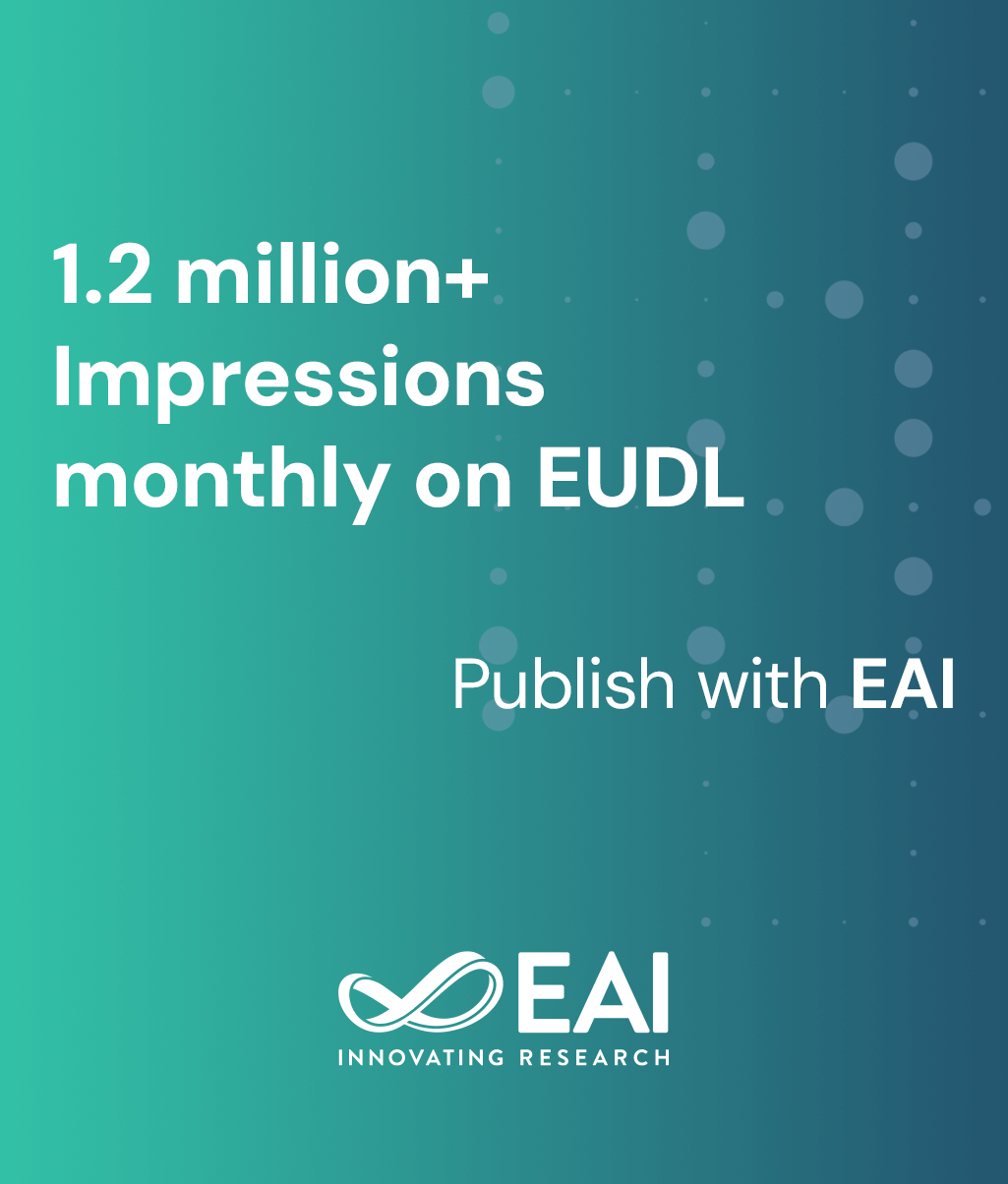
Research Article
Exploring The Efficiency of Metaheuristics in Optimal Hyperparameter Tuning for Ensemble Models on Varied Data Modalities
@ARTICLE{10.4108/eetismla.6461, author={Vivek BC }, title={Exploring The Efficiency of Metaheuristics in Optimal Hyperparameter Tuning for Ensemble Models on Varied Data Modalities}, journal={EAI Endorsed Transactions on Intelligent Systems and Machine Learning}, volume={1}, number={1}, publisher={EAI}, journal_a={ISMLA}, year={2024}, month={8}, keywords={Kidney disease dataset, heart disease dataset, breast-cancer dataset, diabetes dataset, SVM, KNN, Na\~{n}ve Bayes, Logistic Regression, Random Search, Grid Search, PSO}, doi={10.4108/eetismla.6461} }
- Vivek BC
Year: 2024
Exploring The Efficiency of Metaheuristics in Optimal Hyperparameter Tuning for Ensemble Models on Varied Data Modalities
ISMLA
EAI
DOI: 10.4108/eetismla.6461
Abstract
Effective disease detection systems play an important role in healthcare by supporting diagnosis and treatment. This study provides a comparison of hyperparameter tuning methods for disease detection systems using four health datasets; kidney disease, diabetes detection, heart disease and breast cancer detection. The main objective of this research is to prepare datasets by normalizing the input and testing machine learning models such as Naive Bayes Support Vector Machine (SVM), Logistic Regression and k Nearest Neighbor (kNN). to identify effective models for each data set. After implementing the models, we apply three hyperparameter tuning techniques: Grid search, random search, and particle ensemble optimization (PSO). These methods are used to tune the model parameters. Improve overall performance metrics. The evaluation focuses on accuracy measurements to compare model performance before and after hyperparameter tuning. The results of this study illustrate how different tuning techniques can improve the performance of disease detection systems across a range of healthcare datasets. By conducting testing and analysis, we determine the appropriate tuning method for each data set, yielding valuable insights, to develop an accurate and effective disease detection system .These discoveries serve to advance the field of healthcare analytics and machine learning to deliver outcomes for patients and healthcare services.
Copyright © 2024 Vivek BC., licensed to EAI. This is an open access article distributed under the terms of the CC BY-NC-SA 4.0, which permits copying, redistributing, remixing, transformation, and building upon the material in any medium so long as the original work is properly cited.