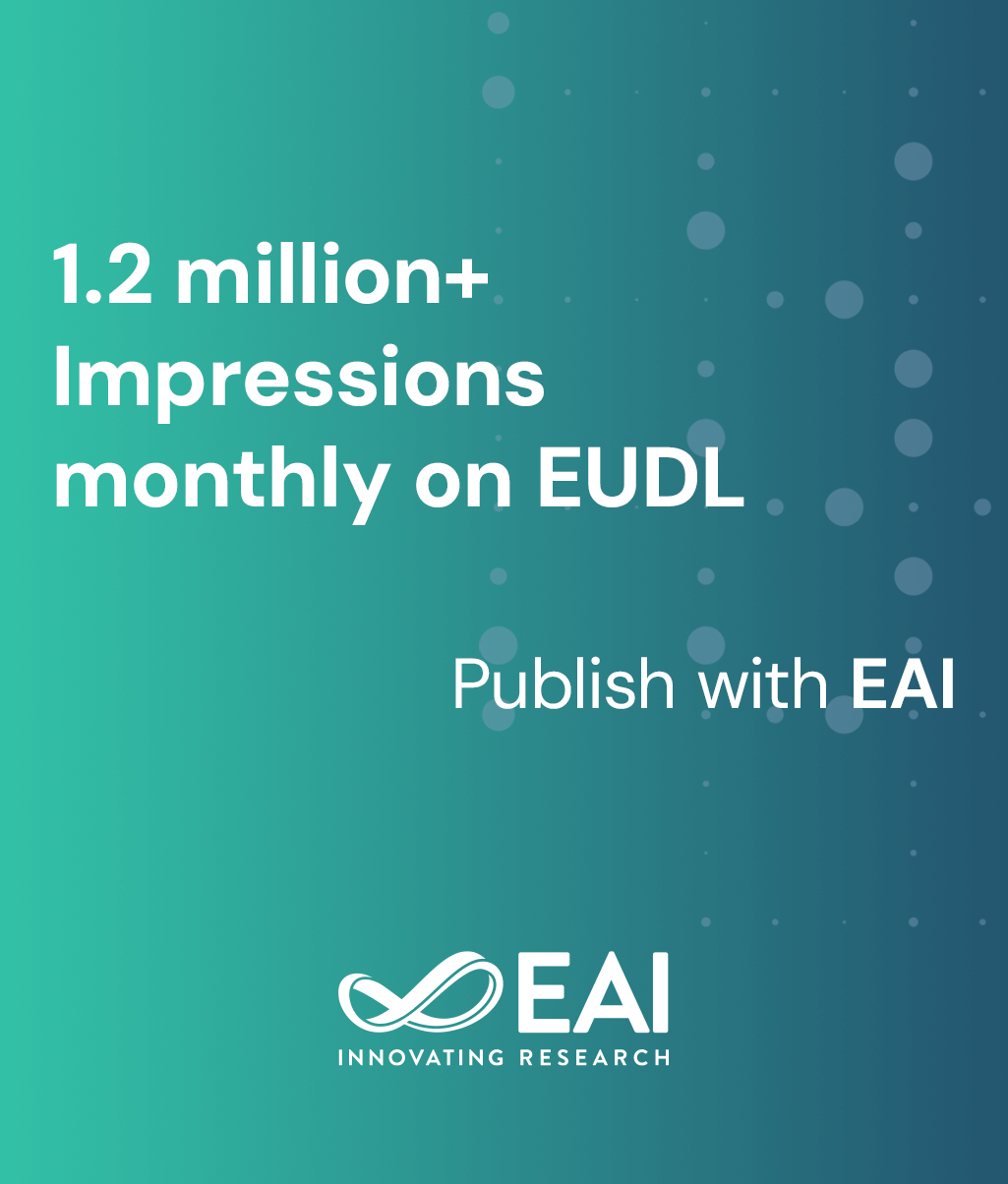
Research Article
Tuberculosis detection bars on VGG19 transfer learning and Zebra Optimization Algorithm
@ARTICLE{10.4108/eetpht.10.5981, author={Tianzhi Le and Fanfeng Shi and Meng Ge and Ran Dong and Dan Shan}, title={Tuberculosis detection bars on VGG19 transfer learning and Zebra Optimization Algorithm}, journal={EAI Endorsed Transactions on Pervasive Health and Technology}, volume={10}, number={1}, publisher={EAI}, journal_a={PHAT}, year={2024}, month={12}, keywords={Tuberculosis Detection, Zebra Optimization Algorithm, Medical Image Analysis}, doi={10.4108/eetpht.10.5981} }
- Tianzhi Le
Fanfeng Shi
Meng Ge
Ran Dong
Dan Shan
Year: 2024
Tuberculosis detection bars on VGG19 transfer learning and Zebra Optimization Algorithm
PHAT
EAI
DOI: 10.4108/eetpht.10.5981
Abstract
Tuberculosis (TB) remains a significant global health challenge, necessitating accurate and efficient diagnostic tools. This study introduces a novel approach combining VGG19, a deep convolutional neural network model, with a newly developed Zebra Optimization Algorithm (ZOA) to enhance the accuracy of TB detection from chest X-ray images. The Zebra Optimization Algorithm, inspired by the social behavior of zebras, was applied to optimize the hyperparameters of the VGG19 model, aiming to improve the model's generalizability and detection performance. Our method was evaluated using a well-defined metric system that included accuracy, sensitivity, and specificity. Results indicate that the combination of VGG19 and ZOA significantly outperforms traditional methods, achieving a high accuracy rate, which underscores the potential of hybrid approaches in TB image analysis.
Copyright © 2024 Le et al., licensed to EAI. This is an open access article distributed under the terms of the CC BY-NC-SA 4.0, which permits copying, redistributing, remixing, transformation, and building upon the material in any medium so long as the original work is properly cited.