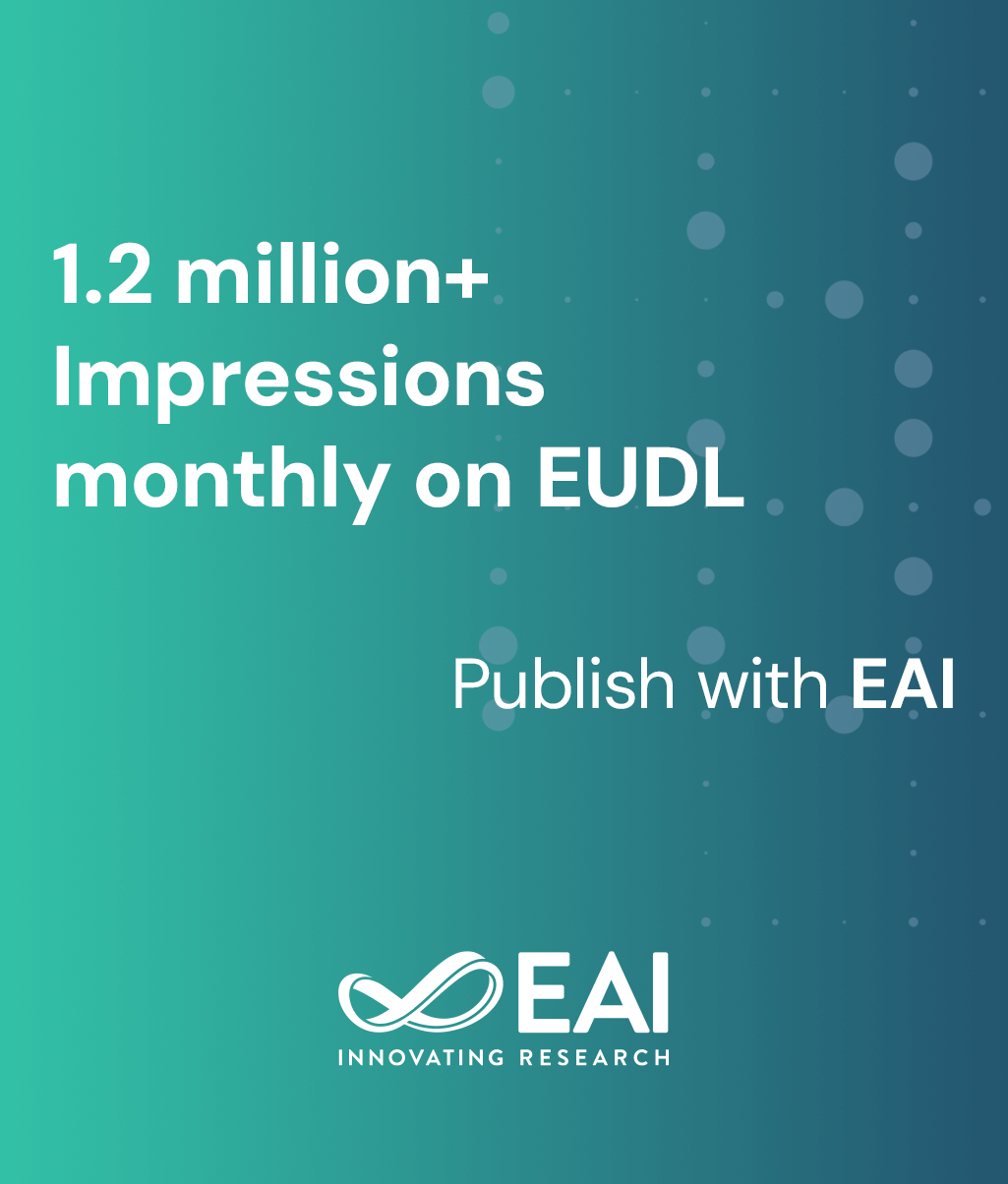
Research Article
An improved ANN-based sequential global-local approximation for small medical data analysis
@ARTICLE{10.4108/eetpht.9.3320, author={Ivan Izonin and Roman Tkachenko and Roman Bliakhar and Michal Kovac and Yevgeniy Bodyanskiy and Olha Chala}, title={An improved ANN-based sequential global-local approximation for small medical data analysis}, journal={EAI Endorsed Transactions on Pervasive Health and Technology}, volume={9}, number={1}, publisher={EAI}, journal_a={PHAT}, year={2023}, month={9}, keywords={small data approach, non-iterative training, global-local approximation, GRNN, machine learning, body fat prediction task}, doi={10.4108/eetpht.9.3320} }
- Ivan Izonin
Roman Tkachenko
Roman Bliakhar
Michal Kovac
Yevgeniy Bodyanskiy
Olha Chala
Year: 2023
An improved ANN-based sequential global-local approximation for small medical data analysis
PHAT
EAI
DOI: 10.4108/eetpht.9.3320
Abstract
INTRODUCTION: The task of approximation of complex nonlinear dependencies, especially in the case of short datasets, is important in various applied fields of medicine. Global approximation methods describe the generalized behavior of the model, while local methods explain the behavior of the model at specific data points. Global-local approximation combines both approaches, which makes such methods a powerful tool for processing short sets of medical data that can have both broad trends and local variations. OBJECTIVES: This paper aims to improve the method of sequential obtaining global and local components of the response surface to increase the accuracy of prediction in the case of short sets of medical data. METHODS: In this paper, the authors developed a new method that combined two ANNs: a non-iterative SGTM neural-like structure for obtaining the global component and GRNN as a powerful tool of local approximation in the case of short datasets. RESULTS: The authors have improved the method of global-local approximation due to the use of a General Regression Neural Network instead of RBF ANN for obtaining the local component, which ensured an increase in the accuracy of the body fat prediction task. The authors optimized the operation of the method and investigated the efficiency of the sequential obtaining global and local components of the response surface in comparison with the efficiency using a number of existing methods. CONCLUSION: The conducted experimental studies for solving the body fat prediction task showed the high efficiency of using the improved method in comparison with a number of existing methods, including ensemble methods.
Copyright © 2023 I. Ivonin et al., licensed to EAI. This is an open access article distributed under the terms of the CC BY-NC-SA 4.0, which permits copying, redistributing, remixing, transformation, and building upon the material in any medium so long as the original work is properly cited.