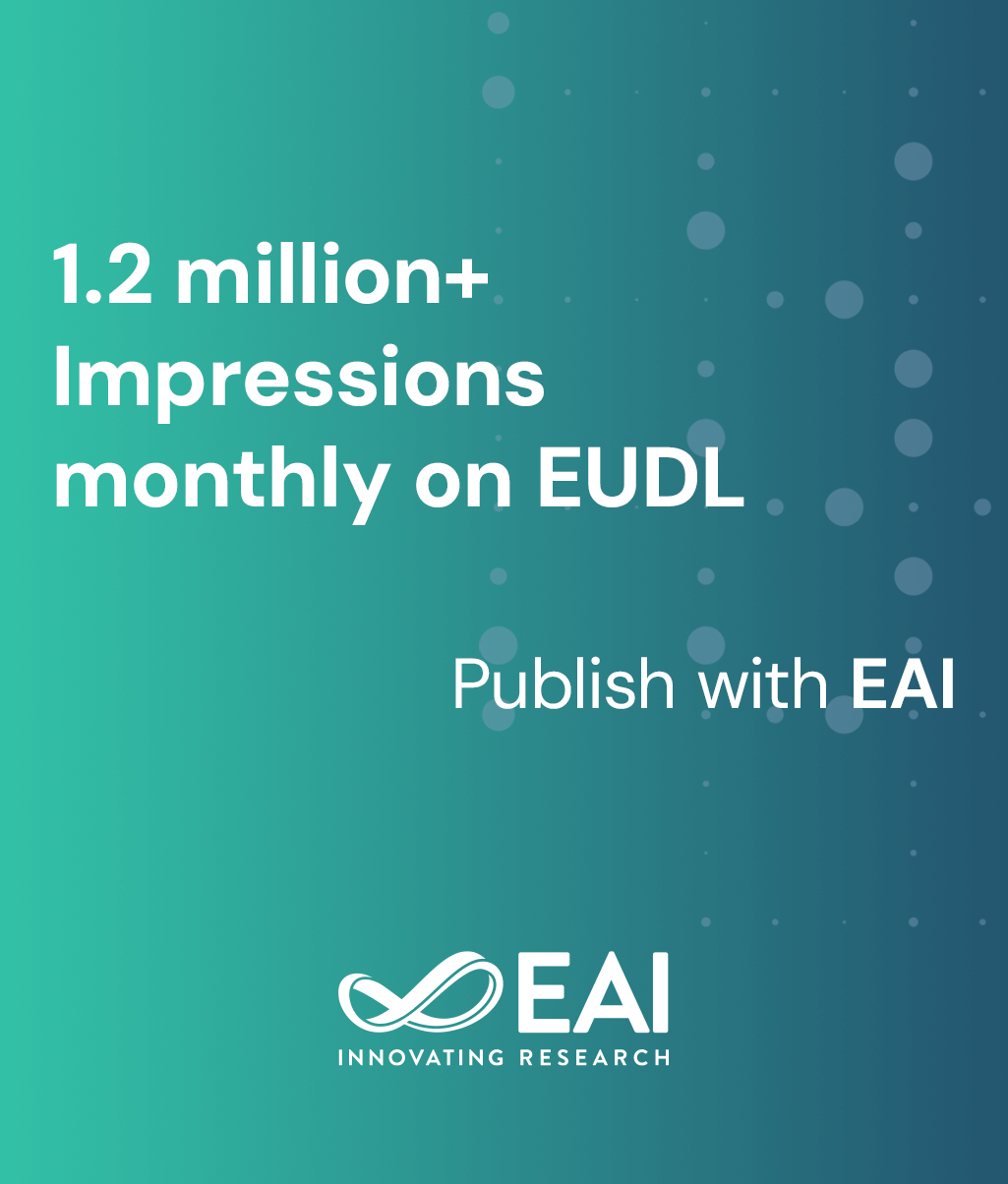
Research Article
Breast cancer early detection in TP53 SNP protein sequences based on a new Convolutional Neural Network model
@ARTICLE{10.4108/eetpht.9.3218, author={Saifeddine Ben Nasr and Imen Messaoudi and Afef Elloumi Oueslati and Zied Lachiri}, title={Breast cancer early detection in TP53 SNP protein sequences based on a new Convolutional Neural Network model}, journal={EAI Endorsed Transactions on Pervasive Health and Technology}, volume={9}, number={1}, publisher={EAI}, journal_a={PHAT}, year={2023}, month={11}, keywords={CNN classification, breast cancer, scalogram, ORB, SNP, tumor suppressor genes, TP53 gene}, doi={10.4108/eetpht.9.3218} }
- Saifeddine Ben Nasr
Imen Messaoudi
Afef Elloumi Oueslati
Zied Lachiri
Year: 2023
Breast cancer early detection in TP53 SNP protein sequences based on a new Convolutional Neural Network model
PHAT
EAI
DOI: 10.4108/eetpht.9.3218
Abstract
INTRODUCTION: Breast cancer (BC) is the most commonly occurring cancer and the second leading cause for women’s disease death. The BC cases are associated with genital mutations which are inherited from older generations or acquired overtime. If the diagnosis is done at the first stage, effects associated with certain treatments can be limited, costs can be saved and the diagnostic time can be minimized. This can also help specialists target the best treatment to increase the rate of cures. Nevertheless, its discovery in patients is very challenging due to silent symptoms aside from the fact the routine screening is not recommended for women under 40 years old. OBJECTIVES: Several efforts are aimed at the BC early detection using machine and deep learning systems. The proposed algorithms use different data types to distinguish between cancerous and non-cancerous cases; as: mammography, ultrasound and MRI (magnetic resonance imaging) images. Then, different learning tools were applied on this data for the classification task. Despite the classification rates which exceed 90%, the major drawback of all these methods is that they are applicable only after the appearance of the cancerous tumors, which reduces the cure rates. METHODS: We propose a new technique for early breast cancer screening. For the data, we focus on cancerous and non-cancerous SNP (Single Nucleotide Polymorphism) protein sequences of the TP53 gene in chromosome 17. This gene is shown to be linked to different single amino acid mutations on which we will shed light here. The method we propose transforms SNP textual sequences into digital vectors via coding. Then, RGB scalogram images are generated using the continuous wavelet transform. A pretreatment of color coefficients is applied to scalograms aiming at creating four different databases. Finally, a CNN deep learning network is used for the binary classification of cancerous and non-cancerous images. RESULTS: During the validation process, we reached good performance with specificity of 97.84%, sensitivity of 96.45%, an overall accuracy of 95.29% and an equal run time of 12 minutes 3 seconds. These values ensure the efficiency of our method.To enhance more these results, we used the ORB feature detection technique. Consequently, the classification rates have been improved to reach 95.9% as accuracy CONCLUSION: Our method will allow significant savings time and lives by detecting the disease in patients whose genetic mutations are beginning to appear.
Copyright © 2023 S. Ben Nasr et al., licensed to EAI. This is an open access article distributed under the terms of the CC BY-NC-SA 4.0, which permits copying, redistributing, remixing, transformation, and building upon the material in any medium so long as the original work is properly cited.