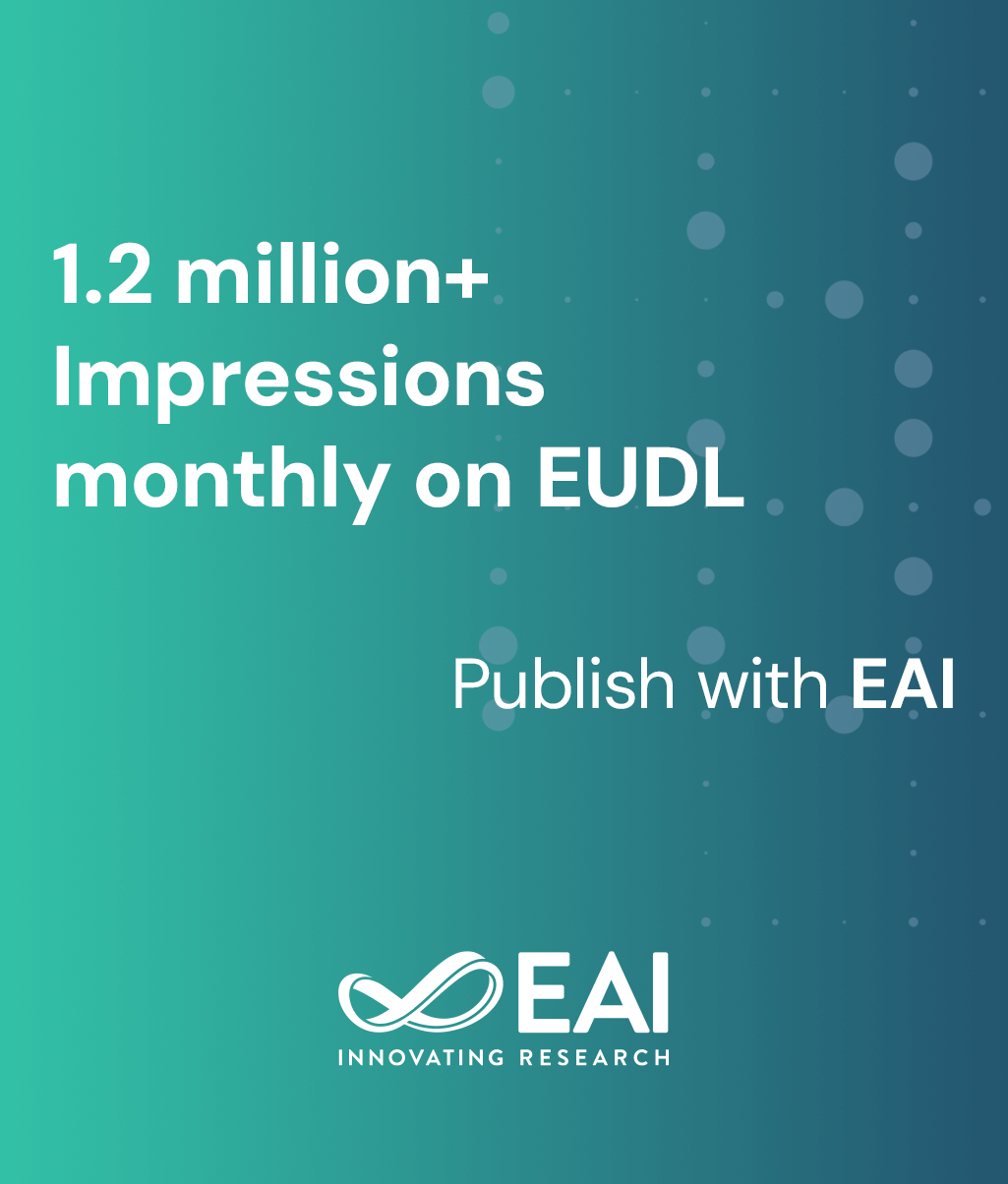
Research Article
Optimized Deep Learning Model for Disease Prediction in Potato Leaves
@ARTICLE{10.4108/eetpht.9.4001, author={Virendra Kumar Shrivastava and Chetan J Shelke and Aastik Shrivastava and Sachi Nandan Mohanty and Nonita Sharma}, title={Optimized Deep Learning Model for Disease Prediction in Potato Leaves}, journal={EAI Endorsed Transactions on Pervasive Health and Technology}, volume={9}, number={1}, publisher={EAI}, journal_a={PHAT}, year={2023}, month={9}, keywords={Deep Learning, Artificial Intelligence, Machine Learning, Deep Convolutional Neural Network, Optimized Deep Convolutional Neural Network Model, Disease Prediction}, doi={10.4108/eetpht.9.4001} }
- Virendra Kumar Shrivastava
Chetan J Shelke
Aastik Shrivastava
Sachi Nandan Mohanty
Nonita Sharma
Year: 2023
Optimized Deep Learning Model for Disease Prediction in Potato Leaves
PHAT
EAI
DOI: 10.4108/eetpht.9.4001
Abstract
Food crops are important for nations and human survival. Potatoes are one of the most widely used foods globally. But there are several diseases hampering potato growth and production as well. Traditional methods for diagnosing disease in potato leaves are based on human observations and laboratory tests which is a cumbersome and time-consuming task. The new age technologies such as artificial intelligence and deep learning can play a vital role in disease detection. This research proposed an optimized deep learning model to predict potato leaf diseases. The model is trained on a collection of potato leaf image datasets. The model is based on a deep convolutional neural network architecture which includes data augmentation, transfer learning, and hyper-parameter tweaking used to optimize the proposed model. Results indicate that the optimized deep convolutional neural network model has produced 99.22% prediction accuracy on Potato Disease Leaf Dataset.
Copyright © 2023 V. K. Shrivastava et al., licensed to EAI. This is an open access article distributed under the terms of the CC BYNC-SA 4.0, which permits copying, redistributing, remixing, transformation, and building upon the material in any medium so long as the original work is properly cited.