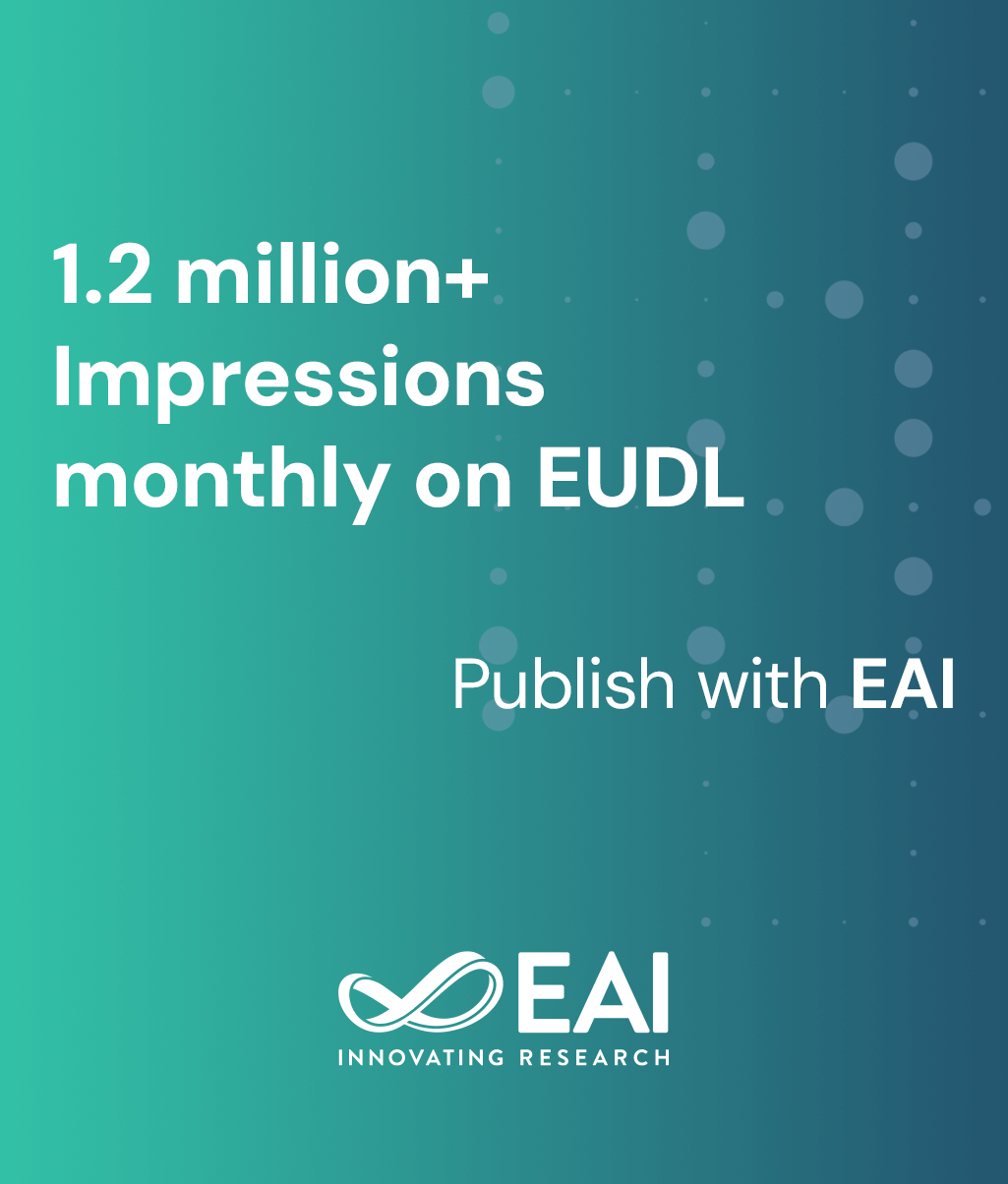
Research Article
Analyzing Machine Learning Classifiers for the Diagnosis of Heart Disease
@ARTICLE{10.4108/eetpht.10.5244, author={Saravanan Thangavel and Saravanakumar Selvaraj and Ganesh Karthikeyan V and K Keerthika}, title={Analyzing Machine Learning Classifiers for the Diagnosis of Heart Disease}, journal={EAI Endorsed Transactions on Pervasive Health and Technology}, volume={10}, number={1}, publisher={EAI}, journal_a={PHAT}, year={2024}, month={2}, keywords={Breast Heart Disease, Edge Detection Classification, Human Intelligence, Segmentation}, doi={10.4108/eetpht.10.5244} }
- Saravanan Thangavel
Saravanakumar Selvaraj
Ganesh Karthikeyan V
K Keerthika
Year: 2024
Analyzing Machine Learning Classifiers for the Diagnosis of Heart Disease
PHAT
EAI
DOI: 10.4108/eetpht.10.5244
Abstract
INTRODUCTION: Preventable deaths from cardiovascular diseases outnumber all others combined. Detecting it at an early stage is crucial. Human lives will be saved as a result. OBJECTIVES: Improved cardiac disease prediction using machine learning classifiers is the focus of this article. METHODS: We have used many different classifiers, such as the support vector machine, naive bayes, random forest, and k-nearest neighbours, to achieve this goal, even though we can’t predict high accuracy in this classifier. So, we have proposed Hyper parameter adjustment was applied to the classifiers, which increased their precision. It was possible to compare the classifiers. RESULTS: In comparison to other machine learning classifiers, Logistic Regression achieves higher prediction accuracy, at 95.5%. CONCLUSION: To help people find the nearest cardiac care facilities, Google Maps has been integrated into a responsive web application that has been built for forecasting heart illness.
Copyright © 2024 S. Thangavel et al., licensed to EAI. This is an open access article distributed under the terms of the CC BY-NC-SA 4.0, which permits copying, redistributing, remixing, transformation, and building upon the material in any medium so long as the original work is properly cited.