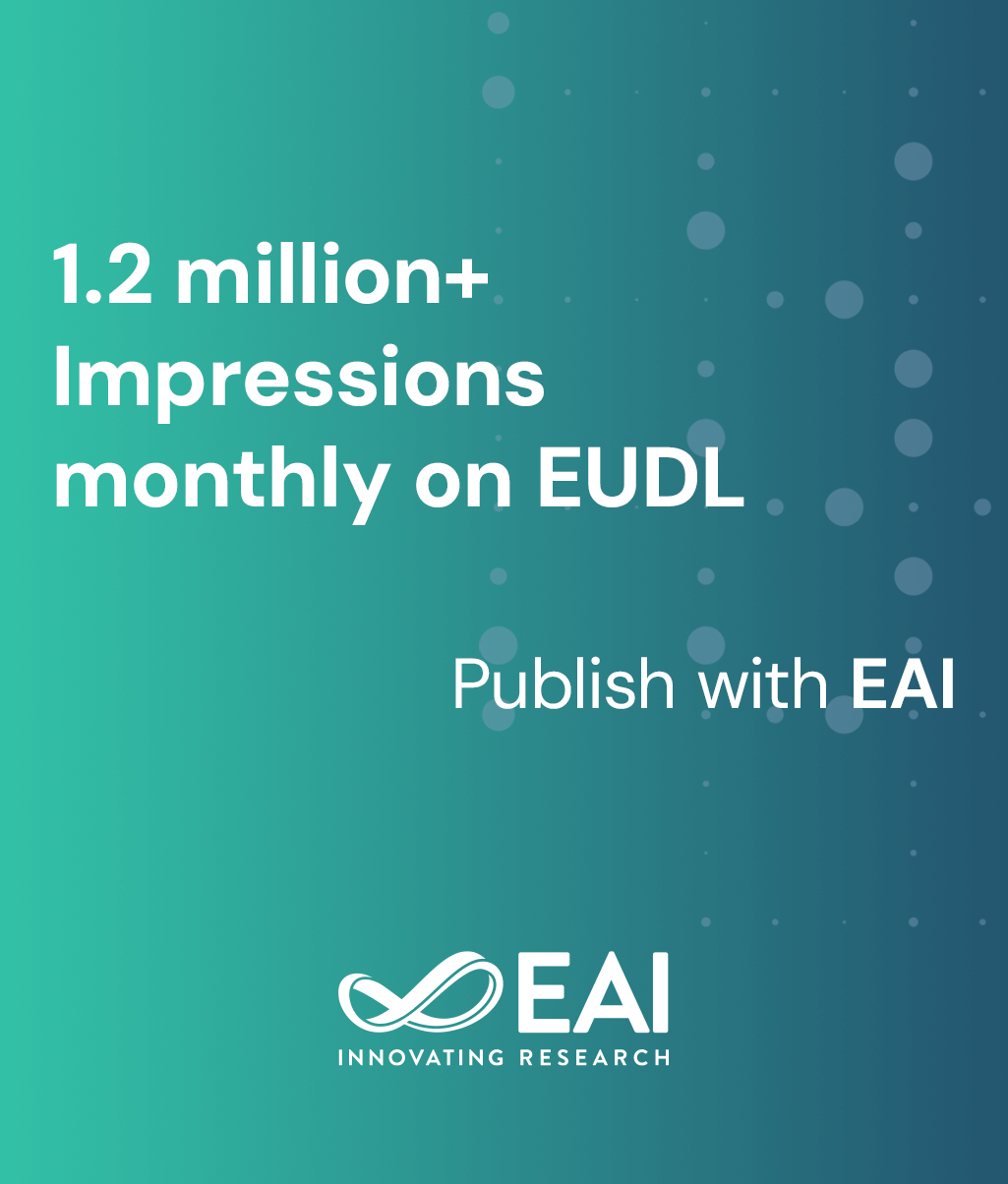
Research Article
Convolutional Neural Networks in Malaria Diagnosis: A Study on Cell Image Classification
@ARTICLE{10.4108/eetpht.10.5551, author={Balajee J and Mohammad Aman Ullah Khan and J Somasekar}, title={Convolutional Neural Networks in Malaria Diagnosis: A Study on Cell Image Classification}, journal={EAI Endorsed Transactions on Pervasive Health and Technology}, volume={10}, number={1}, publisher={EAI}, journal_a={PHAT}, year={2024}, month={3}, keywords={Malaria, ResNet50, AlexNet, Inception V3, VGG19, VGG16, precision, recall, F1-score, deep learning}, doi={10.4108/eetpht.10.5551} }
- Balajee J
Mohammad Aman Ullah Khan
J Somasekar
Year: 2024
Convolutional Neural Networks in Malaria Diagnosis: A Study on Cell Image Classification
PHAT
EAI
DOI: 10.4108/eetpht.10.5551
Abstract
INTRODUCTION: Malaria, a persistent global health threat caused by Plasmodium parasites, necessitates rapid and accurate identification for effective treatment and containment. This study investigates the utilization of convolutional neural networks (CNNs) to enhance the precision and speed of malaria detection through the classification of cell images infected with malaria. OBJECTIVES: The primary objective of this research is to explore the effectiveness of CNNs in accurately classifying malaria-infected cell images. By employing various deep learning models, including ResNet50, AlexNet, Inception V3, VGG19, VGG16, and MobileNetV2, the study aims to assess the performance of each model and identify their strengths and weaknesses in malaria diagnosis. METHODS: A balanced dataset comprising approximately 8,000 enhanced images of blood cells, evenly distributed between infected and uninfected classes, was utilized for model training and evaluation. Performance evaluation metrics such as precision, recall, F1-score, and accuracy were employed to assess the efficacy of each CNN model in malaria classification. RESULTS: The results demonstrate high accuracy across all models, with AlexNet and VGG19 exhibiting the highest levels of accuracy. However, the selection of a model should consider specific application requirements and constraints, as each model presents unique trade-offs between computational efficiency and performance. CONCLUSION: This study contributes to the burgeoning field of deep learning in healthcare, particularly in utilizing medical imaging for disease diagnosis. The findings underscore the considerable potential of CNNs in enhancing malaria diagnosis. Future research directions may involve further model optimization, exploration of larger and more diverse datasets, and the integration of CNNs into practical diagnostic tools for real-world deployment.
Copyright © 2024 H. Ghosh et al., licensed to EAI. This is an open access article distributed under the terms of the CC BY-NC-SA 4.0, which permits copying, redistributing, remixing, transformation, and building upon the material in any medium so long as the original work is properly cited.