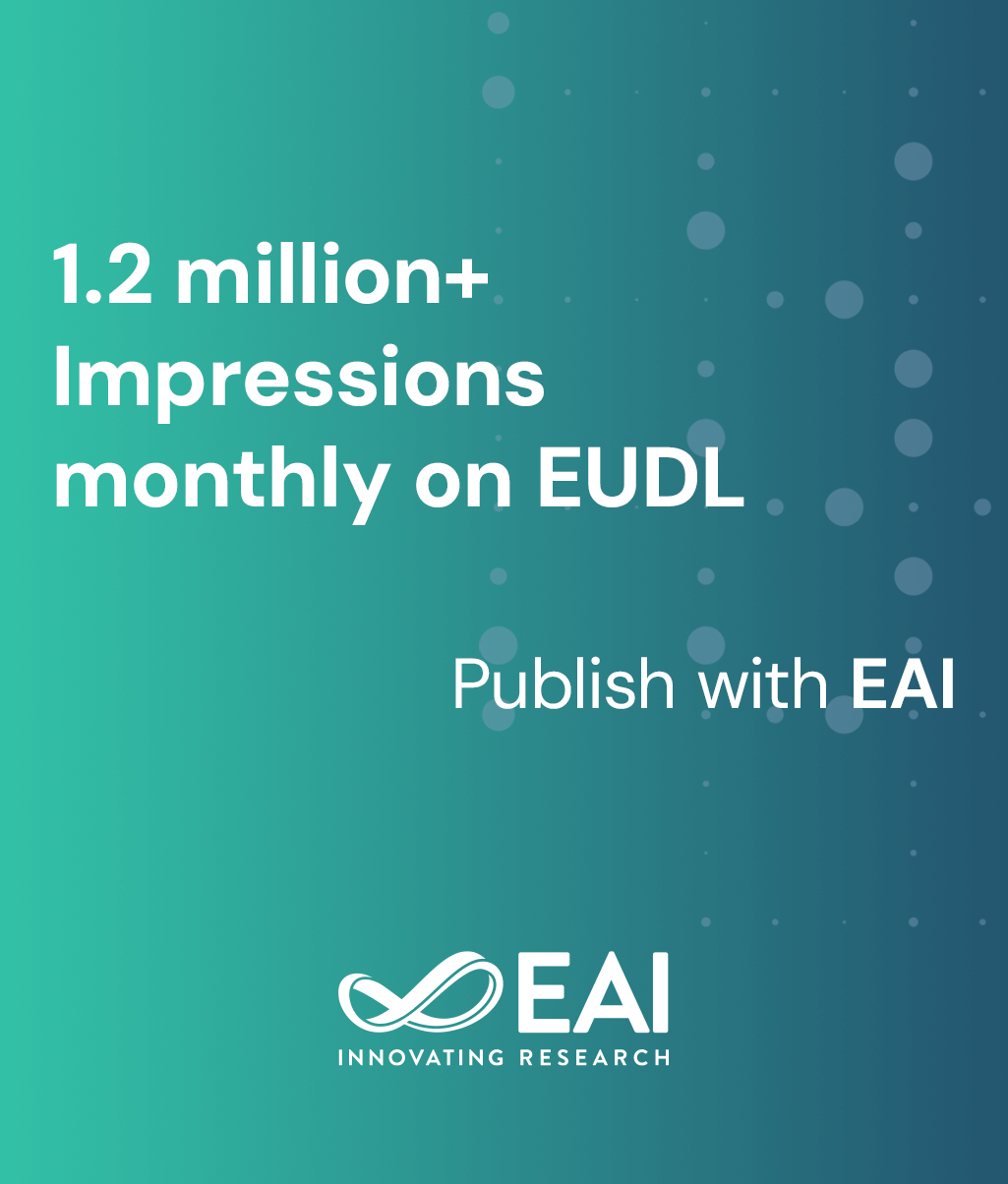
Research Article
Detection, Localization of Cardiomegaly and TB Disease of CXR Images using Deep Learning
@ARTICLE{10.4108/eetismla.4066, author={Ganesh Pradeep P V and Dinesh R and Anwesh Reddy Paduri and Narayana Darapaneni}, title={Detection, Localization of Cardiomegaly and TB Disease of CXR Images using Deep Learning}, journal={EAI Endorsed Transactions on Intelligent Systems and Machine Learning}, volume={1}, number={1}, publisher={EAI}, journal_a={ISMLA}, year={2024}, month={7}, keywords={CNN architectures, Image Classification, Image segmentation, Tuberculosis, Cardiomegaly, Disease Localization}, doi={10.4108/eetismla.4066} }
- Ganesh Pradeep P V
Dinesh R
Anwesh Reddy Paduri
Narayana Darapaneni
Year: 2024
Detection, Localization of Cardiomegaly and TB Disease of CXR Images using Deep Learning
ISMLA
EAI
DOI: 10.4108/eetismla.4066
Abstract
INTRODUCTION: Tuberculosis (TB) continues to pose a significant worldwide public health concern, as it stands as the primary contributor to mortality stemming from infectious illnesses. Cardiomegaly, characterized by an enlarged heart, poses medical concern as well. OBJECTIVES: Timely identification of Cardiomegaly is vital for effective management. Chest X-ray diagnosis is an easily available method with less radiation exposure to detect several lung infections and heart enlargement. Utilizing computer-aided diagnostic systems can aid in the early detection of lung conditions and the enlargement of the heart. METHODS: We worked on different state-of-the-art CNN architectures such as VGG, DenseNet and EfficientNet with customization over dataset generated from combination of multiple publicly available datasets, which consists of 12939 annotated images across three different categories, one being normal and other two being TB and cardiomegaly diseases.. RESULTS: EfficientNetB5 with optimization has shown excellent results amongst others in classifying Tuberculosis and Cardiomegaly with a remarkable accuracy of 97%. CONCLUSION: The proposed model is ready for clinical diagnosis and triaging of X-ray images. Our solution also offers efficient ways to show the presence of the above diseases using Grad-CAM technique.
Copyright © 2024 Ganesh Pradeep P V et al., licensed to EAI. This is an open access article distributed under the terms of the CC BYNC-SA 4.0, which permits copying, redistributing, remixing, transformation, and building upon the material in any medium so long as the original work is properly cited.