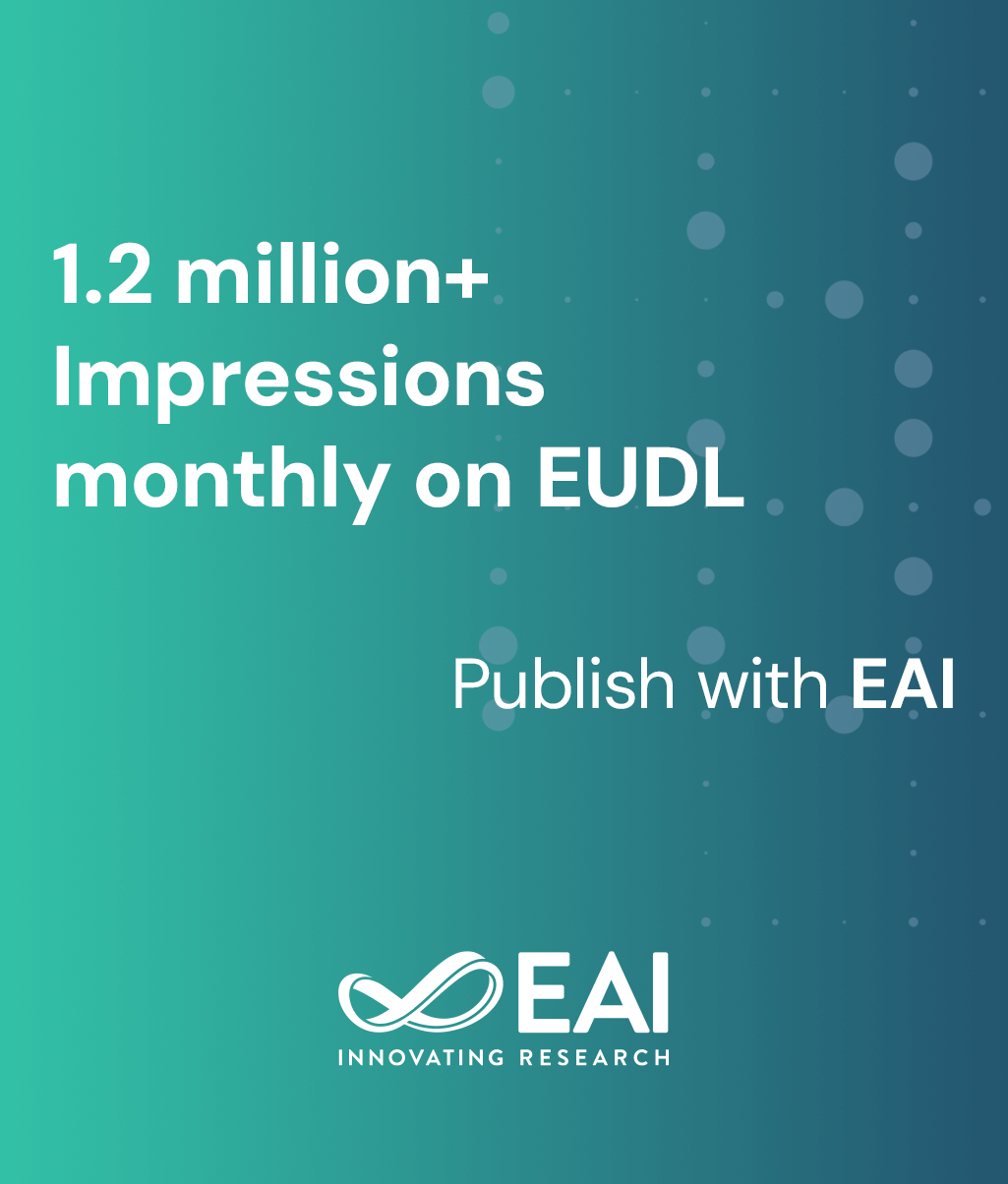
Editorial
Depressonify: BERT a deep learning approach of detection of depression
@ARTICLE{10.4108/eetpht.10.5513, author={Meena Kumari and Gurpreet Singh}, title={Depressonify: BERT a deep learning approach of detection of depression}, journal={EAI Endorsed Transactions on Pervasive Health and Technology}, volume={10}, number={1}, publisher={EAI}, journal_a={PHAT}, year={2024}, month={3}, keywords={Bidirectional Encoder Representations from Transformers, Deep learning, Transformers, Depression Detection}, doi={10.4108/eetpht.10.5513} }
- Meena Kumari
Gurpreet Singh
Year: 2024
Depressonify: BERT a deep learning approach of detection of depression
PHAT
EAI
DOI: 10.4108/eetpht.10.5513
Abstract
INTRODUCTION: Depression is one of the leading psychological problems in the modern tech era where every single person has a social media account that has wide space for the creation of depressed feelings. Since depression can escalate to the point of suicidal thoughts or behavior spotting it early can be vitally important. Traditionally, psychologists rely on patient interviews and questionnaires to gauge the severity of depression. OBJECTIVES: The objective of this paper is earlier depression detection as well as treatment can greatly improve the probability of living a healthy and full life free of depression. METHODS: This paper introduces the utilization of BERT, a novel deep-learning, transformers approach that can detect levels of depression using textual data as input. RESULTS: The main result obtained in this paper is the extensive dataset consists of a total of 20,000 samples, which are categorized into 5 classes and further divided into training, testing, and validation sets, with respective sizes of 16,000, 2,000, and 2,000. This paper has achieved a remarkable result with a training accuracy of 95.5% and validation accuracy of 92.2% with just 5 epochs. CONCLUSION: These are the conclusions of this paper, Deep learning has a lot of potential for use in mental health applications, as seen by the study's outstanding results, which included training accuracy of 95.5%. But the path towards comprehensive and morally sound AI-based mental health support continues into the future.
Copyright © 2024 M. Kumari et al., licensed to EAI. This is an open access article distributed under the terms of the CC BY-NC-SA 4.0, which permits copying, redistributing, remixing, transformation, and building upon the material in any medium so long as the original work is properly cited.