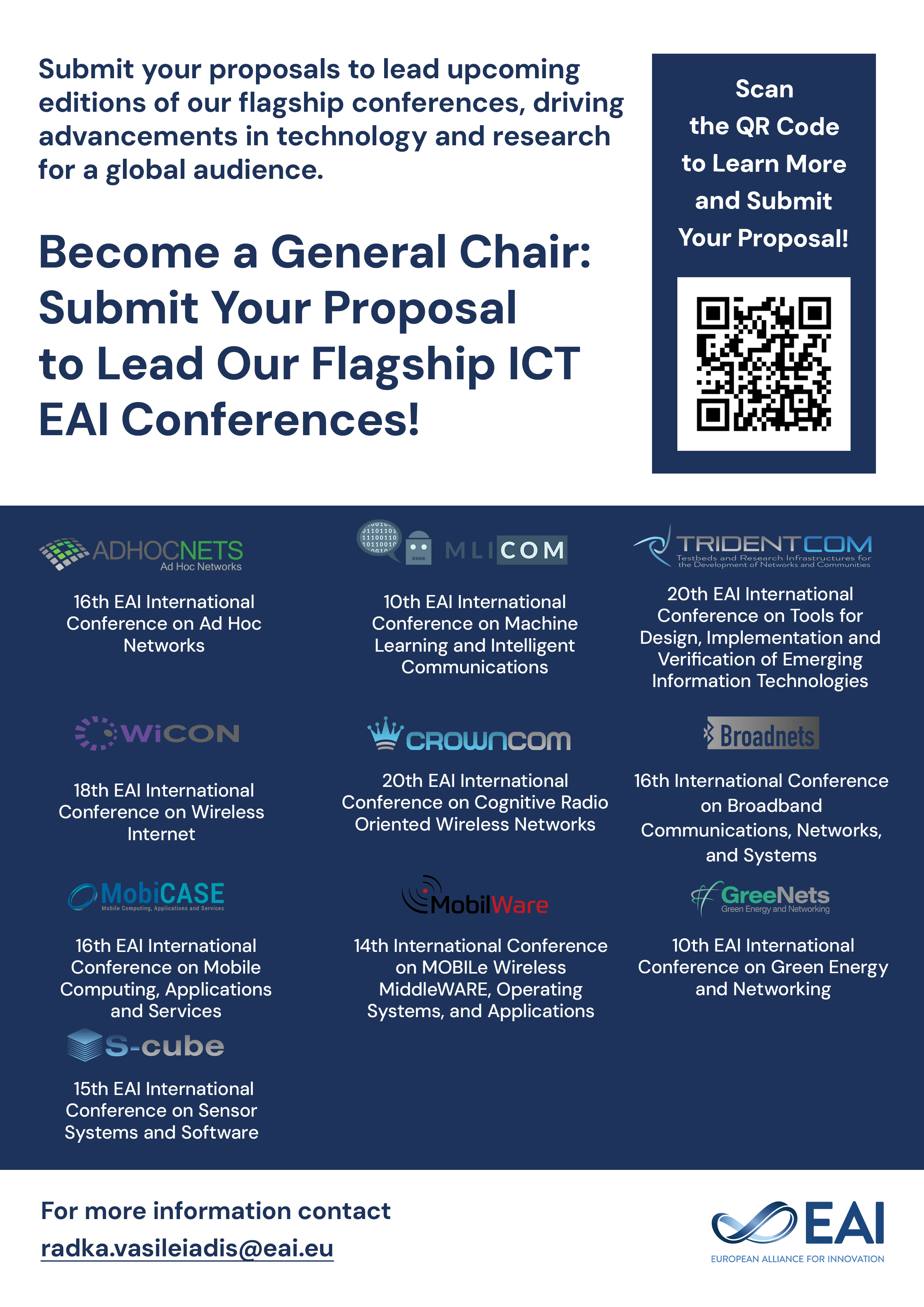
Research Article
A Step Towards Automated Haematology: DL Models for Blood Cell Detection and Classification
@ARTICLE{10.4108/eetpht.10.5477, author={Mohammed Altaf Ahmed and Donepudi Rohini and A Manjula and Abdus Sobur}, title={A Step Towards Automated Haematology: DL Models for Blood Cell Detection and Classification}, journal={EAI Endorsed Transactions on Pervasive Health and Technology}, volume={10}, number={1}, publisher={EAI}, journal_a={PHAT}, year={2024}, month={3}, keywords={Medical Imaging, Diagnostic Methodologies, Blood Cell Classification, Hematology, ResNet50, AlexNet, MobileNetV2, VGG16, VGG19}, doi={10.4108/eetpht.10.5477} }
- Mohammed Altaf Ahmed
Donepudi Rohini
A Manjula
Abdus Sobur
Year: 2024
A Step Towards Automated Haematology: DL Models for Blood Cell Detection and Classification
PHAT
EAI
DOI: 10.4108/eetpht.10.5477
Abstract
INTRODUCTION: Deep Learning has significantly impacted various domains, including medical imaging and diagnostics, by enabling accurate classification tasks. This research focuses on leveraging deep learning models to automate the classification of different blood cell types, thus advancing hematology practices. OBJECTIVES: The primary objective of this study is to evaluate the performance of five deep learning models - ResNet50, AlexNet, MobileNetV2, VGG16, and VGG19 - in accurately discerning and classifying distinct blood cell categories: Eosinophils, Lymphocytes, Monocytes, and Neutrophils. The study aims to identify the most effective model for automating hematology processes. METHODS: A comprehensive dataset containing approximately 8,500 augmented images of the four blood cell types is utilized for training and evaluation. The deep learning models undergo extensive training using this dataset. Performance assessment is conducted using various metrics including accuracy, precision, recall, and F1-score. RESULTS: The VGG19 model emerges as the top performer, achieving an impressive accuracy of 99% with near-perfect precision and recall across all cell types. This indicates its robustness and effectiveness in automated blood cell classification tasks. Other models, while demonstrating competence, do not match the performance levels attained by VGG19. CONCLUSION: This research underscores the potential of deep learning in automating and enhancing the accuracy of blood cell classification, thereby addressing the labor-intensive and error-prone nature of traditional methods in hematology. The superiority of the VGG19 model highlights its suitability for practical implementation in real-world scenarios. However, further investigation is warranted to comprehend model performance variations and ensure generalization to unseen data. Overall, this study serves as a crucial step towards broader applications of artificial intelligence in medical diagnostics, particularly in the realm of automated hematology, fostering advancements in healthcare technology.
Copyright © 2024 I. S. Rahat et al., licensed to EAI. This is an open access article distributed under the terms of the CC BY-NC-SA 4.0, which permits copying, redistributing, remixing, transformation, and building upon the material in any medium so long as the original work is properly cited.