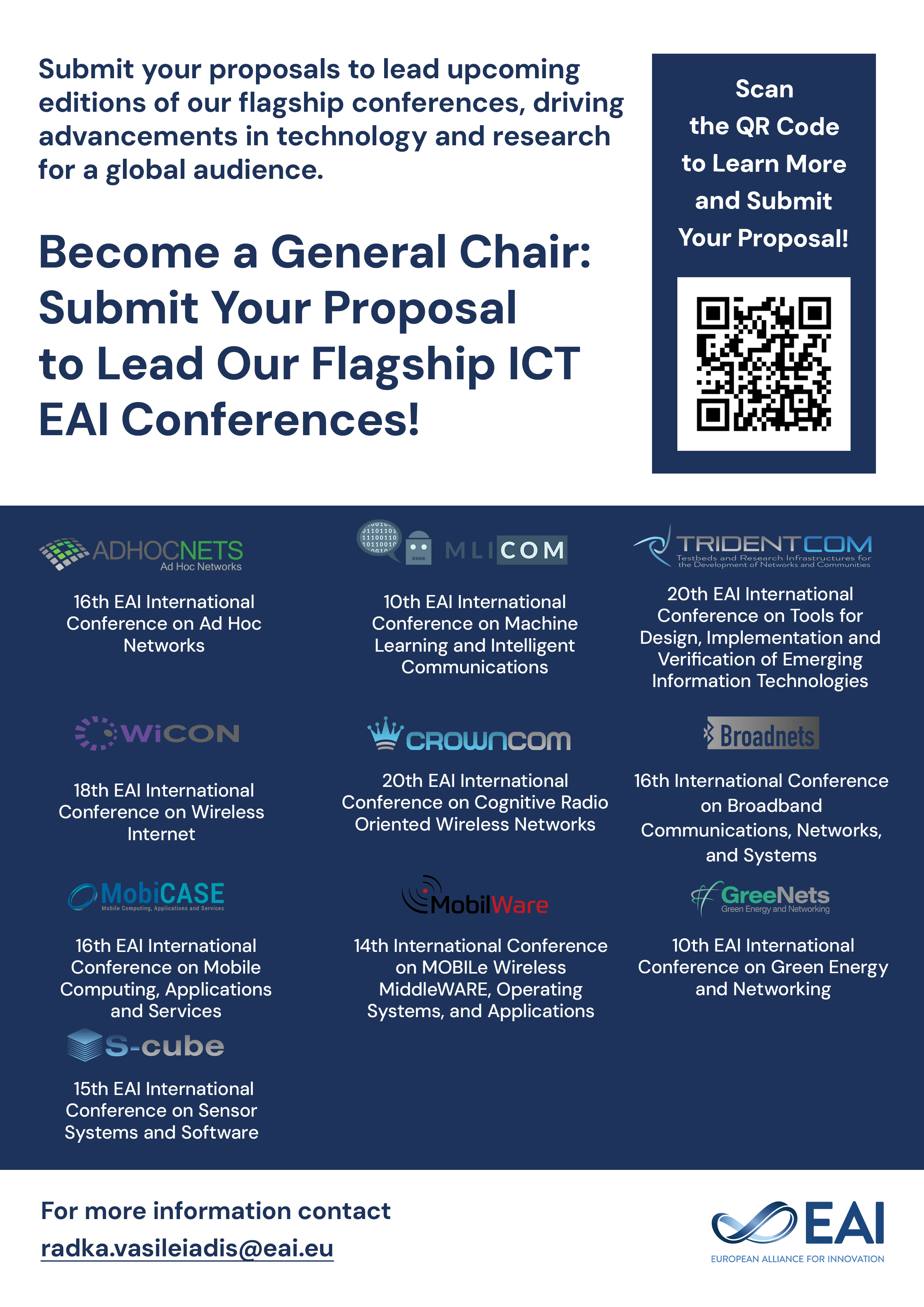
Research Article
A New Deepfake Detection Method Based on Compound Scaling Dual-Stream Attention Network
@ARTICLE{10.4108/eetpht.10.5912, author={Shuya Wang and Chenjun Du and Yunfang Chen }, title={A New Deepfake Detection Method Based on Compound Scaling Dual-Stream Attention Network}, journal={EAI Endorsed Transactions on Pervasive Health and Technology}, volume={10}, number={1}, publisher={EAI}, journal_a={PHAT}, year={2024}, month={12}, keywords={Deepfake detection, compound scaling, channel attention, self-attention, swin transformer}, doi={10.4108/eetpht.10.5912} }
- Shuya Wang
Chenjun Du
Yunfang Chen
Year: 2024
A New Deepfake Detection Method Based on Compound Scaling Dual-Stream Attention Network
PHAT
EAI
DOI: 10.4108/eetpht.10.5912
Abstract
INTRODUCTION: Deepfake technology allows for the overlaying of existing images or videos onto target images or videos. The misuse of this technology has led to increasing complexity in information dissemination on the internet, causing harm to personal and societal public interests. OBJECTIVES: To reduce the impact and harm of deepfake as much as possible, an efficient deepfake detection method is needed. METHODS: This paper proposes a deepfake detection method based on a compound scaling dual-stream attention network, which combines a compound scaling module and a dual-stream attention module based on Swin Transformer to detect deepfake videos. In architectural design, we utilize the compound scaling module to extract shallowlevel features from the images and feed them into the deep-level feature extraction layer based on the dual-stream attention module. Finally, the obtained features are passed through a fully connected layer for classification, resulting in the detection outcome. RESULTS: Experiments on the FF++ dataset demonstrate that the deepfake detection accuracy is 95.62%, which shows its superiority to some extent. CONCLUSION: The method proposed in this paper is feasible and can be used to detect deepfake videos or images.
Copyright © 2024 Wang et al., licensed to EAI. This is an open access article distributed under the terms of the CC BY-NCSA 4.0, which permits copying, redistributing, remixing, transformation, and building upon the material in any medium so long as the original work is properly cited.