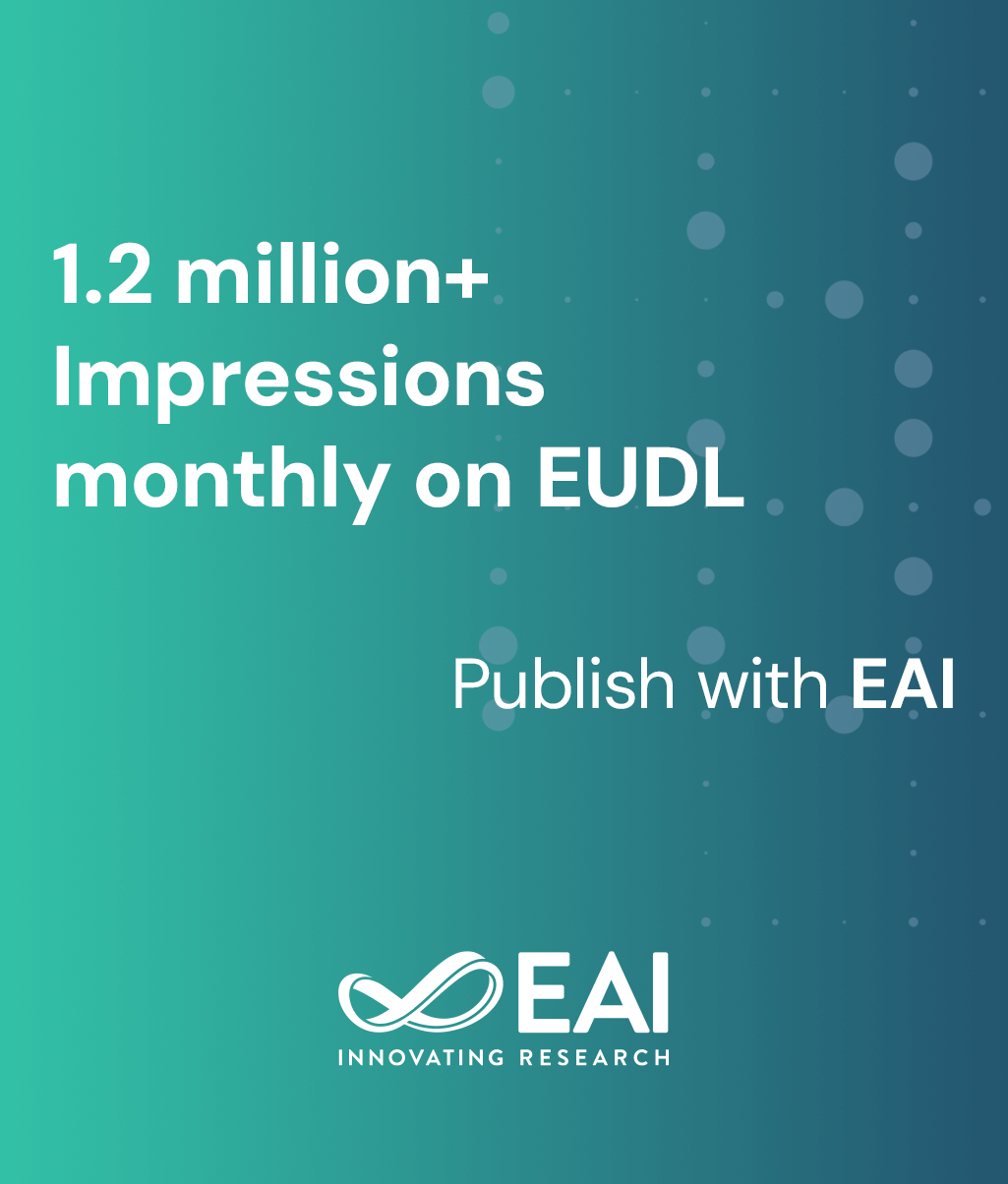
Research Article
Clinical Support System for Cardiovascular Disease Forecasting Using ECG
@ARTICLE{10.4108/eetpht.10.5455, author={Mohammed Altaf Ahmed and Q S Tasmeem Naz and Raghav Agarwal and Mannava Yesubabu and Rajesh Tulasi}, title={Clinical Support System for Cardiovascular Disease Forecasting Using ECG}, journal={EAI Endorsed Transactions on Pervasive Health and Technology}, volume={10}, number={1}, publisher={EAI}, journal_a={PHAT}, year={2024}, month={3}, keywords={Machine Learning, heart failure, decision support system, ensemble classifiers, cross-validation}, doi={10.4108/eetpht.10.5455} }
- Mohammed Altaf Ahmed
Q S Tasmeem Naz
Raghav Agarwal
Mannava Yesubabu
Rajesh Tulasi
Year: 2024
Clinical Support System for Cardiovascular Disease Forecasting Using ECG
PHAT
EAI
DOI: 10.4108/eetpht.10.5455
Abstract
INTRODUCTION: Heart failure is a chronic condition that affects many people worldwide. Regrettably, it is now the biggest cause of mortality globally, and it is becoming more common. Before a cardiac event, early diagnosis of heart disease is challenging. Although healthcare institutions like hospitals and clinics have access to a wealth of heart disease data, it is rarely used to uncover underlying trends. OBJECTIVES: Algorithms for machine learning (ML) can turn this medical data into insightful information. These methods are used to create decision support systems (DSS) that can gain knowledge from the past and advance. It is essential to use an effective ML-based technique to identify early heart failure and take preventive action to address this worldwide issue. Accurately identifying heart illness is our main goal in this study. METHODS: For this work, we benchmark different datasets on heart illness, and we use feature engineering approaches to pick the most pertinent qualities for improved performance. Additionally, we assess nine ML methods using critical parameters including precision, f-measure, sensitivity, specificity, and accuracy. RESULTS: Iterative tests are carried out to evaluate the efficacy of different algorithms. With a flawless cross-validation accuracy score of 99.51% and 100% in all other metrics, our suggested Decision Tree approach performs better than other ML models and cutting-edge studies. CONCLUSION: Each methodology used in our study is validated using cross-validation techniques. The medical community benefits greatly from this research study.
Copyright © 2024 M. A. Ahmed et al., licensed to EAI. This is an open access article distributed under the terms of the CC BY-NCSA 4.0, which permits copying, redistributing, remixing, transformation, and building upon the material in any medium so long as the original work is properly cited.