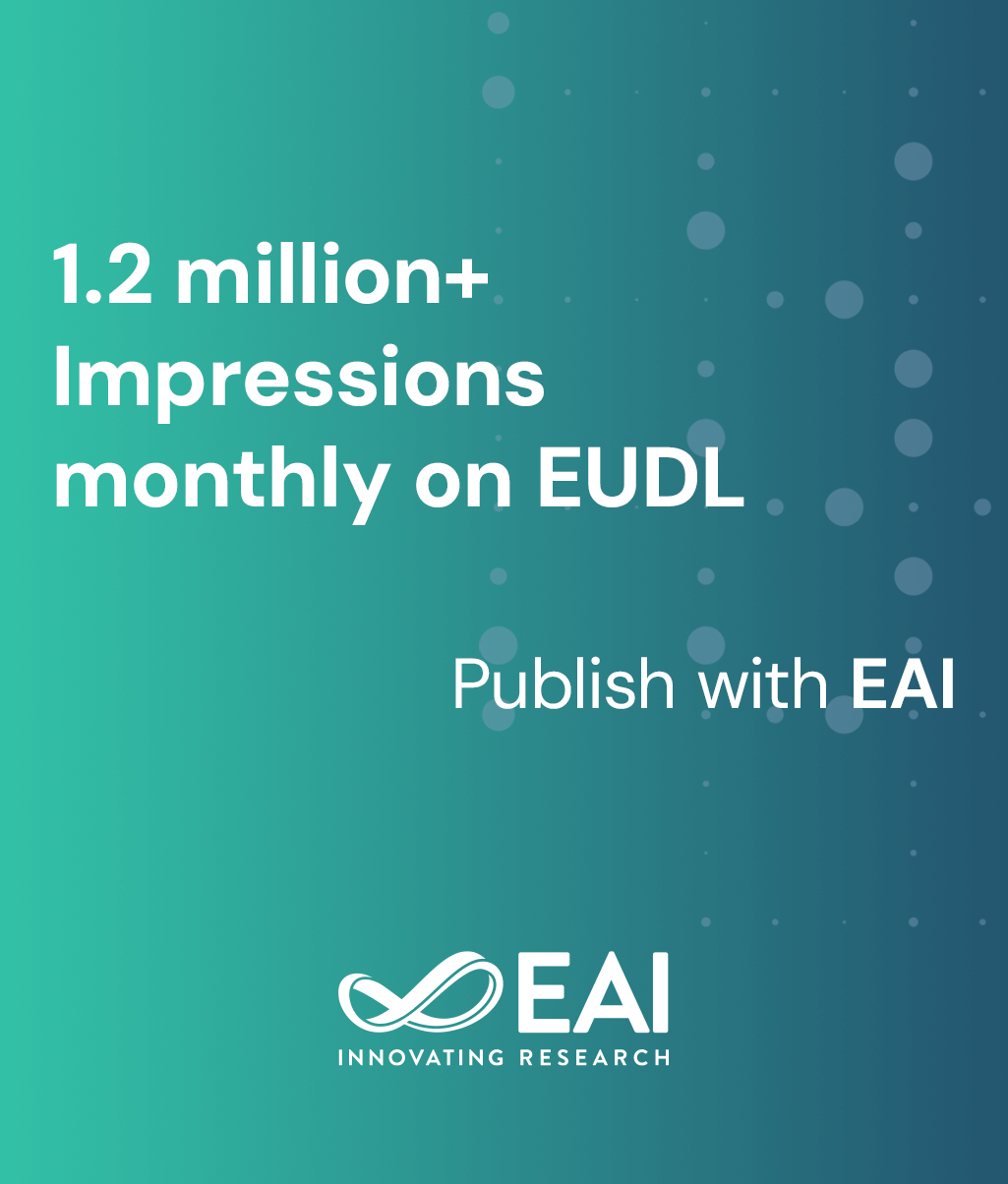
Editorial
Effective Cataract Identification System using Deep Convolution Neural Network
@ARTICLE{10.4108/eetpht.10.5525, author={P N Senthil Prakash and S Sudharson and Venkat Amith Woonna and Sai Venkat Teja Bacham}, title={Effective Cataract Identification System using Deep Convolution Neural Network}, journal={EAI Endorsed Transactions on Pervasive Health and Technology}, volume={10}, number={1}, publisher={EAI}, journal_a={PHAT}, year={2024}, month={3}, keywords={CataractsNET, Cataract Detection, CNN, Deep Learning, Pre-trained networks}, doi={10.4108/eetpht.10.5525} }
- P N Senthil Prakash
S Sudharson
Venkat Amith Woonna
Sai Venkat Teja Bacham
Year: 2024
Effective Cataract Identification System using Deep Convolution Neural Network
PHAT
EAI
DOI: 10.4108/eetpht.10.5525
Abstract
INTRODUCTION: The paper introduces a novel approach for the early detection of cataracts using images captured using smartphones. Cataracts are a significant global eye disease that can lead to vision impairment in individuals aged 40 and above. In this article, we proposed a deep convolution neural network (CataractsNET) trained using an open dataset available in Github which includes images collected through google searches and images generated using standard augmentation mechanism. OBJECTIVES: The main objective of this paper is to design and implement a lightweight network model for cataract identification that outperforms other state-of-the-art network models in terms of accuracy, precision, recall, and F1 Score. METHODS: The proposed neural network model comprises nine layers, guaranteeing the extraction of significant details from the input images and achieving precise classification. The dataset primarily comprises cataract images sourced from a standardized dataset that is publicly available on GitHub, with 8000 training images and 1600 testing images. RESULTS: The proposed CataractsNET model achieved an accuracy of 96.20%, precision of 96.1%, recall of 97.6%, and F1 score of 96.1%. These results demonstrate that the proposed method outperforms other deep learning models like ResNet50 and VGG19. CONCLUSION: The paper concludes that identifying cataracts in the earlier stages is crucial for effective treatment and reducing the likelihood of experiencing blindness. The widespread use of smartphones makes this approach accessible to a broad audience, allowing individuals to check for cataracts and seek timely consultation with ophthalmologists for further diagnosis.
Copyright © 2024 P. N. Senthil Prakash et al., licensed to EAI. This is an open access article distributed under the terms of the CC BY-NC-SA 4.0, which permits copying, redistributing, remixing, transformation, and building upon the material in any medium so long as the original work is properly cited.