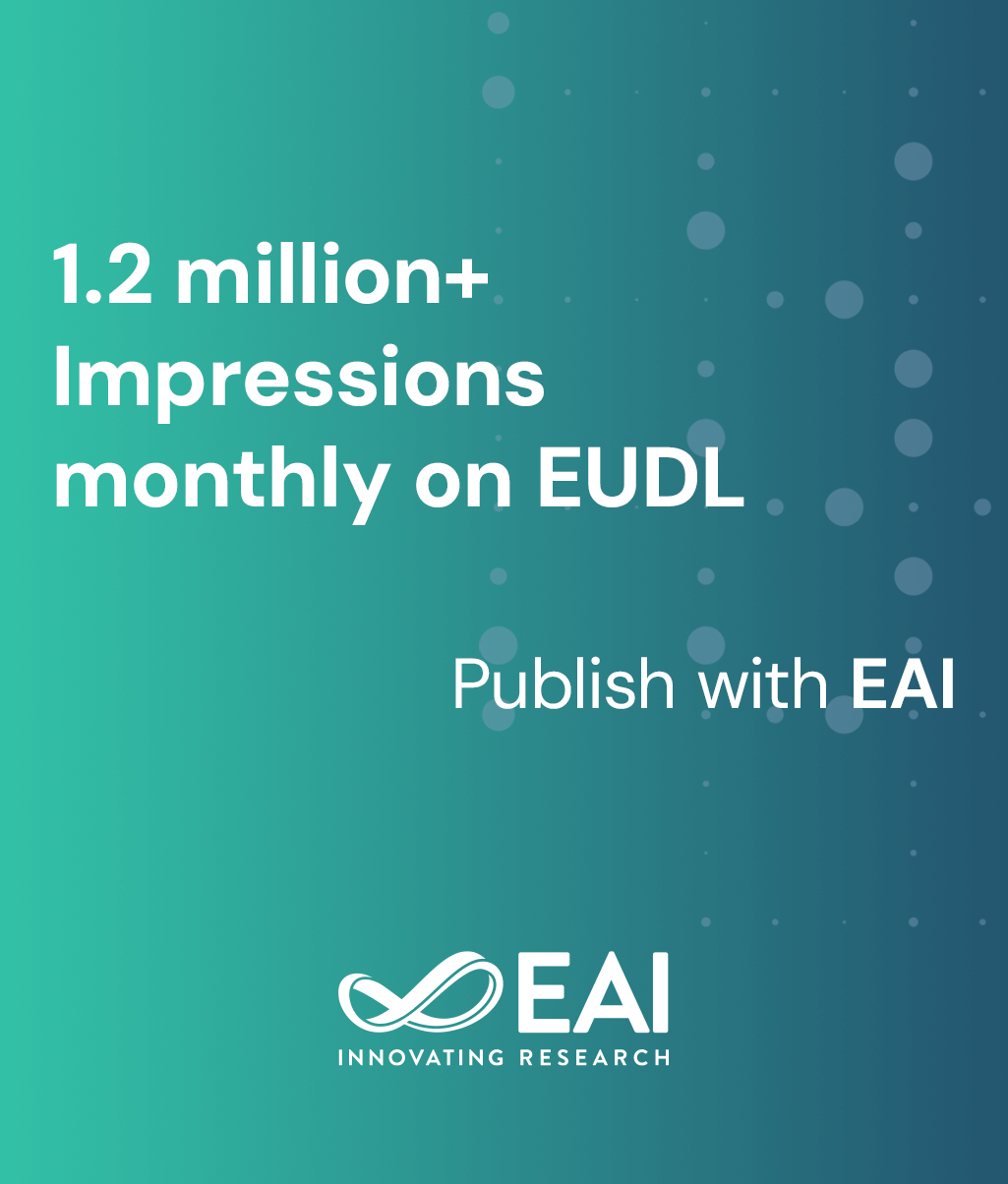
Editorial
SAM2CLIP2SAM: Vision Language Model for Segmentation of 3D CT Scans for Covid-19 Detection
@ARTICLE{10.4108/eetpht.11.9010, author={Dimitrios Kollias and Anastasios Arsenos and James Wingate and Stefanos Kollias}, title={SAM2CLIP2SAM: Vision Language Model for Segmentation of 3D CT Scans for Covid-19 Detection}, journal={EAI Endorsed Transactions of Pervasive Health and Technology}, volume={11}, number={1}, publisher={EAI}, journal_a={PHAT}, year={2025}, month={4}, keywords={RACNet, SAM, CLIP, segmentation, classification, Covid-19 detection, COV-19 CT-DB}, doi={10.4108/eetpht.11.9010} }
- Dimitrios Kollias
Anastasios Arsenos
James Wingate
Stefanos Kollias
Year: 2025
SAM2CLIP2SAM: Vision Language Model for Segmentation of 3D CT Scans for Covid-19 Detection
PHAT
EAI
DOI: 10.4108/eetpht.11.9010
Abstract
This paper presents a new approach for effective segmentation of images that can be integrated into any model and methodology; the paradigm that we choose is classification of medical images (3-D chest CT scans) for Covid-19 detection. Our approach includes a combination of vision-language models that segment the CT scans, which are then fed to a deep neural architecture, named RACNet, for Covid-19 detection. In particular, a novel framework, named SAM2CLIP2SAM, is introduced for segmentation that leverages the strengths of both Segment Anything Model (SAM) and Contrastive Language-Image Pre-Training (CLIP) to accurately segment the right and left lungs in CT scans, subsequently feeding these segmented outputs into RACNet for classification of COVID-19 and non-COVID-19 cases. At first, SAM produces multiple part-based segmentation masks for each slice in the CT scan; then CLIP selects only the masks that are associated with the regions of interest (ROIs), i.e., the right and left lungs; finally SAM is given these ROIs as prompts and generates the final segmentation mask for the lungs. Experiments are presented across two Covid-19 annotated databases which illustrate the improved performance obtained when our method has been used for segmentation of the CT scans.
Copyright © 2025 D. Kollias et al., licensed to EAI. This is an open access article distributed under the terms of the Creative Commons Attribution license (http://creativecommons.org/licenses/by/4.0/), which permits unlimited use, distribution and reproduction in any medium so long as the original work is properly cited.