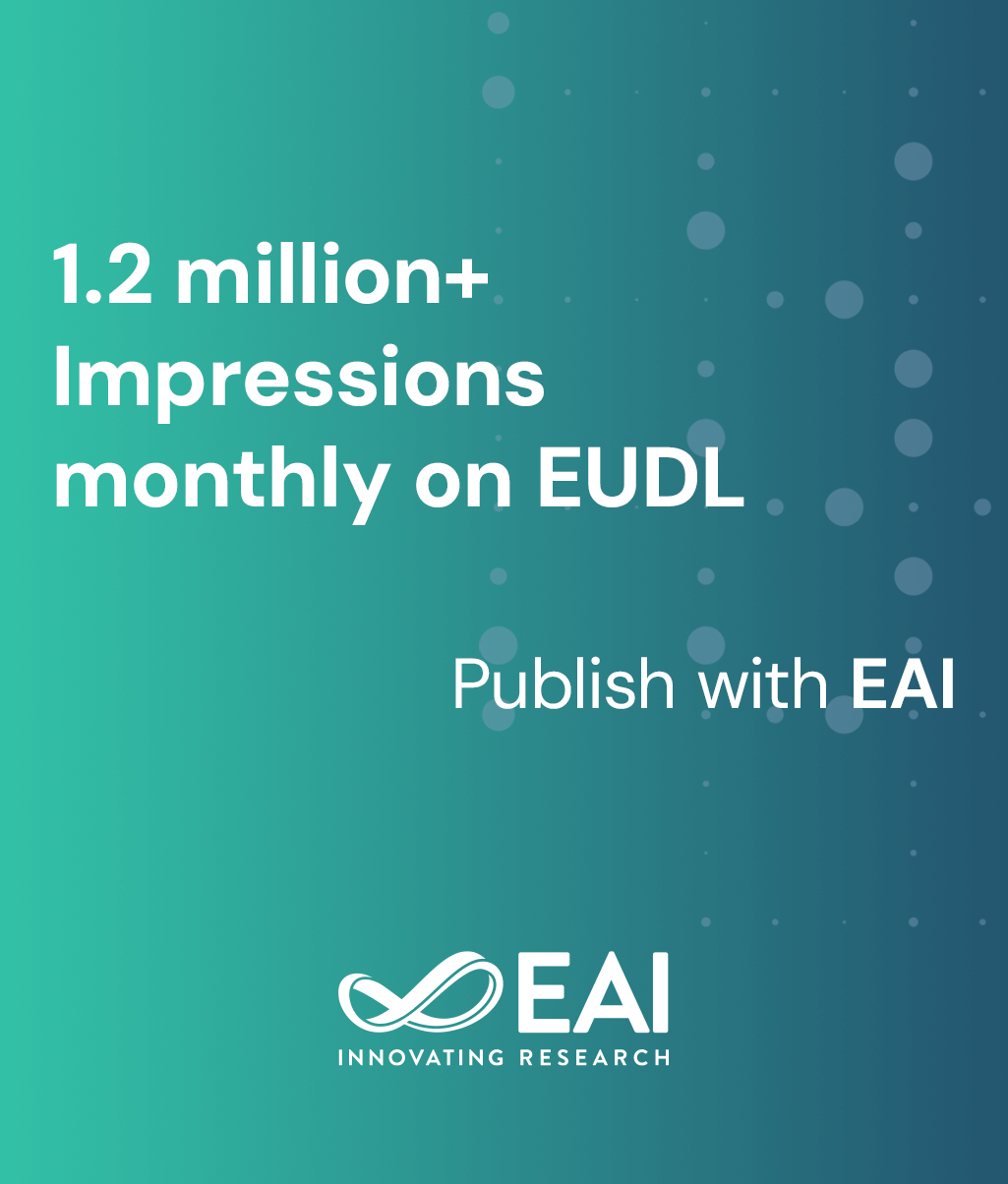
Research Article
Stacking Model for Heart Stroke Prediction using Machine Learning Techniques
@ARTICLE{10.4108/eetpht.9.4057, author={Subasish Mohapatra and Indrani Mishra and Subhadarshini Mohanty}, title={Stacking Model for Heart Stroke Prediction using Machine Learning Techniques}, journal={EAI Endorsed Transactions on Pervasive Health and Technology}, volume={9}, number={1}, publisher={EAI}, journal_a={PHAT}, year={2023}, month={10}, keywords={Feature Selection, Heart Stroke, Machine Learning, Random Forest, RF, Navies Bayes, NB, Logistic Regression, LR, Decision Tree, DT, Adaboost, K- Nearest- Neighbor, Gradient Boosting, GB}, doi={10.4108/eetpht.9.4057} }
- Subasish Mohapatra
Indrani Mishra
Subhadarshini Mohanty
Year: 2023
Stacking Model for Heart Stroke Prediction using Machine Learning Techniques
PHAT
EAI
DOI: 10.4108/eetpht.9.4057
Abstract
The paper presents an adaptive model that utilized the machine learning algorithms to predict the heart diseases. As heart disease is one of the leading causes of death and understanding its mechanism, effective prevention, diagnosis, and treatment is very crucial. With the help of data analytics, machine learning, artificial intelligence, it is possible to provide optimal solution to the heart diseases. But still getting optimal accuracy is a challenging issue. Identifying the data pattern, correlation and algorithms affects the accuracy very much. In this work, a stacking model has been proposed to find the best models out of it and validate the model for better prediction accuracy. The model is stacked with seven algorithms different machine learning algorithms such as Radom Forest, Naïve Bayes, Linear Regression, Decision Tree, Ad boost, K Nearest Neighbour, and Gradient Boosting. The experiment was carried out with a training and testing ration of 80:20 in ration. Evaluations are carried out in different measures such as Precision, Recall, F Score, and Accuracy to demonstrate the efficiency of the algorithms. Form the experimentation it is observed that the gradient boosting outperforms the other competitive approaches as this algorithm combines weak predictive models to form a stronger ensemble model that can make highly accurate predictions with an accuracy of 94.67 percentages.
Copyright © 2023 S. Mohapatra et al., licensed to EAI. This is an open access article distributed under the terms of the CC BY-NC-SA 4.0, which permits copying, redistributing, remixing, transformation, and building upon the material in any medium so long as the original work is properly cited.