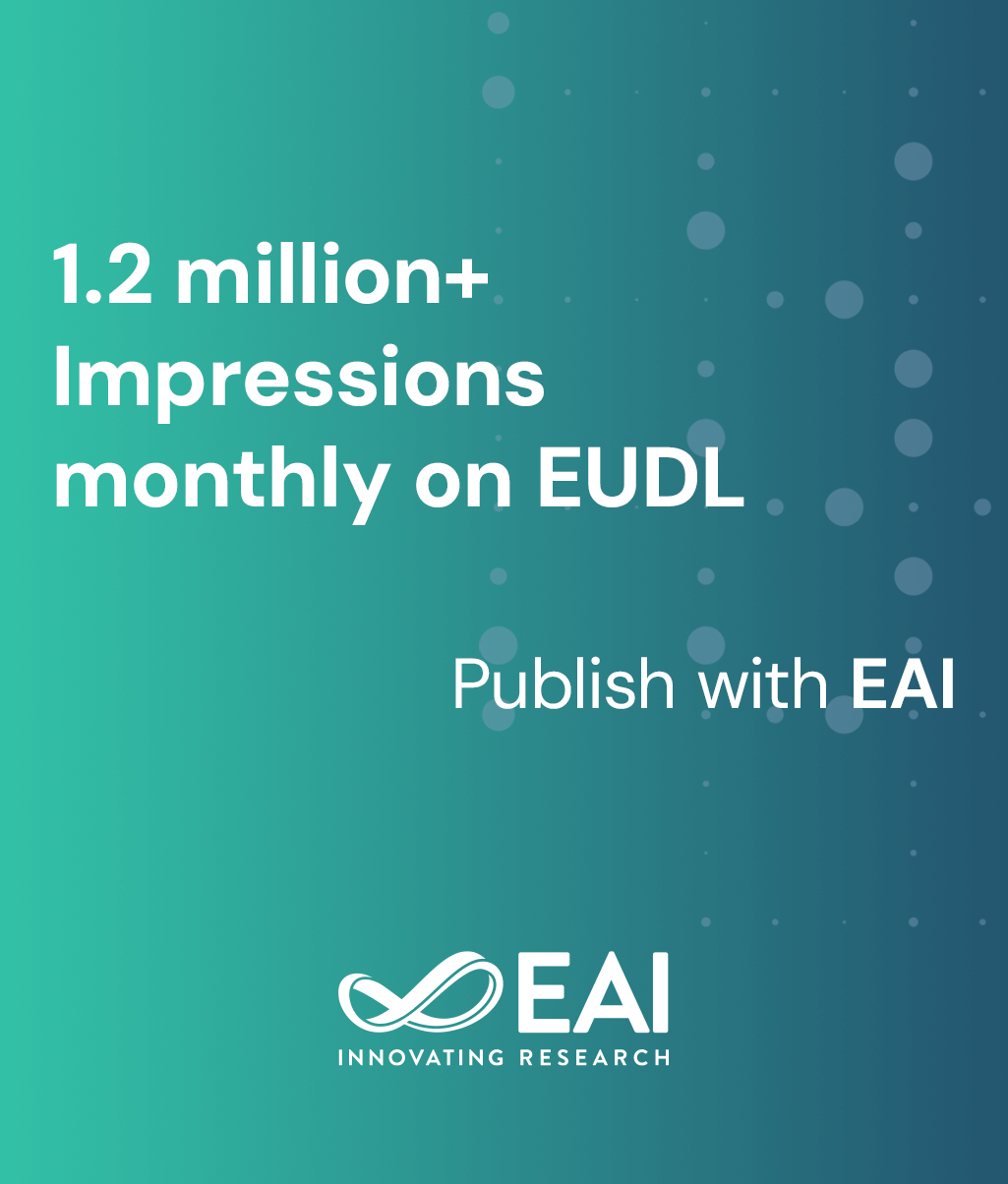
Research Article
Early Alzheimer’s Disease Detection Using Deep Learning
@ARTICLE{10.4108/eetpht.9.3966, author={Kokkula Lokesh and Abbaraju Sai Satwik and Narendra Kumar Rao}, title={Early Alzheimer’s Disease Detection Using Deep Learning}, journal={EAI Endorsed Transactions on Pervasive Health and Technology}, volume={9}, number={1}, publisher={EAI}, journal_a={PHAT}, year={2023}, month={9}, keywords={Classification Detection, Deep Learning, AzNet, DenseNet, ResNet, EfficientNet, InceptionNet}, doi={10.4108/eetpht.9.3966} }
- Kokkula Lokesh
Abbaraju Sai Satwik
Narendra Kumar Rao
Year: 2023
Early Alzheimer’s Disease Detection Using Deep Learning
PHAT
EAI
DOI: 10.4108/eetpht.9.3966
Abstract
The early detection of Alzheimer's disease, a neurodegenerative ailment that affects both cognitive and social functioning, can be accomplished using deep learning technology. Deep learning is more accurate and efficient than human diagnosis in detecting functional connectivity and changes in the brain networks of people with MCI. Early detection of Mild Cognitive Impairment (MCI) can reduce the disease's development. However, achieving high accuracy levels is difficult due to the dearth of reliable biomarkers. The dataset was picked up from the Kaggle database. It contains magnetic resonance images of the brain, each image being unique and in different stages of the disease for classification purpose for our project, as it was most suitable for our project’s needs. We developed a deep learning model using learning AZ net, Dense net, Resnet, Efficient Net and Inception Net with a maximum accuracy of 99.96% for classifying Alzheimer's disease stages and early detection using transfer learning and other approaches.
Copyright © 2023 K. Lokesh et al., licensed to EAI. This is an open access article distributed under the terms of the CC BY-NC-SA 4.0, which permits copying, redistributing, remixing, transformation, and building upon the material in any medium so long as the original work is properly cited.