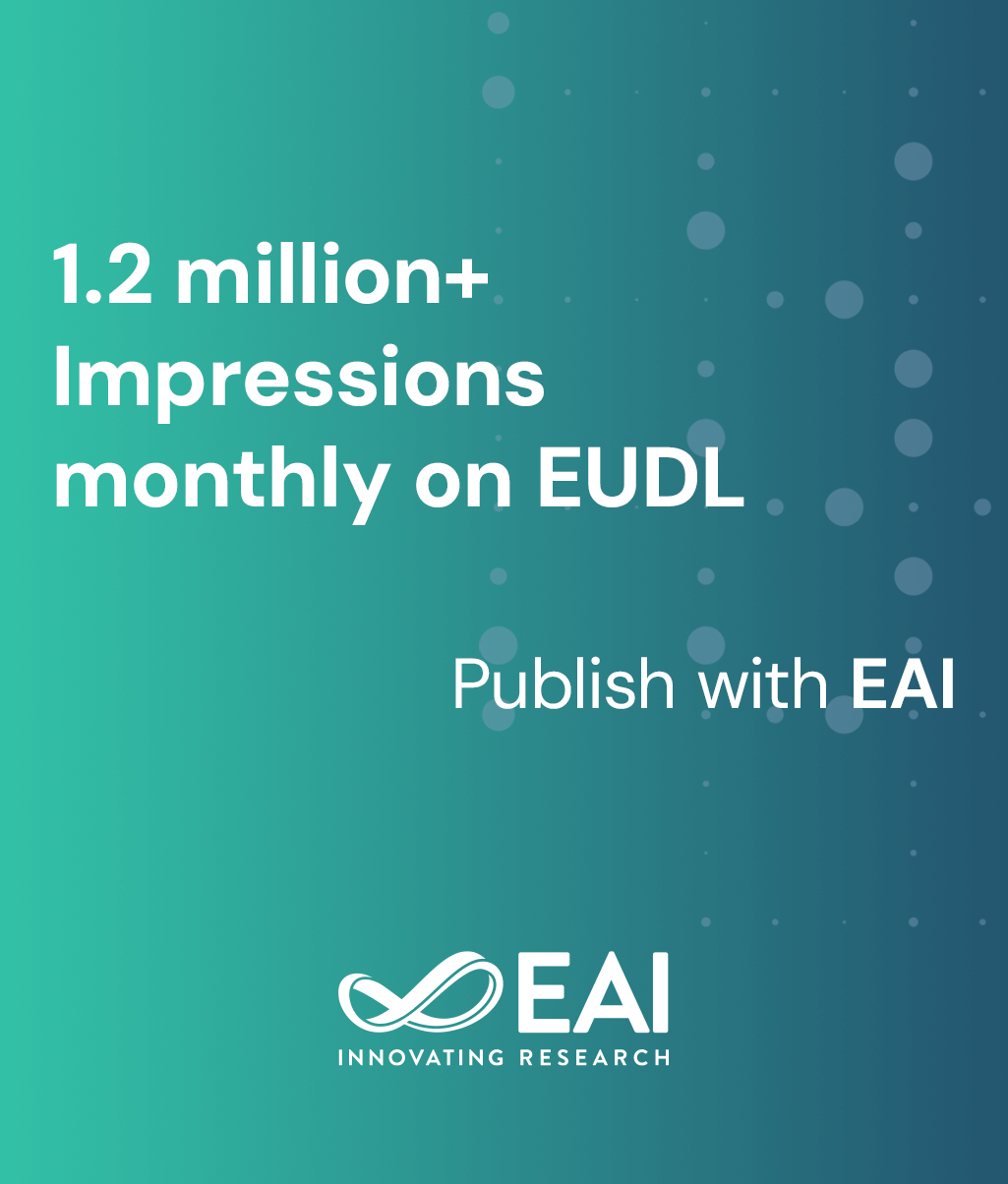
Research Article
Neural Networks for the Diagnosis of Covid-19 in Chest X-ray Images: A Systematic Review and Meta-Analysis
@ARTICLE{10.4108/eetpht.9.4212, author={Daniel Cristobal Andrade-Gir\^{o}n and William Joel Mar\^{\i}n-Rodriguez and Flor de Mar\^{\i}a Lioo-Jord\^{a}n and Gladis Jane Villanueva-Cadenas and Flor de Mar\^{\i}a Garivay-Torres de Salinas}, title={Neural Networks for the Diagnosis of Covid-19 in Chest X-ray Images: A Systematic Review and Meta-Analysis}, journal={EAI Endorsed Transactions on Pervasive Health and Technology}, volume={9}, number={1}, publisher={EAI}, journal_a={PHAT}, year={2023}, month={10}, keywords={prediction, COVID-19, model VGG16, deep learning}, doi={10.4108/eetpht.9.4212} }
- Daniel Cristobal Andrade-Girón
William Joel Marín-Rodriguez
Flor de María Lioo-Jordán
Gladis Jane Villanueva-Cadenas
Flor de María Garivay-Torres de Salinas
Year: 2023
Neural Networks for the Diagnosis of Covid-19 in Chest X-ray Images: A Systematic Review and Meta-Analysis
PHAT
EAI
DOI: 10.4108/eetpht.9.4212
Abstract
Introduction: The COVID-19 pandemic has triggered a global crisis with significant repercussions in terms of mortality and an ever-increasing demand for urgent medical care, particularly in emergency care settings. This demand arises mainly from the prevailing need to carry out real-time diagnoses and provide immediate care to patients at high risk of serious complications. With the purpose of addressing this problem in a rigorous manner, we have carried out a systematic review focused on evaluating the effectiveness of models based on neural networks for the diagnosis of COVID-19 from chest x-ray images. Methods: This review has been carried out through an exhaustive search in various renowned electronic bibliographic databases, such as Scopus, IEEE Xplore, PubMed and ScienceDirect. The search period has been extended until September 2023, culminating in the identification of a total of 1,250 relevant articles. Results: The culminating phase of our review involved the inclusion of 37 studies that met rigorously established selection criteria. These studies have been the subject of a thorough analysis, where various performance metrics such as accuracy/precision, sensitivity/recall, specificity and the F1 value (F1-score) have been evaluated. Conclusions: Our results reveal that the VGG16 (Visual Geometry Group 16) model, based on neural networks, has emerged as the most widely adopted, manifesting itself in 13.04% of the total models analyzed and in 16.21% of the models supported by the 37 studies. selected. Notably, this algorithm has exhibited an impressive accuracy of over 99% in predicting the diagnosis of patients with suspected COVID-19.
Copyright © 2023 Andrade-Girón et al., licensed to EAI. This is an open access article distributed under the terms of the CC BY-NCSA 4.0, which permits copying, redistributing, remixing, transformation, and building upon the material in any medium so long as the original work is properly cited.