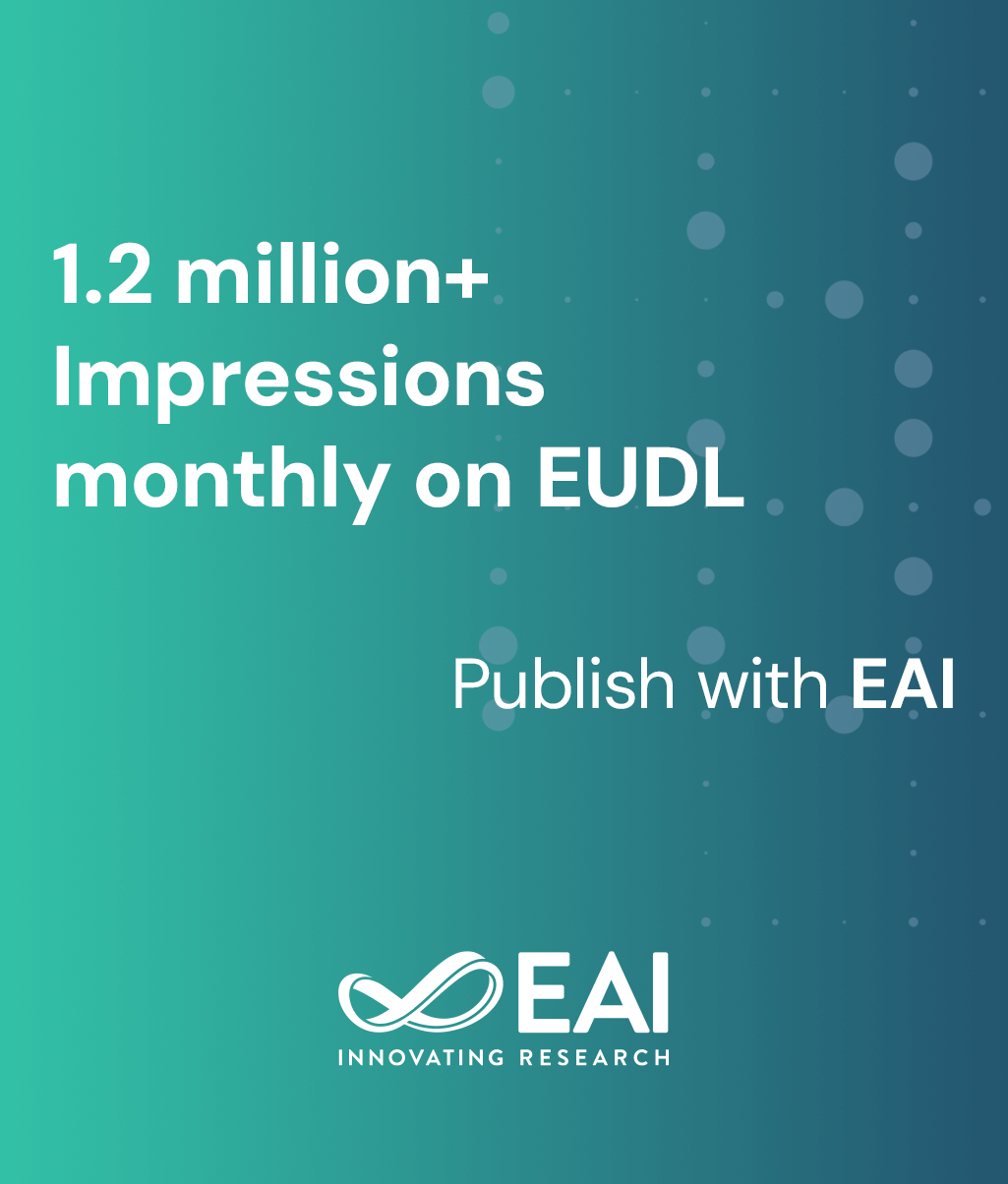
Research Article
Unraveling the Heterogeneity of Lower-Grade Gliomas: Deep Learning-Assisted Flair Segmentation and Genomic Analysis of Brain MR Images
@ARTICLE{10.4108/eetpht.9.4016, author={Hritwik Ghosh and Kareemulla Shaik and Syed Khasim and Gnanajeyaraman Rajaram}, title={Unraveling the Heterogeneity of Lower-Grade Gliomas: Deep Learning-Assisted Flair Segmentation and Genomic Analysis of Brain MR Images}, journal={EAI Endorsed Transactions on Pervasive Health and Technology}, volume={9}, number={1}, publisher={EAI}, journal_a={PHAT}, year={2023}, month={9}, keywords={Lower-grade gliomas, Brain MR images, FLAIR abnormalities, Deep learning models, Segmentation, DeepLabv3, UNet, DenseNet121Unet, ResNet50, EfficientNet, CCE, WCE, WMDL, Border region artifacts, 3D image patches, The Cancer Image Archive, TCIA, Genomic cluster data}, doi={10.4108/eetpht.9.4016} }
- Hritwik Ghosh
Kareemulla Shaik
Syed Khasim
Gnanajeyaraman Rajaram
Year: 2023
Unraveling the Heterogeneity of Lower-Grade Gliomas: Deep Learning-Assisted Flair Segmentation and Genomic Analysis of Brain MR Images
PHAT
EAI
DOI: 10.4108/eetpht.9.4016
Abstract
The precise identification of FLAIR abnormalities in brain MR images is essential for diagnosing and managing lower-grade gliomas, segmentation continues to be a difficult task. In this research, we develop an exhaustive strategy that integrates advanced deep learning models such as DeepLabv3, U-Net, DenseNet121-Unet, ResNet50, Attention U-Net and EfficientNet to effectively segment FLAIR abnormalities in a dataset comprising 110 lower-grade glioma patients. The cancer Imaging achieve (TCIA), includes genomic cluster data and patient-specific details. Our methodology tackles the multi-class data imbalanced by employing a customized loss function, which merges Categorical Cross Entropy (CCE) WCE and WMDL functions are used to calculate loss, allowing the network to accurately segment smaller tumor regions. By performing dense network training on 3D picture patches, the suggested technique improves detection of border region artifacts and efficiently manages storage and system limited resources. We evaluate our strategy’s effectiveness on the presented dataset, emphasizing its potential for assisting correct diagnosis and individualized treatment strategies for patients with lower-grade gliomas.
Copyright © 2023 I. S. Rahat et al., licensed to EAI. This is an open access article distributed under the terms of the CC BY-NC-SA 4.0, which permits copying, redistributing, remixing, transformation, and building upon the material in any medium so long as the original work is properly cited.