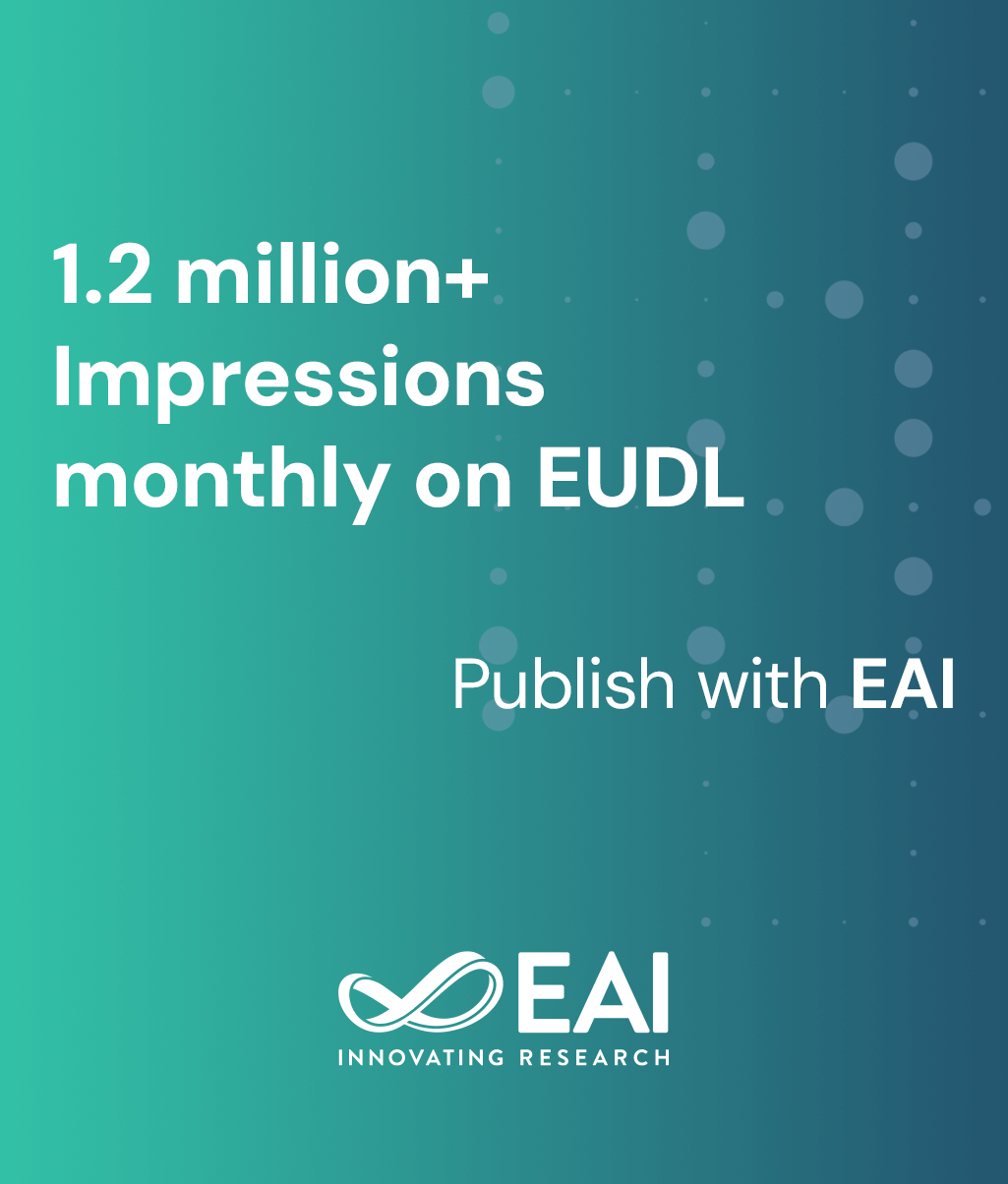
Research Article
NLP and Machine Learning for Sentiment Analysis in COVID-19 Tweets: A Comparative Study
@ARTICLE{10.4108/eetpht.10.7051, author={Shahedhadeennisa Shaik and Chaitra S P}, title={NLP and Machine Learning for Sentiment Analysis in COVID-19 Tweets: A Comparative Study}, journal={EAI Endorsed Transactions on Pervasive Health and Technology}, volume={10}, number={1}, publisher={EAI}, journal_a={PHAT}, year={2024}, month={8}, keywords={Sentiment analysis, Machine learning Algorithms, Performance evaluation, NLP (Natural Language Processing), Sentiment classification, Bidi- rectional Long Short-Term Memory (BLSTM), Decision Tree Classifier, Logistic Regression, K Nearest Neighbors (KNN)}, doi={10.4108/eetpht.10.7051} }
- Shahedhadeennisa Shaik
Chaitra S P
Year: 2024
NLP and Machine Learning for Sentiment Analysis in COVID-19 Tweets: A Comparative Study
PHAT
EAI
DOI: 10.4108/eetpht.10.7051
Abstract
In response to the COVID-19 pandemic, a novel technique is given for assessing the sentiment of individuals using Twitter data obtained from the UCI repository. Our approach involves the identification of tweets with a discernible sentiment, followed by the application of specific data preprocessing techniques to enhance data quality. We have developed a robust model capable of effectively discerning the sentiments behind these tweets. To evaluate the performance of our model, we employ four distinct machine learning algorithms: logistic regres sion, decision tree, k-nearest neighbor and BLSTM. We classify the tweets into three categories: positive, neutral, and negative sentiments. Our performance evaluation is based on several key metrics, including accuracy, precision, recall, and F1-score. Our experimental results indicate that our proposed model excels in accurately capturing the perceptions of individuals regarding the COVID-19 pandemic.
Copyright © 2024 Shahedhadeennisa Shaik et al., licensed to EAI. This is an open access article distributed under the terms of the CC BY-NC-SA 4.0, which permits copying, redistributing, remixing, transformation, and building upon the material in any medium so long as the original work is properly cited.