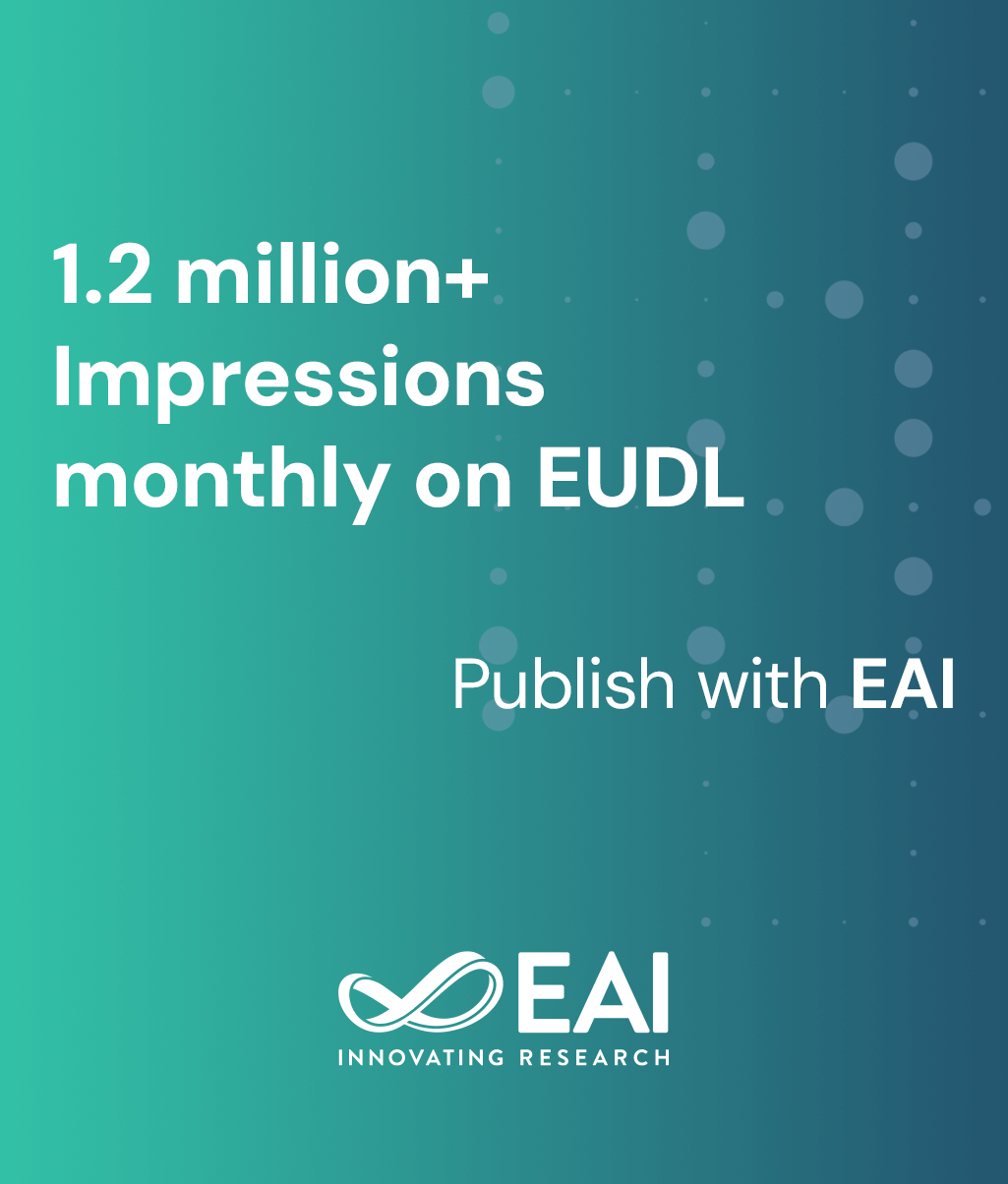
Editorial
Human Muscle sEMG Signal and Gesture Recognition Technology Based on Multi-Stream Feature Fusion Network
@ARTICLE{10.4108/eetpht.10.7230, author={Xiaoyun Wang}, title={Human Muscle sEMG Signal and Gesture Recognition Technology Based on Multi-Stream Feature Fusion Network}, journal={EAI Endorsed Transactions on Pervasive Health and Technology}, volume={10}, number={1}, publisher={EAI}, journal_a={PHAT}, year={2024}, month={9}, keywords={Multi-stream Characteristics, Convolutional Neural Networks, Surface Electromyography Signal, Gestures, Recognition}, doi={10.4108/eetpht.10.7230} }
- Xiaoyun Wang
Year: 2024
Human Muscle sEMG Signal and Gesture Recognition Technology Based on Multi-Stream Feature Fusion Network
PHAT
EAI
DOI: 10.4108/eetpht.10.7230
Abstract
Surface electromyography signals have significant value in gesture recognition due to their ability to reflect muscle activity in real time. However, existing gesture recognition technologies have not fully utilized surface electromyography signals, resulting in unsatisfactory recognition results. To this end, firstly, a Butterworth filter was adopted to remove high-frequency noise from the signal. A combined method of moving translation threshold was introduced to extract effective signals. Then, a gesture recognition model based on multi-stream feature fusion network was constructed. Feature extraction and fusion were carried out through multiple parallel feature extraction paths, combined with convolutional neural networks and residual attention mechanisms. Compared to popular methods of the same type, this new recognition method had the highest recognition accuracy of 92.1% and the lowest recognition error of 5%. Its recognition time for a single-gesture image was as short as 4s, with a maximum Kappa coefficient of 0.92. Therefore, this method combining multi-stream feature fusion networks can effectively improve the recognition accuracy and robustness of gestures and has high practical value.
Copyright © 2024 Xiaoyun Wang, licensed to EAI. This is an open access article distributed under the terms of the CC BY-NC-SA 4.0, which permits copying, redistributing, remixing, transformation, and building upon the material in any medium so long as the original work is properly cited.