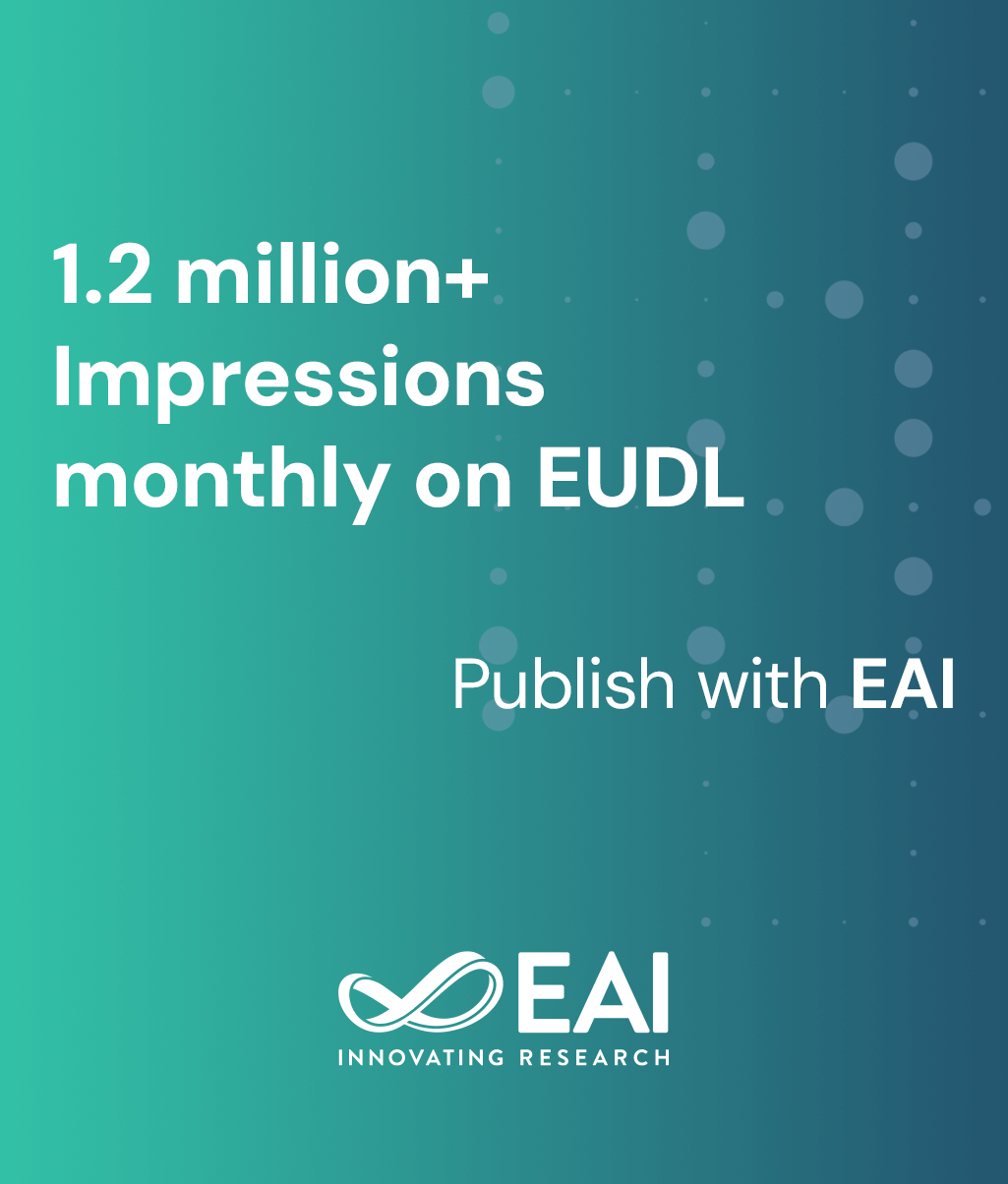
Research Article
Exploring the Potential of Deep Learning in the Classification and Early Detection of Parkinson's Disease
@ARTICLE{10.4108/eetpht.10.5568, author={V S Bakkialakshmi and V Arulalan and Gowdham Chinnaraju and Hritwik Ghosh and Irfan Sadiq Rahat and Ankit Saha}, title={Exploring the Potential of Deep Learning in the Classification and Early Detection of Parkinson's Disease}, journal={EAI Endorsed Transactions on Pervasive Health and Technology}, volume={10}, number={1}, publisher={EAI}, journal_a={PHAT}, year={2024}, month={3}, keywords={Parkinson's Disease, ResNet50, VGG16, Inception v2, AlexNet, VGG19, DL, accuracy, healthcare}, doi={10.4108/eetpht.10.5568} }
- V S Bakkialakshmi
V Arulalan
Gowdham Chinnaraju
Hritwik Ghosh
Irfan Sadiq Rahat
Ankit Saha
Year: 2024
Exploring the Potential of Deep Learning in the Classification and Early Detection of Parkinson's Disease
PHAT
EAI
DOI: 10.4108/eetpht.10.5568
Abstract
INTRODUCTION: Parkinson's Disease (PD) is a progressive neurological disorder affecting a significant portion of the global population, leading to profound impacts on daily life and imposing substantial burdens on healthcare systems. Early identification and precise classification are crucial for effectively managing this disease. This research investigates the potential of deep learning techniques in facilitating early recognition and accurate classification of PD. OBJECTIVES: The primary objective of this study is to leverage advanced deep learning techniques for the early detection and precise classification of Parkinson's Disease. By utilizing a rich dataset comprising speech signal features extracted from 3000 PD patients, including Time Frequency Features, Mel Frequency Cepstral Coefficients (MFCCs), Wavelet Transform based Features, Vocal Fold Features, and TWQT features, this research aims to evaluate the performance of various deep learning models in PD classification. METHODS: The dataset containing diverse speech signal features from PD patients' recordings serves as the foundation for training and evaluating five different deep learning models: ResNet50, VGG16, Inception v2, AlexNet, and VGG19. Each model undergoes training and assessment to determine its capability in accurately classifying PD patients. Performance metrics such as accuracy are employed to evaluate the models' effectiveness. RESULTS: The results demonstrate promising potential, with overall accuracies ranging from 89% to 95% across the different deep learning models. Notably, AlexNet emerges as the top-performing model, achieving an accuracy of 95% and demonstrating balanced performance in accurately identifying both true and false PD cases. CONCLUSION: This research highlights the significant potential of deep learning in facilitating the early detection and classification of Parkinson's Disease. Leveraging speech signal features offers a non-invasive and cost-effective approach to PD assessment. The findings contribute to the growing body of evidence supporting the integration of artificial intelligence in healthcare, particularly in the realm of neurodegenerative disorders. Further exploration into the application of deep learning in this domain holds promise for advancing PD diagnosis and management.
Copyright © 2024 V. S. Bakkialakshmi et al., licensed to EAI. This is an open access article distributed under the terms of the CC BYNC-SA 4.0, which permits copying, redistributing, remixing, transformation, and building upon the material in any medium so long as the original work is properly cited.