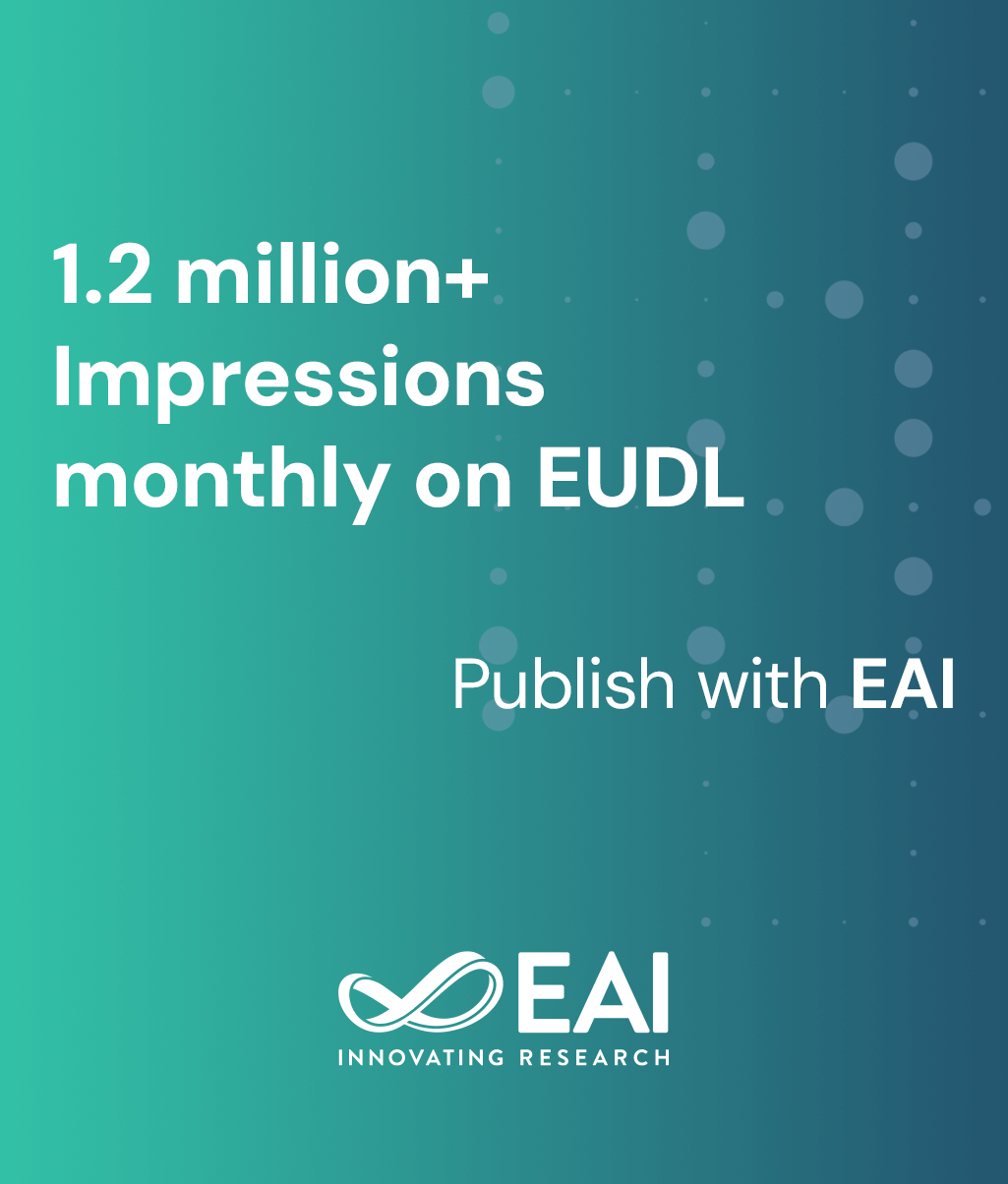
Editorial
Application of Several Transfer Learning Approach for Early Classification of Lung Cancer
@ARTICLE{10.4108/eetpht.10.5434, author={Janjhyam Venkata Naga Ramesh and Raghav Agarwal and Polireddy Deekshita and Shaik Aashik Elahi and Saladi Hima Surya Bindu and Juluru Sai Pavani}, title={Application of Several Transfer Learning Approach for Early Classification of Lung Cancer}, journal={EAI Endorsed Transactions on Pervasive Health and Technology}, volume={10}, number={1}, publisher={EAI}, journal_a={PHAT}, year={2024}, month={3}, keywords={Lung Cancer Classification, Deep Learning, Computed Tomography, CT, Transfer Learning, Clinical Decision Support System}, doi={10.4108/eetpht.10.5434} }
- Janjhyam Venkata Naga Ramesh
Raghav Agarwal
Polireddy Deekshita
Shaik Aashik Elahi
Saladi Hima Surya Bindu
Juluru Sai Pavani
Year: 2024
Application of Several Transfer Learning Approach for Early Classification of Lung Cancer
PHAT
EAI
DOI: 10.4108/eetpht.10.5434
Abstract
INTRODUCTION: Lung cancer, a fatal disease characterized by abnormal cell growth, ranks as the second most lethal worldwide, as observed in recent research conducted in India and other regions. Early detection is crucial for effective treatment, and manual differentiation of nodule types in CT images poses challenges for radiologists. OBJECTIVES: To enhance accuracy and efficiency, deep learning algorithms are proposed for early lung cancer detection. Transfer learning-based computer recognition algorithms have shown promise in providing radiologists with additional insights. METHODS: The dataset used in this study comprises 1000 CT scan images representing lung large cell carcinoma, lung adenocarcinoma, lung squamous cell carcinoma, and normal lung cases. A preprocessing phase, including picture rescaling and modification, is applied to the input CT scan images of the lungs, followed by the utilization of a specific transfer learning model to develop a lung cancer detection system. RESULTS: The performance of various transfer learning strategies is evaluated using measures such as accuracy, precision, recall, specificity, area under the curve, and F1-score. CONCLUSION: Comparative analysis indicates that VGG16 outperforms other models in accurately categorizing different types of lung cancer.
Copyright © 2024 J. K. Naga Ramesh et al., licensed to EAI. This is an open access article distributed under the terms of the CC BYNC-SA 4.0, which permits copying, redistributing, remixing, transformation, and building upon the material in any medium so long as the original work is properly cited.