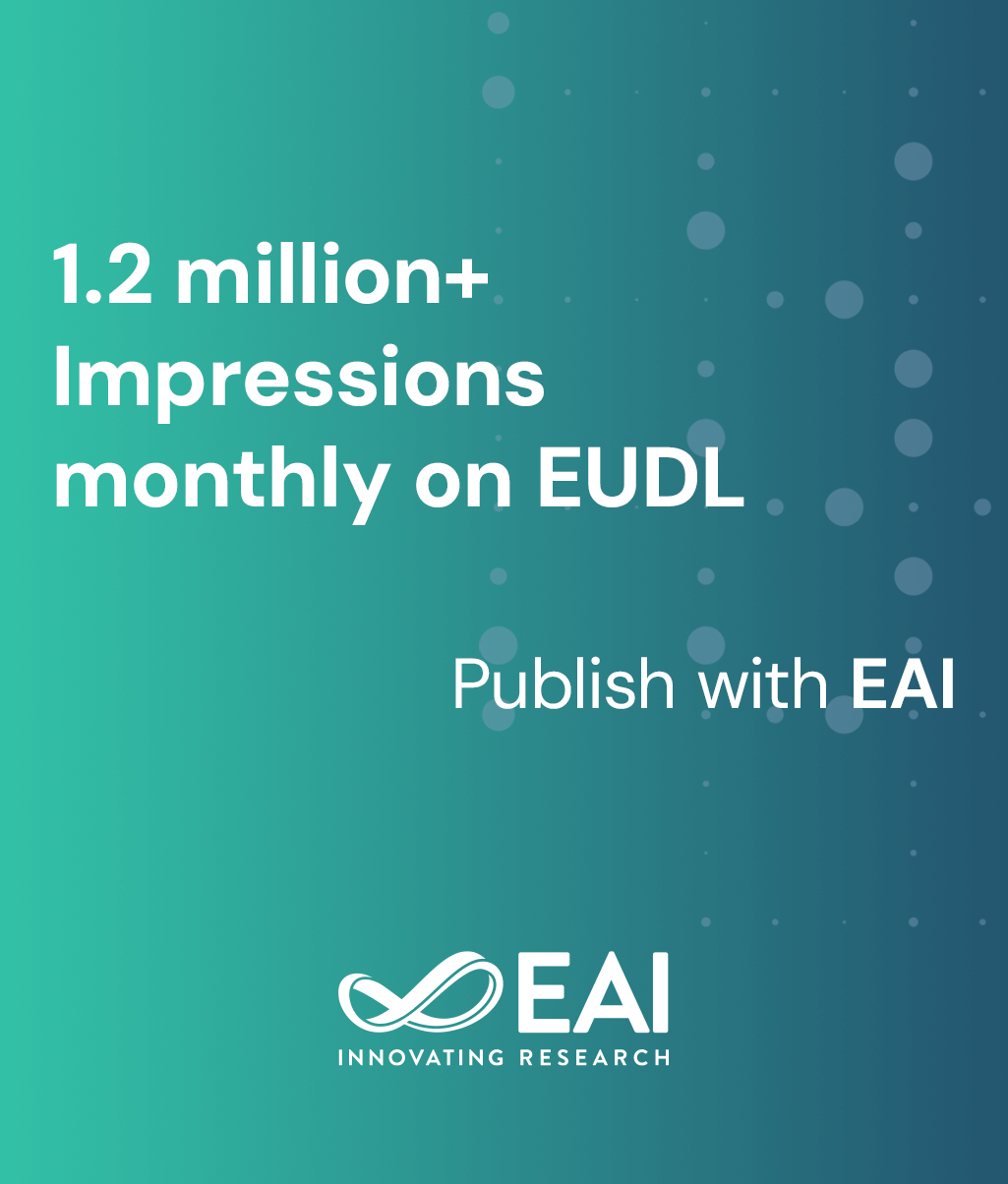
Research Article
A Multi-Model Machine Learning Approach for Monitoring Calories Being Burnt During Workouts Using Smart Calorie Tracer
@ARTICLE{10.4108/eetpht.10.5407, author={Yagnesh Challagundla and Badri Narayanan K and Krishna Sai Devatha and Bharathi V C}, title={A Multi-Model Machine Learning Approach for Monitoring Calories Being Burnt During Workouts Using Smart Calorie Tracer}, journal={EAI Endorsed Transactions on Pervasive Health and Technology}, volume={10}, number={1}, publisher={EAI}, journal_a={PHAT}, year={2024}, month={3}, keywords={Machine Learning, Calories, Neural Network, Cross-validation, Data visualization, Workouts, Fitness applications, Multi-model approach, Dataset analysis}, doi={10.4108/eetpht.10.5407} }
- Yagnesh Challagundla
Badri Narayanan K
Krishna Sai Devatha
Bharathi V C
Year: 2024
A Multi-Model Machine Learning Approach for Monitoring Calories Being Burnt During Workouts Using Smart Calorie Tracer
PHAT
EAI
DOI: 10.4108/eetpht.10.5407
Abstract
INTRODUCTION: In today's health-conscious world, accurate calorie monitoring during exercise is crucial for achieving fitness goals and maintaining a healthy lifestyle. However, existing methods often lack precision, driving the need for more reliable tracking systems. This paper explores the use of a multi-model machine learning approach to predict calorie burn during workouts by utilizing a comprehensive dataset. OBJECTIVES: The objective of this paper is to develop a user-friendly program capable of accurately predicting calorie expenditure during exercise, leveraging advanced machine learning techniques. METHODS: Techniques from social network analysis were employed to analyze the dataset, which included information on age, gender, height, weight, workout intensity, and duration. Data preprocessing involved handling missing values, eliminating irrelevant columns, and preparing features for analysis. The dataset was then divided into training and testing sets for model development and evaluation. Machine learning models, including Neural Networks, AdaBoost, Random Forest, and Gradient Boosting, were chosen based on their performance in regression tasks. RESULTS: The neural network model demonstrated superior performance in predicting calorie burn, outperforming other models in terms of MSE, RMSE, and an R2 score. Data visualization techniques aided in understanding the relationship between variables and calorie burn, highlighting the effectiveness of the neural network model. CONCLUSION: The findings suggest that a multi-model machine learning approach offers a promising solution for accurate calorie tracking during exercise. The neural network model, in particular, shows potential for developing user-friendly calorie monitoring applications. While limitations exist, such as dataset scope and environmental factors, this study lays the groundwork for future advancements in calorie monitoring and contributes to the development of holistic fitness applications.
Copyright © 2024 Y. Challagundla et al., licensed to EAI. This is an open access article distributed under the terms of the CC BY-NCSA 4.0, which permits copying, redistributing, remixing, transformation, and building upon the material in any medium so long as the original work is properly cited.