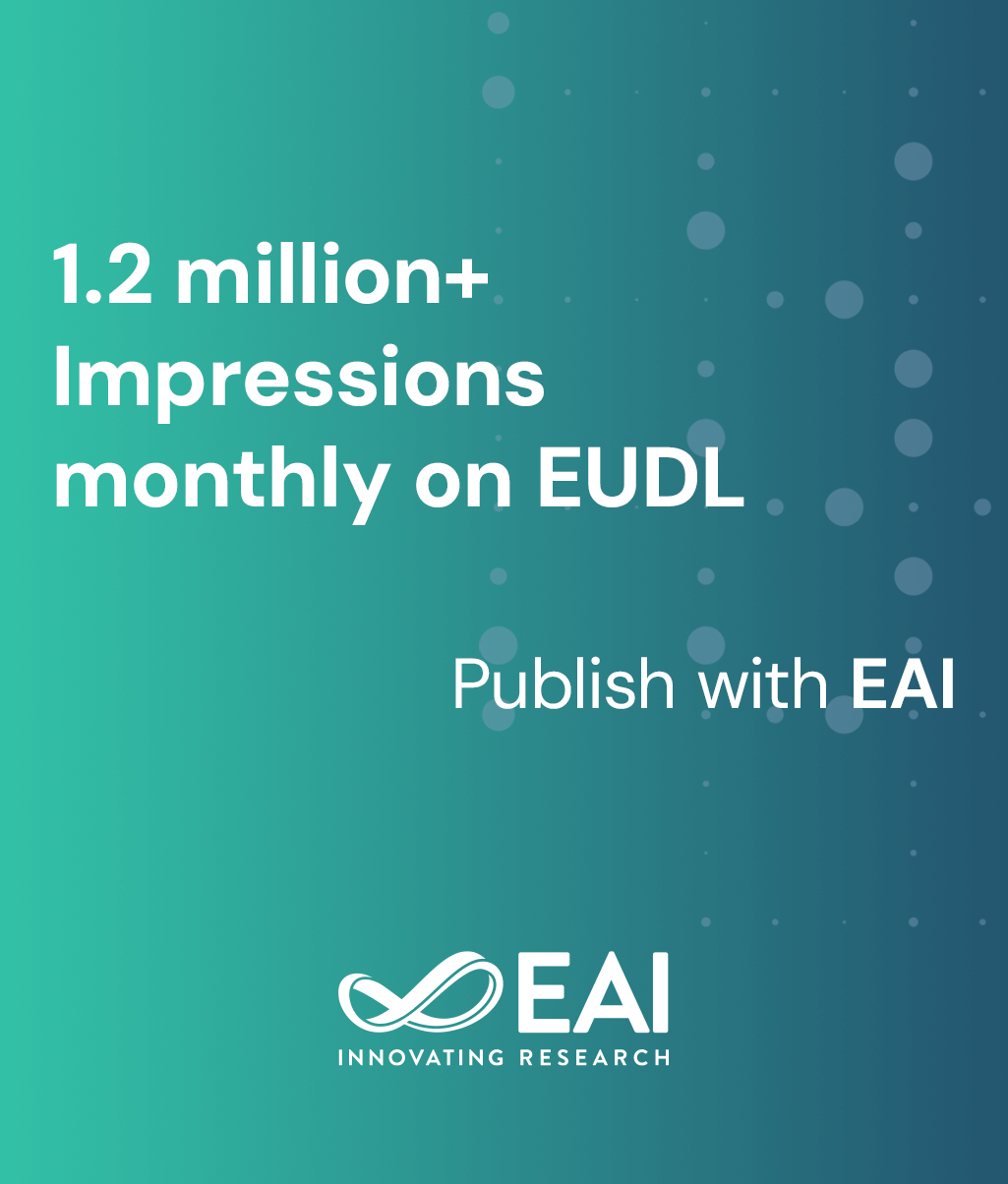
Research Article
Extreme Learning Machine for Biomedical Image Classification: A Multi-Case Study
@ARTICLE{10.4108/eetpht.10.5542, author={Francesco Mercaldo and Luca Brunese and Antonella Santone and Fabio Martinelli and Mario Cesarelli}, title={Extreme Learning Machine for Biomedical Image Classification: A Multi-Case Study}, journal={EAI Endorsed Transactions on Pervasive Health and Technology}, volume={10}, number={1}, publisher={EAI}, journal_a={PHAT}, year={2024}, month={3}, keywords={Extreme Learning Machine, ELM, Biomedical Image, Classification, Machine Learning}, doi={10.4108/eetpht.10.5542} }
- Francesco Mercaldo
Luca Brunese
Antonella Santone
Fabio Martinelli
Mario Cesarelli
Year: 2024
Extreme Learning Machine for Biomedical Image Classification: A Multi-Case Study
PHAT
EAI
DOI: 10.4108/eetpht.10.5542
Abstract
In the current realm of biomedical image classification, the predominant choice remains deep learning networks, particularly convolutional neural network (CNN) models. However, deep learning suffers from a notable drawback in terms of its high training cost, mainly due to intricate data models. A recent alternative, known as the Extreme Learning Machine (ELM), has emerged as a promising solution. Empirical investigations have indicated that ELM can offer satisfactory predictive performance for a wide array of classification tasks, while significantly reducing training costs when compared to deep learning networks trained using back propagation. This research paper introduces a methodology designed to evaluate the suitability of employing the Extreme Learning Machine for biomedical classification tasks. Our study encompasses binary and multiclass classification across four distinct scenarios, involving the analysis of biomedical images obtained from both dermatoscopes and blood cell microscopes. The findings underscore the effectiveness of the Extreme Learning Machine, showcasing its successful utilization in the classification of biomedical images.
Copyright © 2024 F. Mercaldo et al., licensed to EAI. This is an open access article distributed under the terms of the Creative Commons Attribution license (http://creativecommons.org/licenses/by/3.0/), which permits unlimited use, distribution and reproduction in any medium so long as the original work is properly cited.