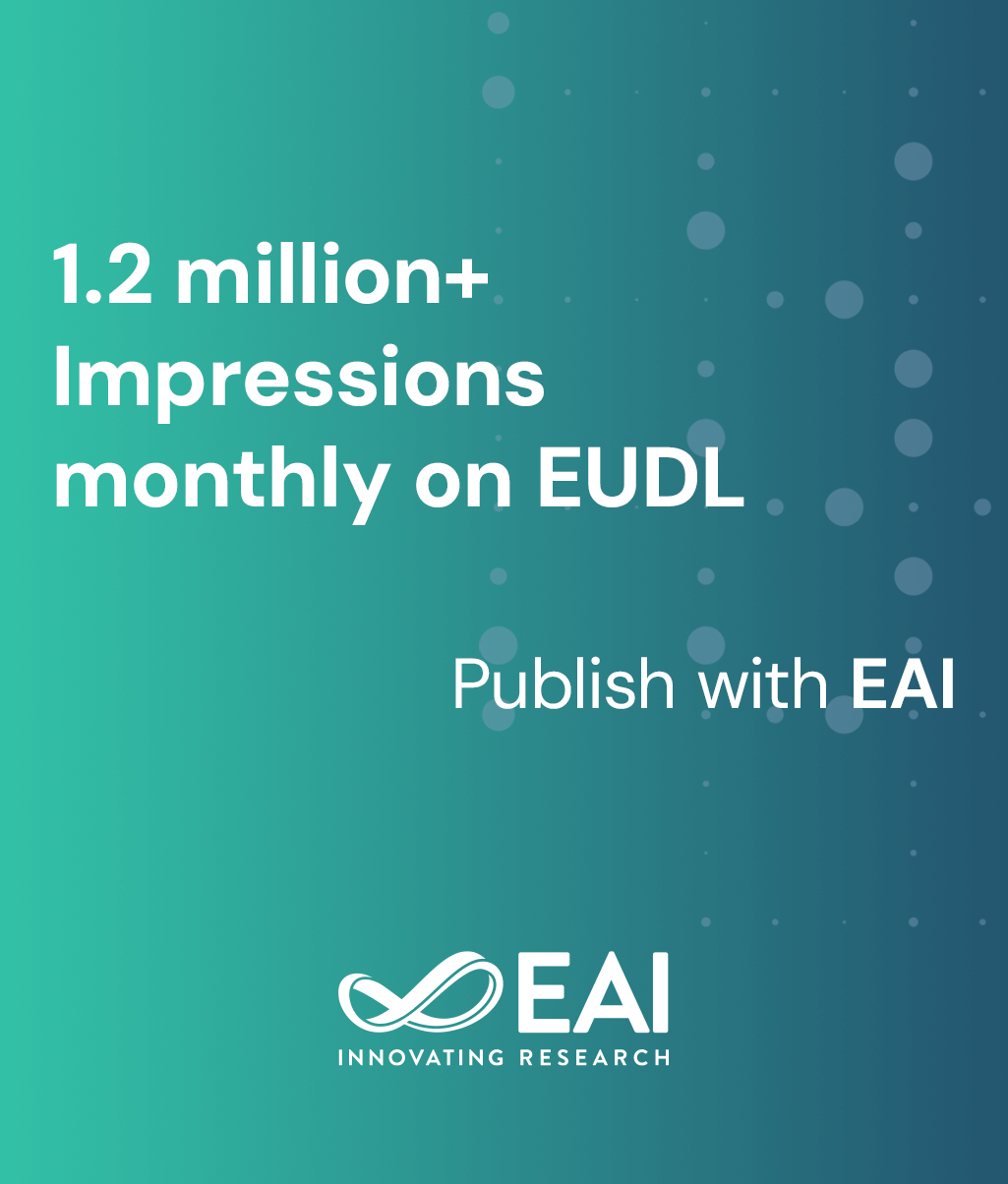
Research Article
Automatic Data-Driven Classification Systems for Cardiovascular Disease
@ARTICLE{10.4108/eetpht.10.6430, author={Muralidharan Jayaraman and Shanmugavadivu Pichai}, title={Automatic Data-Driven Classification Systems for Cardiovascular Disease}, journal={EAI Endorsed Transactions on Pervasive Health and Technology}, volume={10}, number={1}, publisher={EAI}, journal_a={PHAT}, year={2024}, month={12}, keywords={Cardiovascular disease, neural networks, machine learning algorithms, classification}, doi={10.4108/eetpht.10.6430} }
- Muralidharan Jayaraman
Shanmugavadivu Pichai
Year: 2024
Automatic Data-Driven Classification Systems for Cardiovascular Disease
PHAT
EAI
DOI: 10.4108/eetpht.10.6430
Abstract
Cardiovascular disease (CVD) continues to contribute significantly to preventable deaths and avoidable disability worldwide. Prediction and prevention are of utmost importance in the support of public health. Machine learning and deep learning algorithms have emerged as powerful tools to improve the accuracy of diagnosis, prognosis, and treatment of cardiovascular disease. By employing these technologies, medical professionals can gain valuable insights into the risk factors associated with CVD. The focus of this research is to classify and predict cardiovascular diseases using techniques such as support vector machines, ensemble methods, decision trees, random forests, and neural networks. The effectiveness of these algorithms is evaluated based on metrics including accuracy, sensitivity, specificity, area under the curve (AUC), and F1 score. Results show that support vector machines and ensemble methods offer superior accuracy, while neural networks exhibit higher sensitivity and specificity in predicting cardiovascular diseases.
Copyright © 2024 Jayaraman et al., licensed to EAI. This is an open access article distributed under the terms of the CC BY-NC-SA 4.0, which permits copying, redistributing, remixing, transformation, and building upon the material in any medium so long as the original work is properly cited.