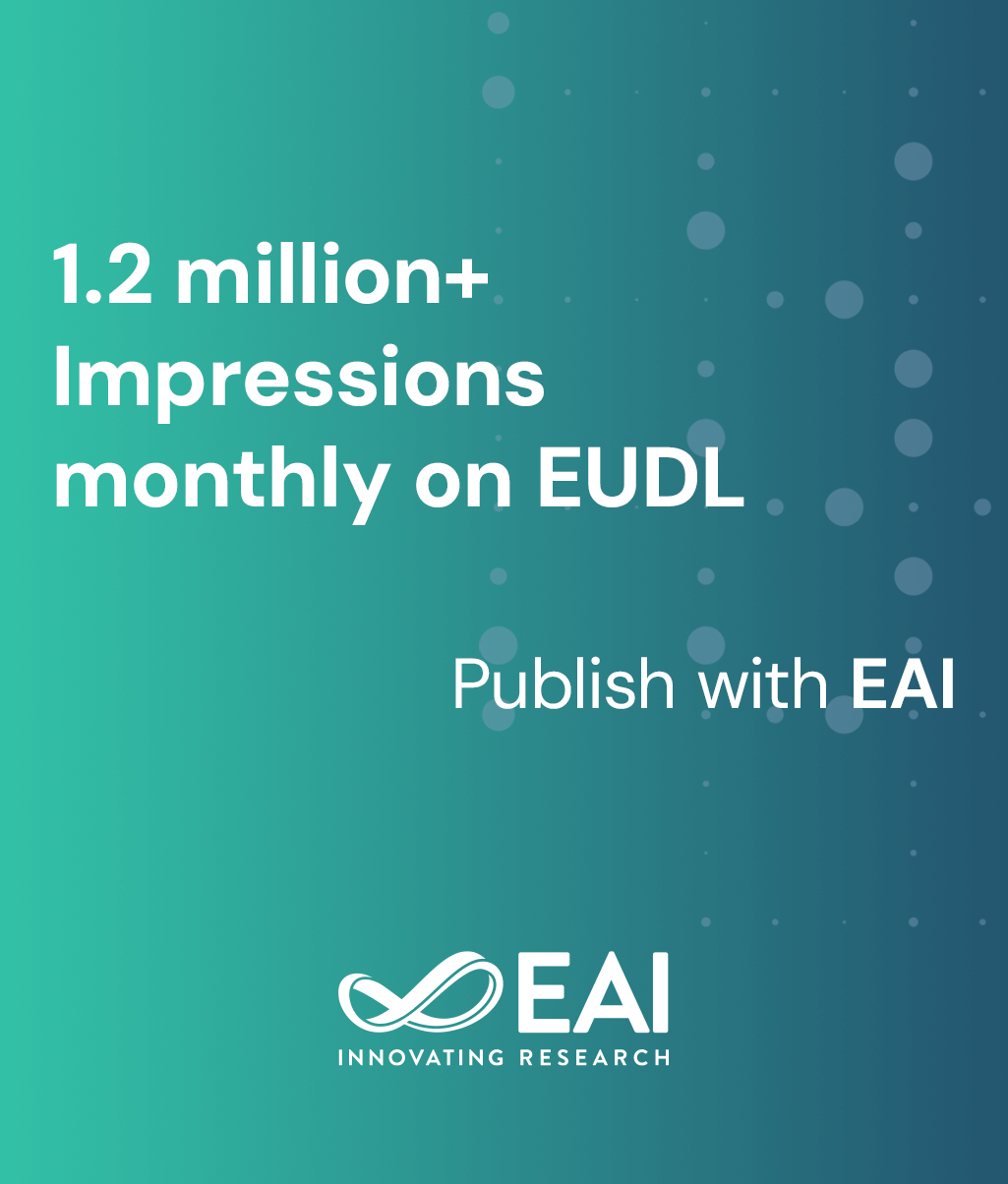
Editorial
Anomaly Detection in Skull scanning Images based on Multi-sensor Fusion
@ARTICLE{10.4108/eetpht.11.5828, author={Xiaochun Guo and Hashim Ali}, title={Anomaly Detection in Skull scanning Images based on Multi-sensor Fusion}, journal={EAI Endorsed Transactions of Pervasive Health and Technology}, volume={11}, number={1}, publisher={EAI}, journal_a={PHAT}, year={2025}, month={3}, keywords={Skull scanning images, Bilateral filter, Double domain decomposition, High and low frequency fusion, Deep neural networks}, doi={10.4108/eetpht.11.5828} }
- Xiaochun Guo
Hashim Ali
Year: 2025
Anomaly Detection in Skull scanning Images based on Multi-sensor Fusion
PHAT
EAI
DOI: 10.4108/eetpht.11.5828
Abstract
INTRODUCTION:Skull bones typically possess complex structures and features. When scanned with ordinary sensors, they are easily affected by noise due to the small difference between abnormal areas and normal tissue. OBJECTIVES: In order to solve the problem of small differences between abnormal areas and normal tissues, which make them susceptible to noise interference, this paper proposes a multi-sensor fusion based skull scan image anomaly detection method. METHODS: Firstly, the frequency correction factor is utilized to modify the frequency domain characteristics of the sensor signal during the skull scanning image acquisition process, aiming to enhance signal quality and reduce noise impact during acquisition. Secondly, bilateral filters and discrete wavelet transform are employed to subject the skull scanning image to dual domain decomposition in spatial and transformation domains, aiding in distinguishing between normal and abnormal regions. Subsequently, the low-frequency fusion algorithm guided by filtering and the high-frequency fusion algorithm based on multi-scale morphological gradients are fused, and the fused dual frequency components are merged back into the original spatial domain to retain important details. The fused reconstructed image aids in improving the accuracy of anomaly detection. Finally, a backbone network with an auto encoder structure is established to learn the feature representation of fused images, and an unsupervised deep neural network is employed to establish a detection model for anomaly detection in skull scanning images. RESULTS:Through experiments, it has been demonstrated that the F1 score approaches 1, the ROC curve closely approaches the upper left corner, and the AUC value approaches 1 after applying the proposed method for anomaly detection in skull scanning images. CONCLUSION: This algorithm has high sensitivity and low specificity, achieving high detection accuracy and demonstrating good performance.
Copyright © 2025 X. Guo et al., licensed to EAI. This is an open access article distributed under the terms of the CC BY-NC-SA 4.0, which permits copying, redistributing, remixing, transformation, and building upon the material in any medium so long as the original work is properly cited.