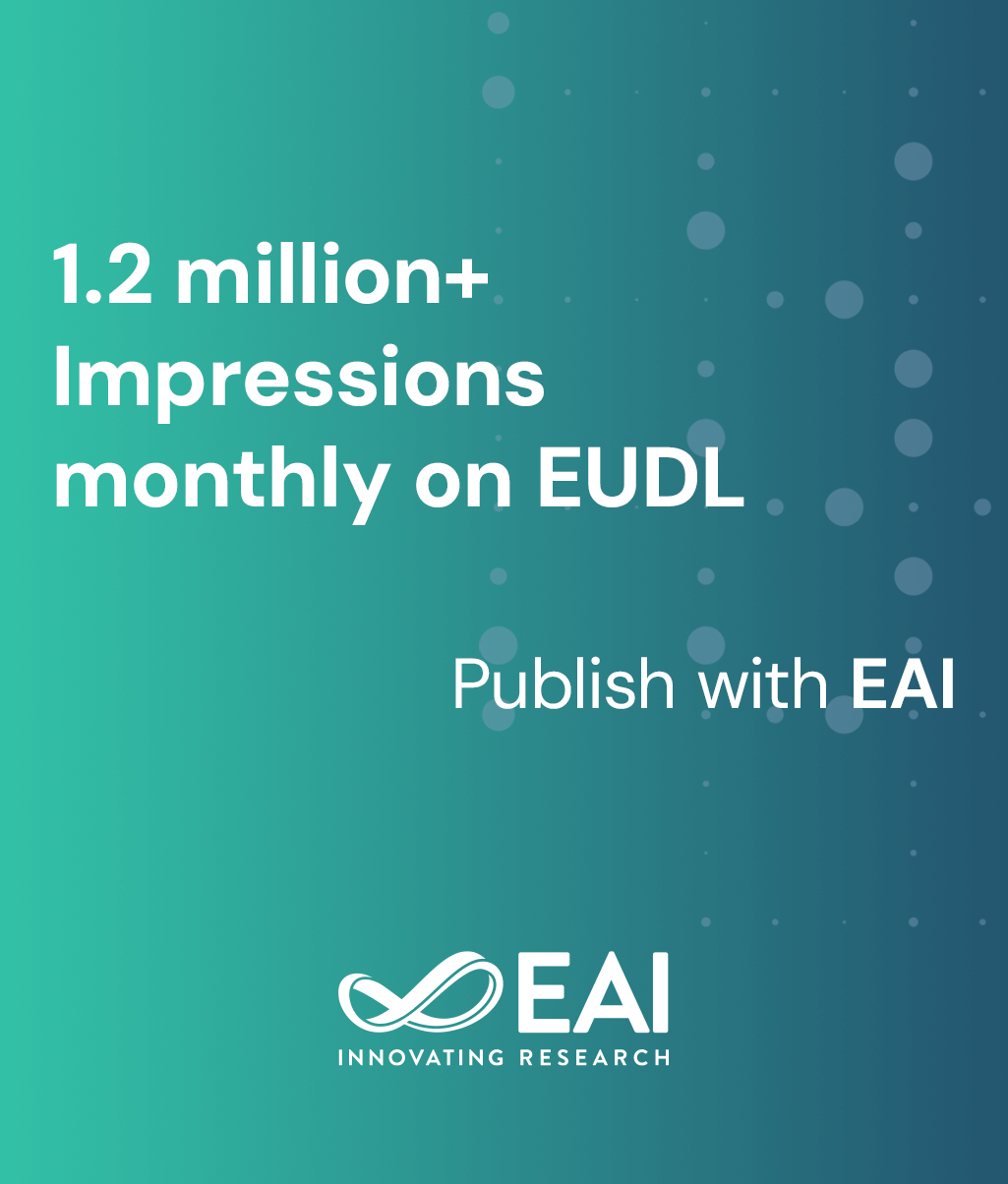
Research Article
Enhanced Diagnosis of Influenza and COVID-19 Using Machine Learning
@ARTICLE{10.4108/eetcasa.v9i1.4030, author={Dang Nhu Phu and Phan Cong Vinh and Nguyen Kim Quoc}, title={Enhanced Diagnosis of Influenza and COVID-19 Using Machine Learning}, journal={EAI Endorsed Transactions on Context-aware Systems and Applications}, volume={9}, number={1}, publisher={EAI}, journal_a={CASA}, year={2023}, month={10}, keywords={COVID-19, influenza-like illness, machine learning, predictive modeling, xgboost, random forest, diagnostic parameters, clinical symptoms, disease detection, pandemic response strategies}, doi={10.4108/eetcasa.v9i1.4030} }
- Dang Nhu Phu
Phan Cong Vinh
Nguyen Kim Quoc
Year: 2023
Enhanced Diagnosis of Influenza and COVID-19 Using Machine Learning
CASA
EAI
DOI: 10.4108/eetcasa.v9i1.4030
Abstract
The Coronavirus Disease 2019 (COVID-19) has rapidly spread globally, causing a significant impact on public health. This study proposes a predictive model employing machine learning techniques to distinguish between influenza-like illness and COVID-19 based on clinical symptoms and diagnostic parameters. Leveraging a dataset sourced from BMC Med Inform Decis Mak, comprising cases of influenza and COVID-19, we explore a diverse set of features, including clinical symptoms and blood assay parameters. Two prominent machine learning algorithms, XGBoost and Random Forest, are employed and compared for their predictive capabilities. The XGBoost model, in particular, demonstrates superior accuracy with an AUC under the ROC curve of 98.8%, showcasing its potential for clinical diagnosis, especially in settings with limited specialized testing equipment. Our model's practical applicability in community-based testing positions it as a valuable tool for efficient COVID-19 detection. This study advances the field of predictive modeling for disease detection, offering promising prospects for improved public health outcomes and pandemic response strategies. The model's reliability and effectiveness make it a valuable asset in the ongoing fight against the COVID-19 pandemic.
Copyright © 2023 D. N. Phu et al., licensed to EAI. This is an open access article distributed under the terms of the CC BY-NC-SA 4.0, which permits copying, redistributing, remixing, transformation, and building upon the material in any medium so long as the original work is properly cited.