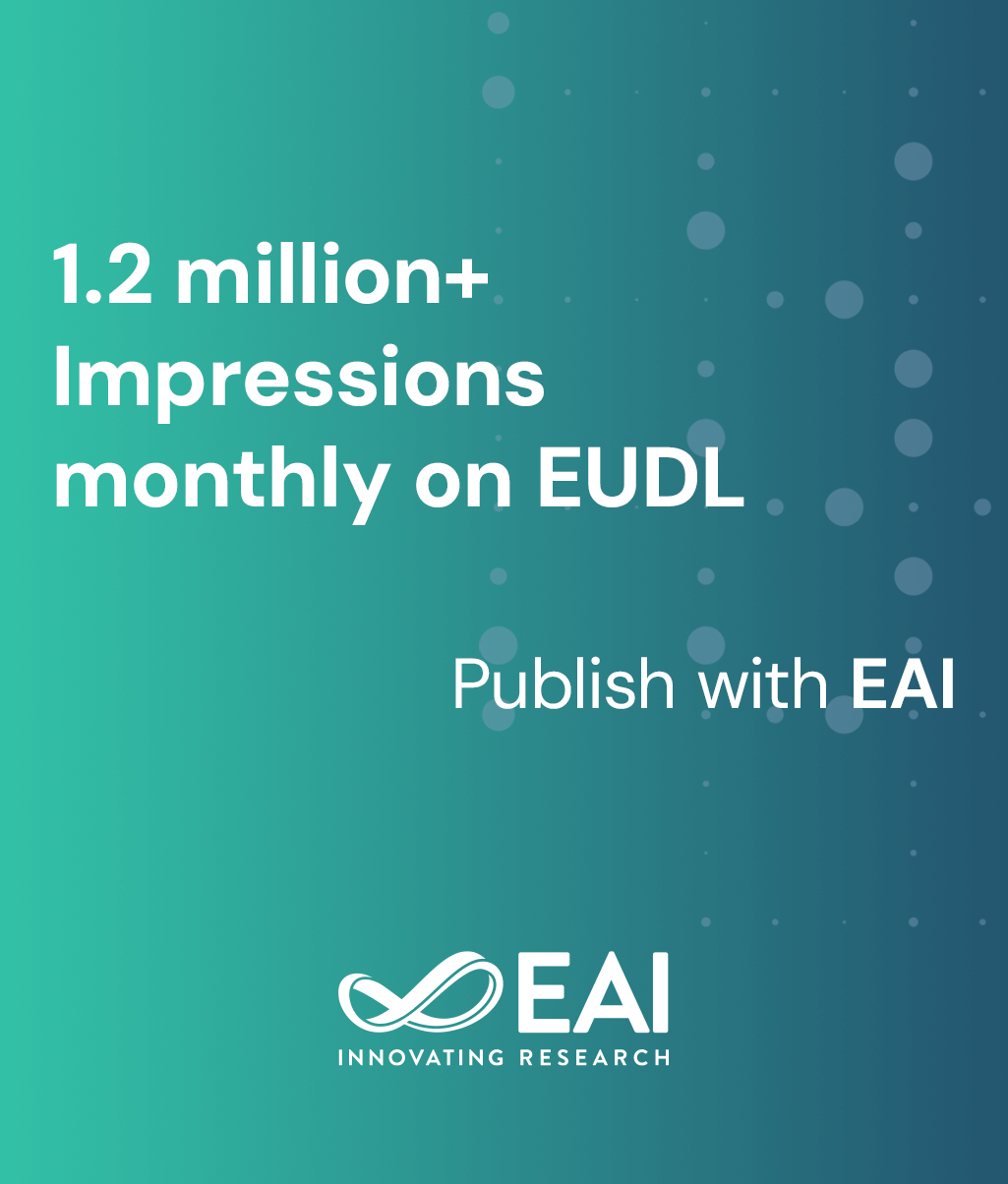
Research Article
Neural Networks Application for the Data of LQG Regulator for the Flexible Joint Robot
@ARTICLE{10.4108/eetcs.v7i22.2856, author={Nguyen Cong Danh}, title={Neural Networks Application for the Data of LQG Regulator for the Flexible Joint Robot}, journal={EAI Endorsed Transactions on Cloud Systems}, volume={7}, number={22}, publisher={EAI}, journal_a={CS}, year={2022}, month={11}, keywords={flexible joint, LQG, joint robot, arm robot, neural networks}, doi={10.4108/eetcs.v7i22.2856} }
- Nguyen Cong Danh
Year: 2022
Neural Networks Application for the Data of LQG Regulator for the Flexible Joint Robot
CS
EAI
DOI: 10.4108/eetcs.v7i22.2856
Abstract
This paper describes the investigation of a flexible robotic arm with Degree of Freedom (DOF) through Linear Quadratic Gaussian (LQG) regulator under the influence of noise signals with using diagrams below. The derived model is based on the Euler-Lagrange approach while LQG mode is proposed as a modern control method. A flexible robotic arm that is test in the modern regulator is essential for applications. This is even more interesting while these arms have joints that work independently of each other, but they still ensure a tight connection between the joints. Testing the stability characteristics of the system through this regulator helps readers determine the output signals of a system exactly. From here, the author came up with strategies the match the requirements. Although the initial stage of the operating cycle is not stable with the use of LQG regulator, most of later stages of this process of closed systems of robotic systems gradually become stable. In particular, adjusting LQG for flexible joint robot gives satisfactory simulation results. This promises a positive result of other joint robot. Neural networks applied in this paper have proven its effectiveness in information security issues. It is also very useful for tight control of possible situations such as a large scale attack on network systems, automated control systems. The main contributions of this paper have been clearly demonstrated through two strategies that need to be addressed: LQG, neural networks for the data of LQG regulator.The methods described above have not been previously published for this model. Therefore, this article was born to outline the above objectives and the plans have been satisfactorily implemented. These studies are important because they serve as a necessary database in the selection of control strategies according to the requirements. Simulation results are done by Matlab. The purpose of the study of this paper: the author has searched for a stability of the system through the above methods. The applied method is a thorough study of the structure of the system as well as the construction of algorithms to form the regulator. The result ia a reliable simulation of events after the system is controlled by these regulators.
Copyright © 2022 Nguyen Cong Danh, licensed to EAI. This is an open access article distributed under the terms of the CC BY-NCSA 4.0, which permits copying, redistributing, remixing, transformation, and building upon the material in any medium so long as the original work is properly cited.