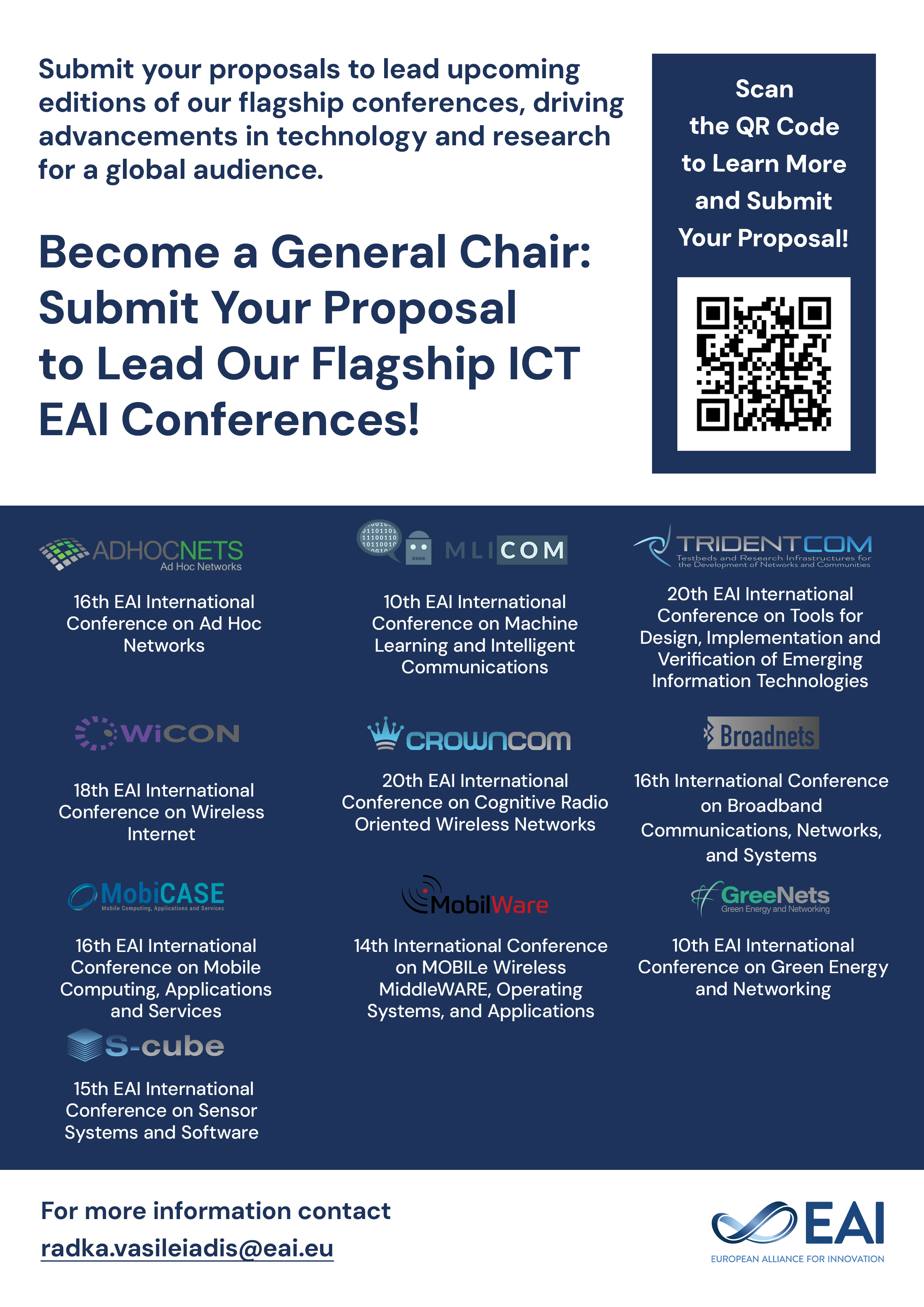
Research Article
AI Fuzzy Based Prediction and Prorogation of Alzheimer's Cancer
@ARTICLE{10.4108/eetpht.10.5478, author={Srinivas Kolli and Muniyandy Elangovan and M Vamsikrishna and Pramoda Patro}, title={AI Fuzzy Based Prediction and Prorogation of Alzheimer's Cancer}, journal={EAI Endorsed Transactions on Pervasive Health and Technology}, volume={10}, number={1}, publisher={EAI}, journal_a={PHAT}, year={2024}, month={3}, keywords={Alzheimer's cancer, big data, artificial intelligence, computer-aided diagnosis, Fuzzy Models, Machine Learning}, doi={10.4108/eetpht.10.5478} }
- Srinivas Kolli
Muniyandy Elangovan
M Vamsikrishna
Pramoda Patro
Year: 2024
AI Fuzzy Based Prediction and Prorogation of Alzheimer's Cancer
PHAT
EAI
DOI: 10.4108/eetpht.10.5478
Abstract
INTRODUCTION: Although decades of experimental and clinical research have shed a lot of light on the pathogenesis of Alzheimer's disease (AD), there are still a lot of questions that need to be answered. The current proliferation of open data-sharing initiatives that collect clinical, routine, and biological data from individuals with Alzheimer's disease presents a potentially boundless wealth of information about a condition. METHODS: While it is possible to hypothesize that there is no comprehensive collection of puzzle pieces, there is currently a proliferation of such initiatives. This abundance of data surpasses the cognitive capacity of humans to comprehend and interpret fully. In addition, the psychophysiology mechanisms underlying the whole biological continuum of AD may be investigated by combining Big Data collected from multi-omics studies. In this regard, Artificial Intelligence (AI) offers a robust toolbox for evaluating large, complex data sets, which might be used to gain a deeper understanding of AD. This review looks at the recent findings in the field of AD research and the possible obstacles that AI may face in the future. RESULTS: This research explores the use of CAD tools for diagnosing AD and the potential use of AI in healthcare settings. In particular, investigate the feasibility of using AI to stratify patients according to their risk of developing AD and to forecast which of these patients would benefit most from receiving personalized therapies. CONCLUSION: To improve these, fuzzy membership functions and rule bases, fuzzy models are trained using fuzzy logic and machine learning.
Copyright © 2024 S. Kolli et al., licensed to EAI. This is an open access article distributed under the terms of the CC BY-NCSA 4.0, which permits copying, redistributing, remixing, transformation, and building upon the material in any medium so long as the original work is properly cited.