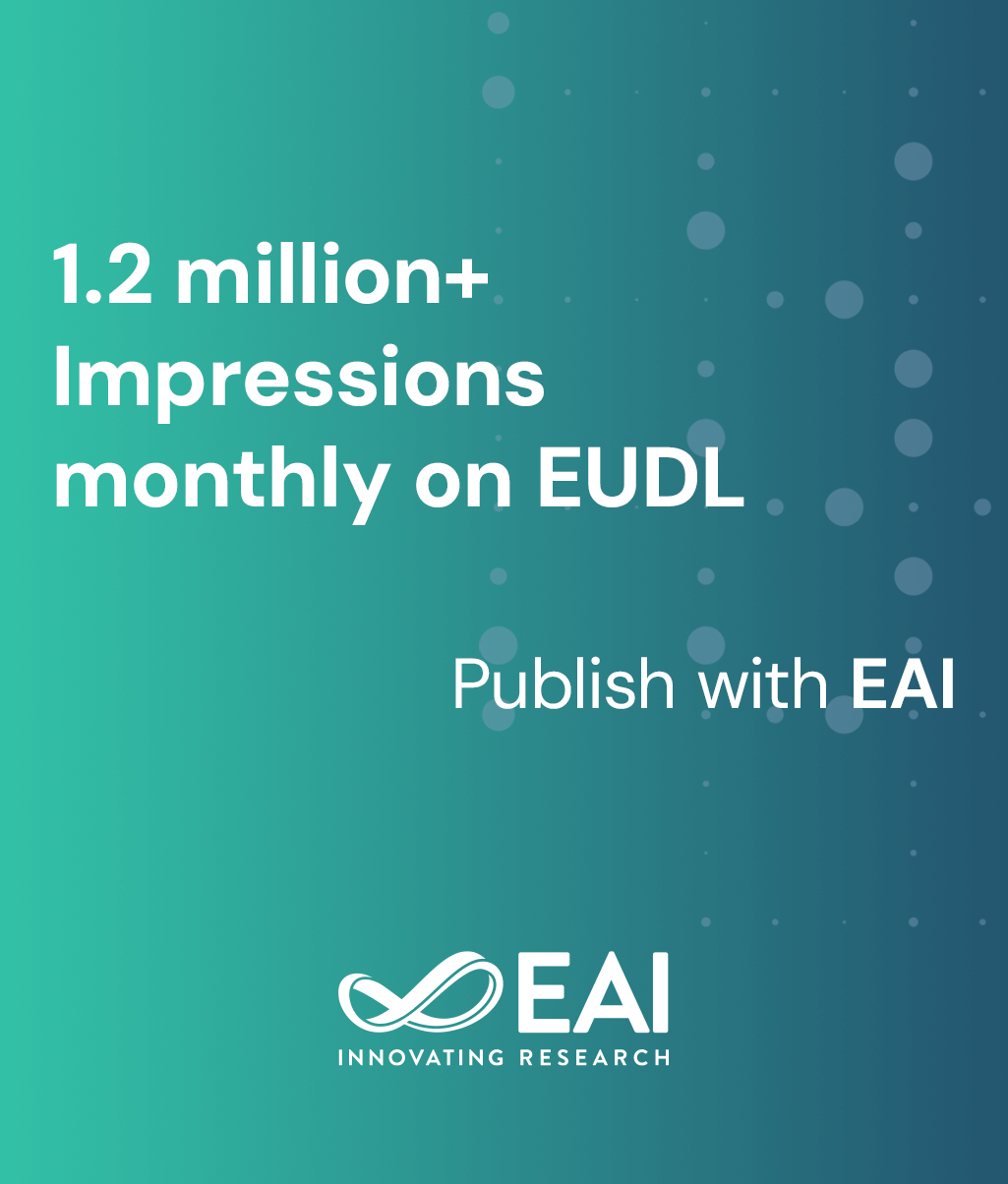
Editorial
Automated Life Stage Classification of Malaria Using Deep Learning
@ARTICLE{10.4108/eetpht.10.5439, author={Janjhyam Venkata Naga Ramesh and Raghav Agarwal and Harshitha Jyasta and Bommisetty Sivani and Palacholla Anuradha Sri Tulasi Mounika and Bollineni Bhargavi}, title={Automated Life Stage Classification of Malaria Using Deep Learning}, journal={EAI Endorsed Transactions on Pervasive Health and Technology}, volume={10}, number={1}, publisher={EAI}, journal_a={PHAT}, year={2024}, month={12}, keywords={Deep learning, Malaria microscopic cell images, life stage classification, Blood smear}, doi={10.4108/eetpht.10.5439} }
- Janjhyam Venkata Naga Ramesh
Raghav Agarwal
Harshitha Jyasta
Bommisetty Sivani
Palacholla Anuradha Sri Tulasi Mounika
Bollineni Bhargavi
Year: 2024
Automated Life Stage Classification of Malaria Using Deep Learning
PHAT
EAI
DOI: 10.4108/eetpht.10.5439
Abstract
INTRODUCTION: Malaria, an infectious illness spread by mosquitoes, is a serious hazard to humans and animals, with an increasing number of cases recorded yearly. Prompt and precise diagnosis, as well as preventative actions, are critical for effectively combating this condition. Malaria is now diagnosed using standard techniques. Microscopy of blood smears, which consists of small pictures, is used by trained specialists to identify diseased cells and define their life phases. The World Health Organisation (WHO) has approved this microscopy-based malaria diagnostic method. Drawing a blood sample from the finger, pricking it, spreading it onto a clean glass slide, and allowing it to dry naturally are all steps in the method. Thin blood smears were previously used to identify parasites under the microscope, but thick blood smears are utilized when parasite levels are low. OBJECTIVES: Due to its reliance on medical knowledge, high prices, time-consuming nature, and unsatisfactory outcomes, this technique has significant disadvantages. However, as deep learning algorithms progress, these activities may be completed more effectively and with fewer human resources. METHODS: This study demonstrates the usefulness of transfer learning, a type of deep learning, in categorizing microscopic pictures of parasitized versus uninfected malaria cells. Six models were evaluated using the publicly accessible NIH dataset, proving the usefulness of the suggested technique. RESULTS: VGG19 model fared better than its competitors, obtaining 95.05% accuracy, 92.83% precision, 96.88% sensitivity, 93.46% specificity, and 94.81% F1-score. CONCLUSION: This categorization of malaria cell photos will benefit microscopists in particular, as it will improve their workflow and provide a viable alternative for detecting malaria using microscopic cell images.
Copyright © 2024 J. V. Naga Ramesh et al., licensed to EAI. This is an open access article distributed under the terms of the CC BYNC-SA 4.0, which permits copying, redistributing, remixing, transformation, and building upon the material in any medium so long as the original work is properly cited.