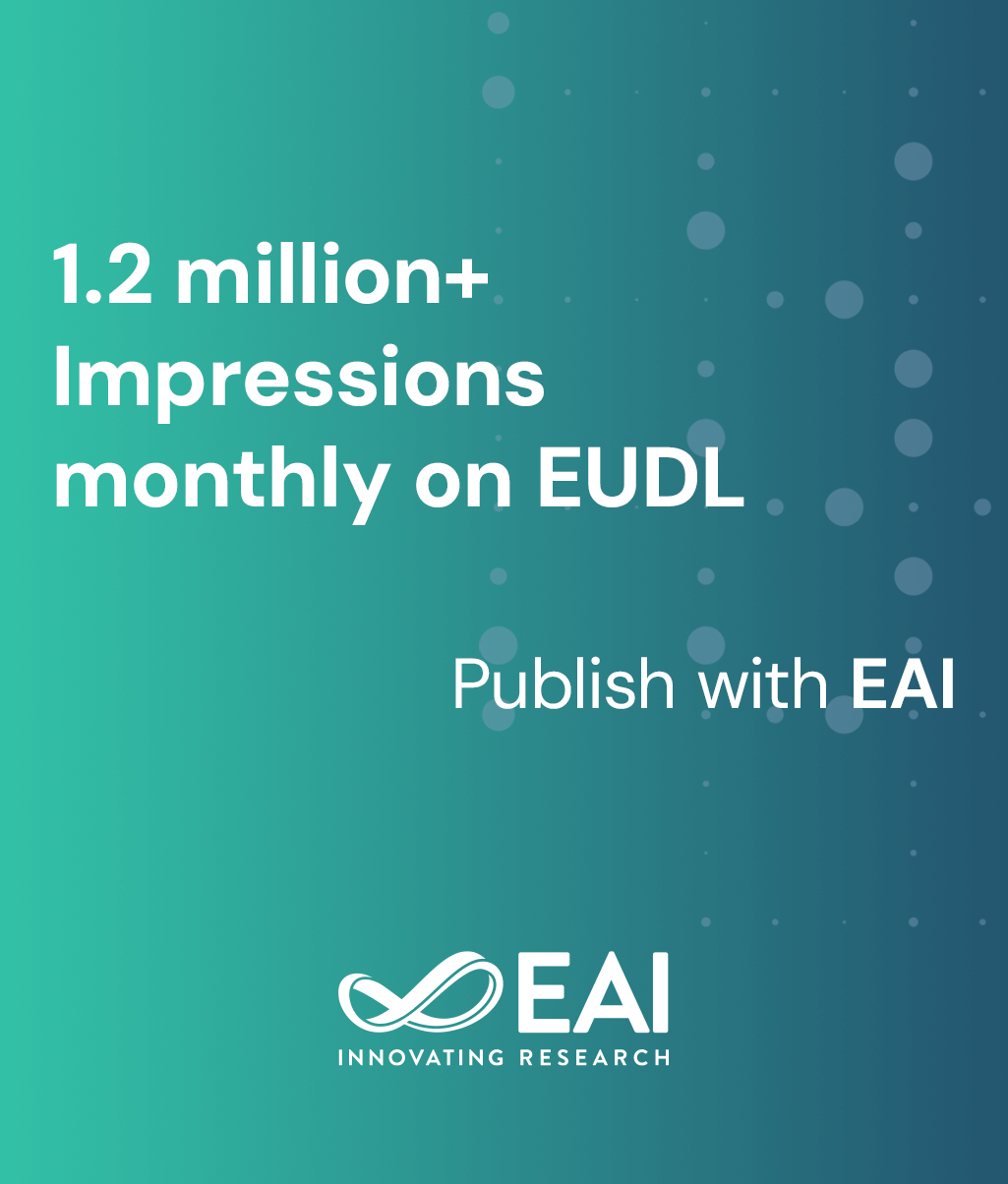
Research Article
Deep Learning Approaches for Stock Price Prediction A Comparative Study on Nifty 50 Dataset
@ARTICLE{10.4108/eetismla.7481, author={Sushma P Kallimath and Narayana Darapaneni and Anwesh Reddy Paduri}, title={Deep Learning Approaches for Stock Price Prediction A Comparative Study on Nifty 50 Dataset}, journal={EAI Endorsed Transactions on Intelligent Systems and Machine Learning}, volume={1}, number={1}, publisher={EAI}, journal_a={ISMLA}, year={2025}, month={2}, keywords={NSE50, regression models, R2 score, RMSE, MAPE, MAE}, doi={10.4108/eetismla.7481} }
- Sushma P Kallimath
Narayana Darapaneni
Anwesh Reddy Paduri
Year: 2025
Deep Learning Approaches for Stock Price Prediction A Comparative Study on Nifty 50 Dataset
ISMLA
EAI
DOI: 10.4108/eetismla.7481
Abstract
Stock price prediction is essential for investors and traders in financial markets. Deep learning methods have emerged as promising tools for capturing intricate patterns in stock market data. In this paper, we explore a comprehensive comparative study of various deep learning architectures for stock price prediction using the Nifty 50 dataset. The models evaluated include Linear regression, LSTM, GRU, CNN, RNN, Temporal Convolutional Network (TCN), as well as combination models such as LSTM+GRU, CNN+RNN, CNN+TCN, and LSTM+TCN. Our study aims to evaluate how well they perform and suitability of these methodologies in capturing the dynamics of stock price movements. Utilizing historical Nifty 50 data spanning multiple years, we evaluate the models’ predictive capabilities using standard evaluation metrics such as MSE, R2 Score, RMSE, MAE, and MAPE. Results from our experiments unveil distinct strengths and weaknesses among the different deep learning architectures. While linear regression provides a baseline for comparison, deep learning models like LSTM, GRU, CNN, RNN, and TCN exhibit superior performance in capturing the nonlinear and time-varying nature of stock market data. Additionally, hybrid architectures demonstrate promising results by leveraging the complementary strengths of individual models. This comparative study offers meaningful perspectives on the effectiveness of various deep learning approaches for stock price prediction, which can benefit researchers, practitioners, and stakeholders in the financial domain. By understanding the performance characteristics of these models, stakeholders can make informed decisions in their investment strategies.
Copyright © 2025 S. P. Kallimath et al., licensed to EAI. This is an open access article distributed under the terms of the Creative Commons Attribution license (http://creativecommons.org/licenses/by/4.0/), which permits unlimited use, distribution and reproduction in any medium so long as the original work is properly cited.