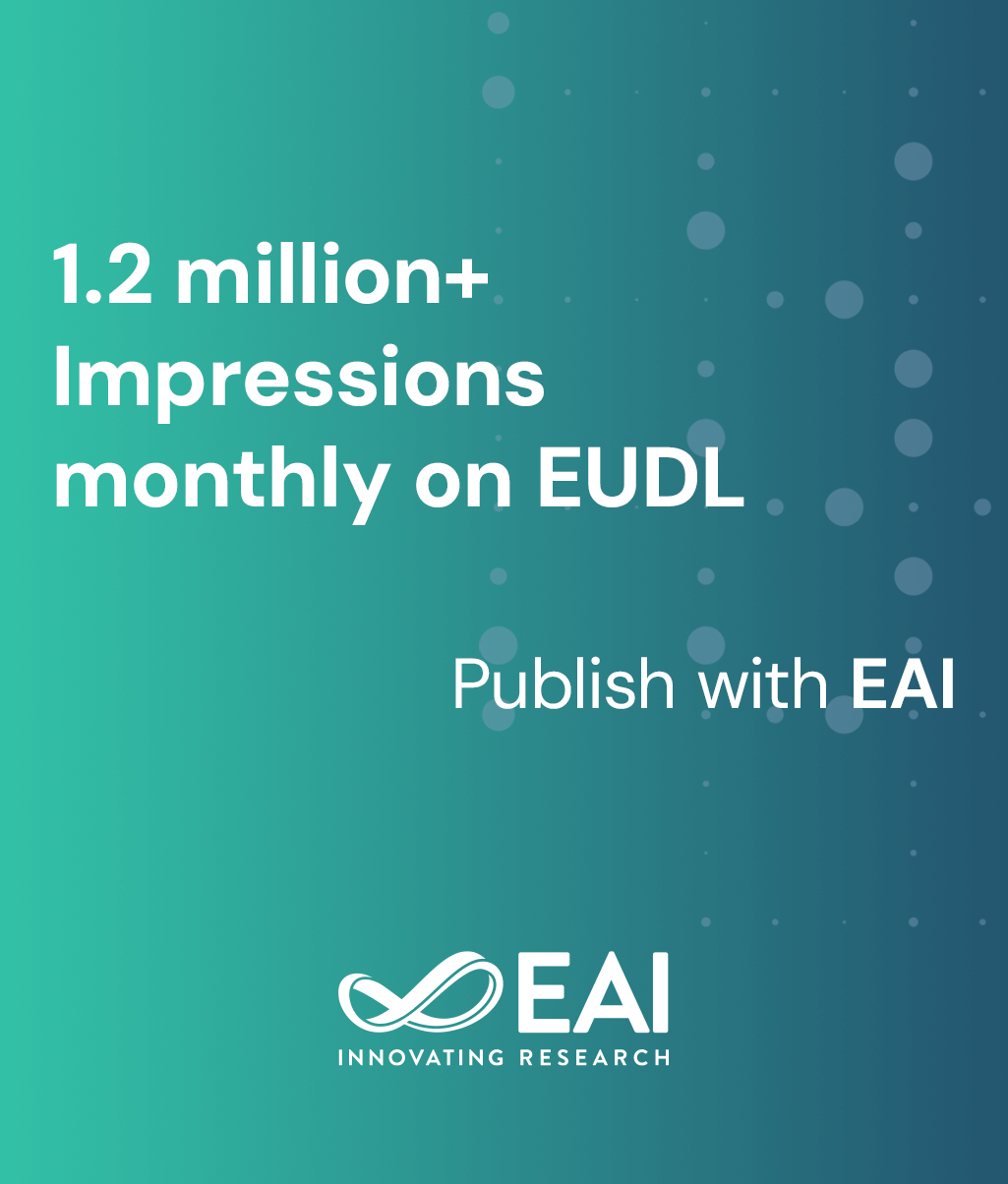
Research Article
An Innovative CBR-Enhanced Approach for Skin Cancer Classification using Cascade Forest Model and Convolutional Neural Network with Attention Mechanism
@ARTICLE{10.4108/eetpht.10.3875, author={Safa Gasmi and Akila DJEBBAR and Hayet Farida Merouani and Hanene Djedi}, title={An Innovative CBR-Enhanced Approach for Skin Cancer Classification using Cascade Forest Model and Convolutional Neural Network with Attention Mechanism}, journal={EAI Endorsed Transactions on Pervasive Health and Technology}, volume={10}, number={1}, publisher={EAI}, journal_a={PHAT}, year={2024}, month={12}, keywords={skin cancer classification, case-based reasoning (CBR), retrieval phase, convolutional neural network (CNN), ccascade forest model, attention mechanism, SMOTE technique}, doi={10.4108/eetpht.10.3875} }
- Safa Gasmi
Akila DJEBBAR
Hayet Farida Merouani
Hanene Djedi
Year: 2024
An Innovative CBR-Enhanced Approach for Skin Cancer Classification using Cascade Forest Model and Convolutional Neural Network with Attention Mechanism
PHAT
EAI
DOI: 10.4108/eetpht.10.3875
Abstract
INTRODUCTION: In recent years, skin cancer has emerged as a pressing concern, necessitating advanced diagnostic and classification techniques. OBJECTIVES: This paper introduces an innovative hybrid approach that combines deep learning and machine learning to enhance the retrieval phase of the Case-Based Reasoning (CBR) system for skin cancer classification. METHODS: The proposed approach leverages a Convolutional Neural Network (CNN) with an attention mechanism for feature extraction, which is used to build the case base. Additionally, it uses a modified cascade forest model, augmented with traditional machine learning classifiers for classification. This modified cascade forest model incorporates the XGBoost model in its initial layer to facilitate more effective ensemble learning and bolster predictive performance. Subsequently, in the following layers, it use the random forest model to capitalize on its ability to handle high-dimensional feature spaces and maintain diversity within the ensemble. RESULTS: Rigorous experimentation on the balanced HAM10000 dermoscopic image dataset, employing the Synthetic Minority Oversampling Technique (SMOTE), demonstrates the superiority of the modified cascade forest model in multi-class skin cancer classification. This model consistently achieves the highest metrics, including accuracy (95.40%), precision (95.49%), F1-Score (95.38%), and recall (95.44%). CONCLUSION: This research highlights the efficacy of the proposed model compared to other classifiers, emphasizing the significance of the modified cascade forest model in enhancing the accuracy and reliability of skin cancer classification.
Copyright © 2024 Gasmi et al., licensed to EAI. This is an open access article distributed under the terms of the CC BY-NC-SA 4.0, which permits copying, redistributing, remixing, transformation, and building upon the material in any medium so long as the original work is properly cited.