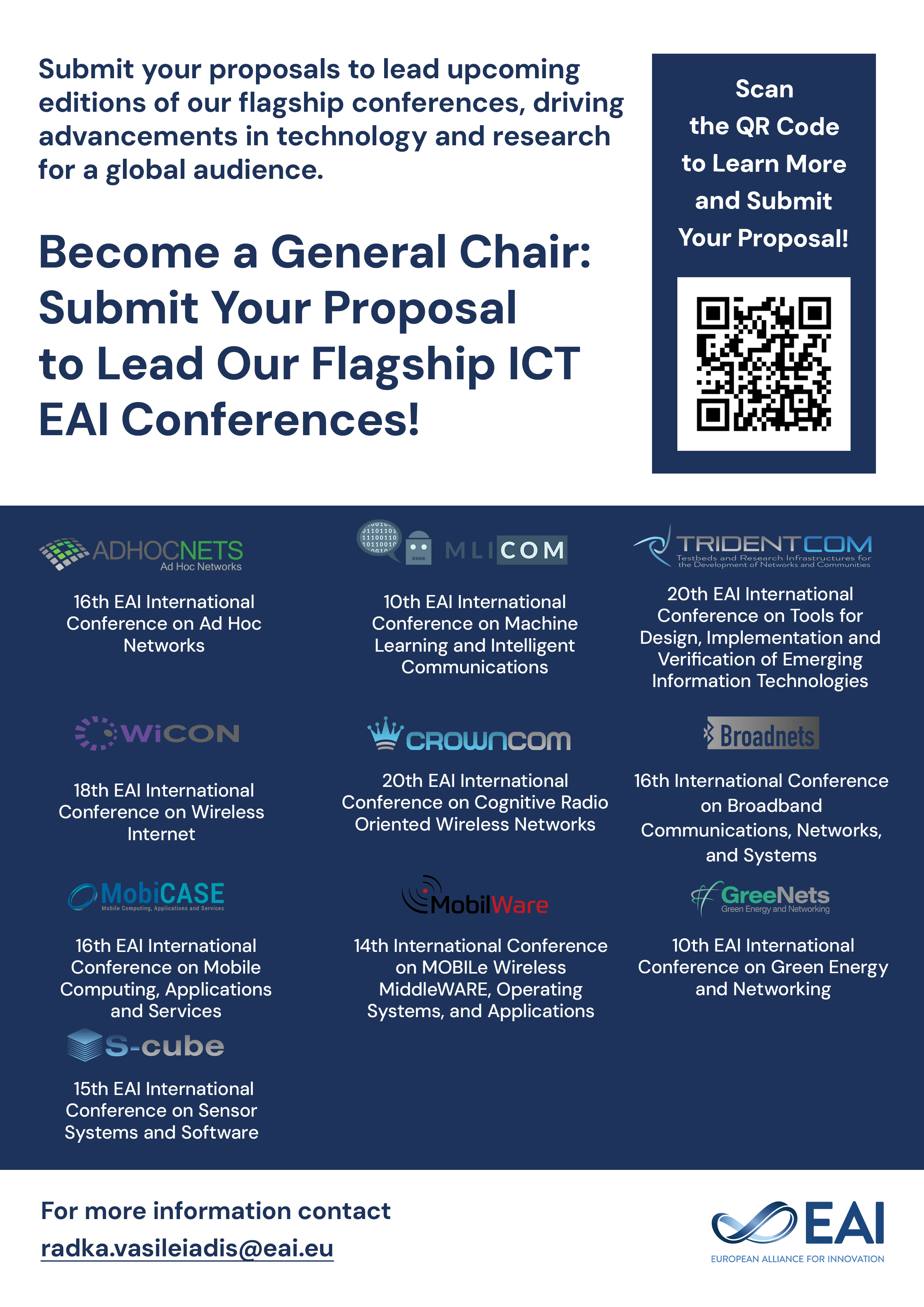
Research Article
Reinforced Hybrid Graph Transformer for Medical Recommendations
@ARTICLE{10.4108/eetpht.9.4285, author={Anil V Turukmane and Sagar Pande and Vaidehi Bedekar and Aditya Kadam}, title={Reinforced Hybrid Graph Transformer for Medical Recommendations}, journal={EAI Endorsed Transactions on Pervasive Health and Technology}, volume={9}, number={1}, publisher={EAI}, journal_a={PHAT}, year={2023}, month={10}, keywords={Disease symptom diagnosis knowledge graph, DSDKG, graph neural network with attention mechanism, GAT, generative pretrained transformer 2, GPT2, reinforced learning environment}, doi={10.4108/eetpht.9.4285} }
- Anil V Turukmane
Sagar Pande
Vaidehi Bedekar
Aditya Kadam
Year: 2023
Reinforced Hybrid Graph Transformer for Medical Recommendations
PHAT
EAI
DOI: 10.4108/eetpht.9.4285
Abstract
An enormous amount of heterogeneous Textual Medical Knowledge (TMK), which is crucial to healthcare information systems, has been produced by the explosion of healthcare information. Existing efforts to incorporate and use textual medical knowledge primarily concentrate on setting up simple links and pay less attention to creating computers comprehend information accurately and rapidly. Self-diagnostic symptom checkers and clinical decision support systems have seen a significant rise in demand in recent years. Existing systems rely on knowledge bases that are either automatically generated using straightforward paired statistics or manually constructed through a time-consuming procedure. The study explored process to learn textual data, linking disease and symptoms from web-based documents. Medical concepts were scrapped and collected from different web-based sources. The research aims to generate a disease- symptom-diagnosis knowledge graph (DSDKG), with the help of web-based documents. Moreover, the knowledge graph is fed in to Graph neural network with Attention Mechanism (GAT) for learning the nodes and edges relationships. . Lastly Generative Pretrained Transformer 2 (GPT2) all enclosed in a Reinforced learning environment, is used on the trained model to generate text based recommendations.
Copyright © 2023 A. V. Turukmane et al., licensed to EAI. This is an open access article distributed under the terms of the CC BYNC-SA 4.0, which permits copying, redistributing, remixing, transformation, and building upon the material in any medium so long as the original work is properly cited.