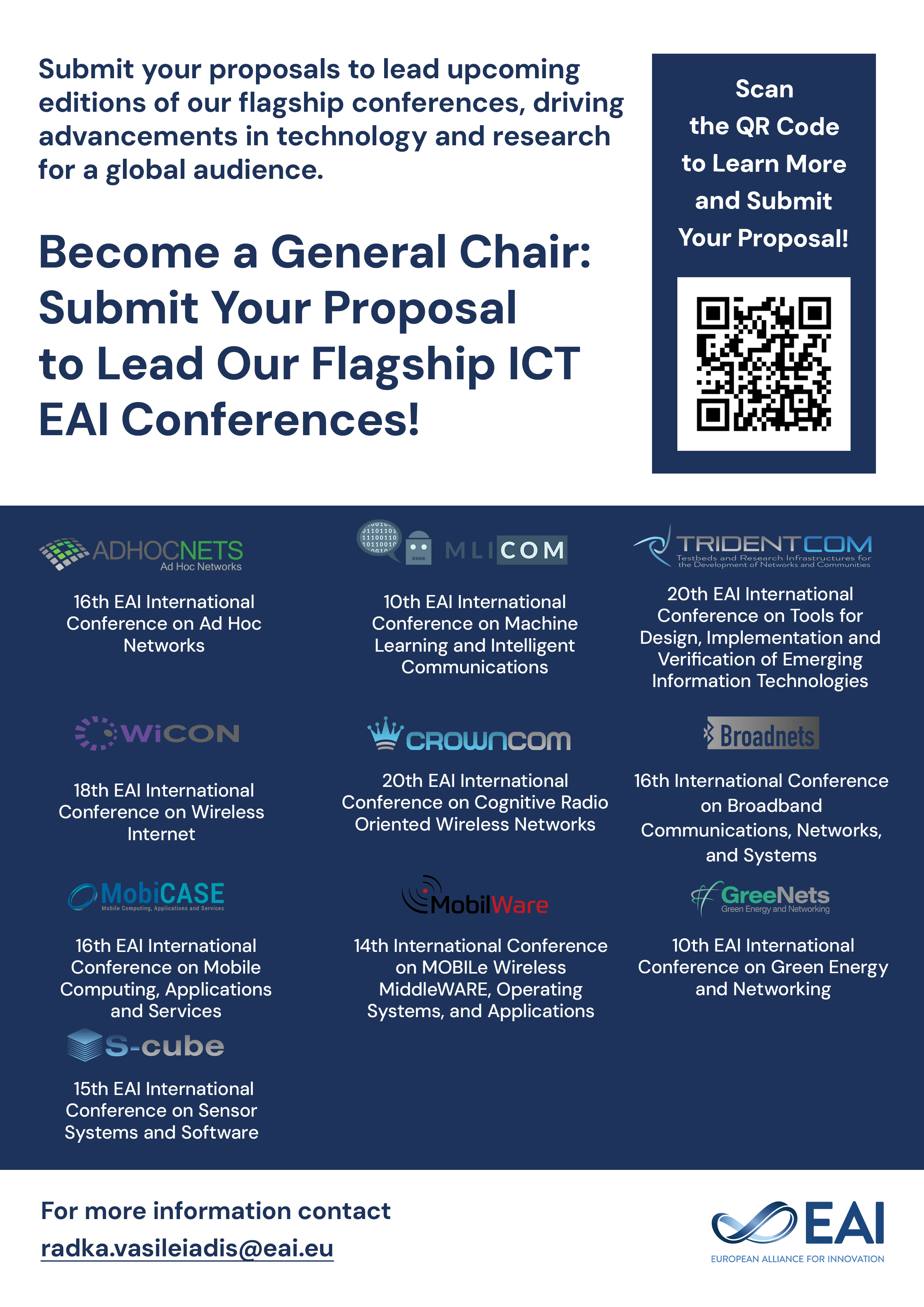
Research Article
Prediction of Paediatric Systemic Lupus Erythematosus Patients Using Machine Learning
@ARTICLE{10.4108/eetpht.10.6386, author={Raja Rajeswari Ponnusamy and Lim Chun Cheak and Elaine Chan Wan Ling and Lim Sern Chin}, title={Prediction of Paediatric Systemic Lupus Erythematosus Patients Using Machine Learning}, journal={EAI Endorsed Transactions on Pervasive Health and Technology}, volume={10}, number={1}, publisher={EAI}, journal_a={PHAT}, year={2024}, month={12}, keywords={Neural Network, Support Vector Machine, PLSE}, doi={10.4108/eetpht.10.6386} }
- Raja Rajeswari Ponnusamy
Lim Chun Cheak
Elaine Chan Wan Ling
Lim Sern Chin
Year: 2024
Prediction of Paediatric Systemic Lupus Erythematosus Patients Using Machine Learning
PHAT
EAI
DOI: 10.4108/eetpht.10.6386
Abstract
Paediatric systemic lupus erythematosus (pSLE) is an autoimmune disease where the body's immune system attacks its own tissues, leading to organ damage. Advances in medical technology and the integration of artificial intelligence have significantly reduced the mortality rate of pSLE patients and improved their quality of life. Various studies have explored the link between environmental pollution and pSLE, utilizing machine learning to identify common gene expressions associated with the disease. However, the application of machine learning, particularly neural networks, to predict the status of pSLE patients over different timeframes remains underexplored. This study aims to demonstrate the effectiveness of support vector machines (SVMs) and neural networks in predicting the status of pSLE patients. Results show that without SMOTE balancing, both SVMs and neural networks achieved an accuracy of 68.09%, while neural networks achieved the highest accuracy of 77.78% after SMOTE balancing. Healthcare stakeholders can employ these machine learning techniques to provide early insights into patients' future health status based on their current condition, thereby improving patient outcomes.
Copyright © 2024 Ponnusamy et al., licensed to EAI. This is an open access article distributed under the terms of the CC BY-NC-SA 4.0, which permits copying, redistributing, remixing, transformation, and building upon the material in any medium so long as the original work is properly cited.