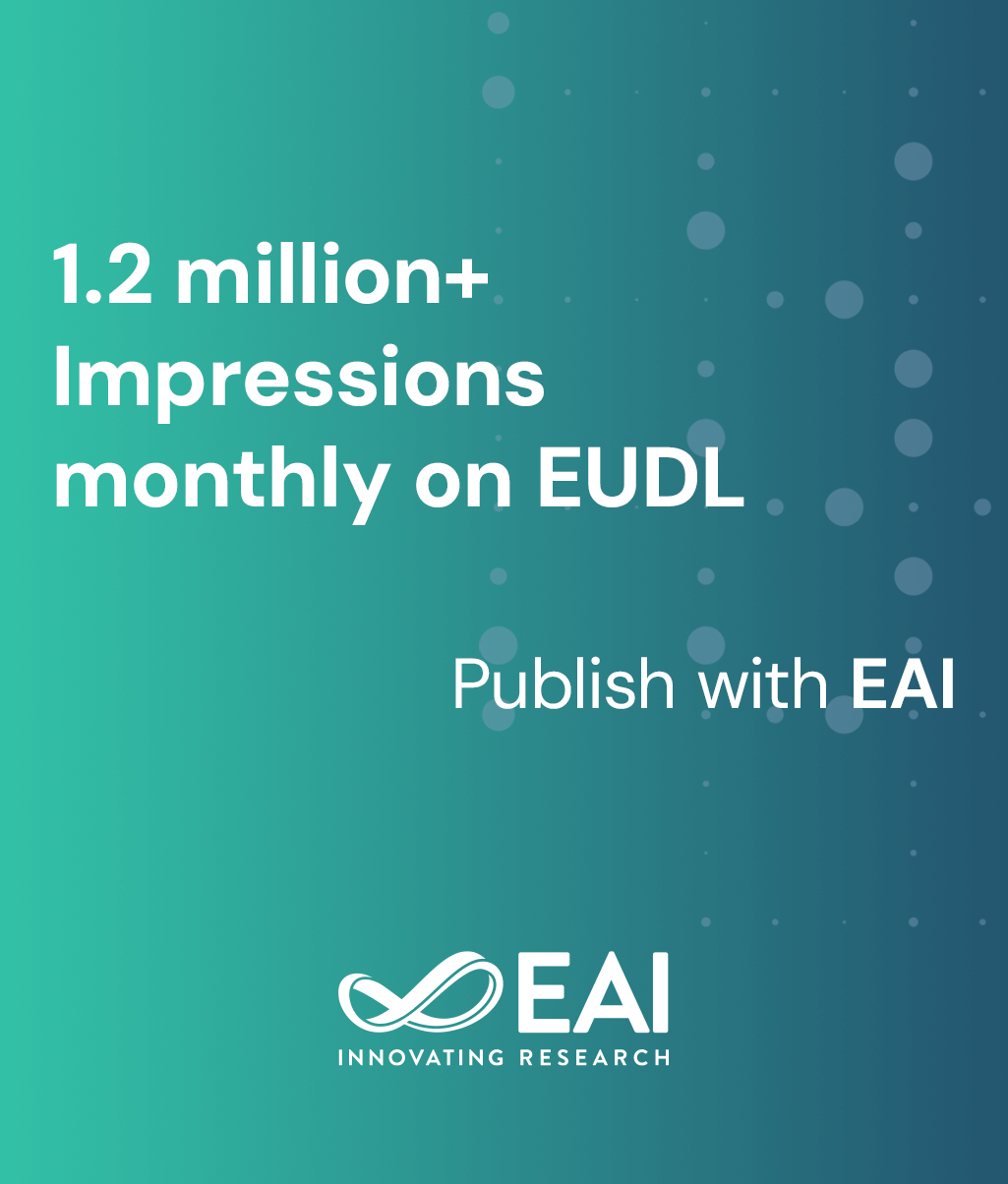
Research Article
Deep Biased Matrix Factorization for Student Performance Prediction
@ARTICLE{10.4108/eetcasa.v9i1.3147, author={Thanh-Nhan Huynh-Ly and Huy-Thap Le and Nguyen Thai-Nghe}, title={Deep Biased Matrix Factorization for Student Performance Prediction}, journal={EAI Endorsed Transactions on Context-aware Systems and Applications}, volume={9}, number={1}, publisher={EAI}, journal_a={CASA}, year={2023}, month={4}, keywords={Educational data mining, deep matrix factorization, courses recommendation, student performance prediction}, doi={10.4108/eetcasa.v9i1.3147} }
- Thanh-Nhan Huynh-Ly
Huy-Thap Le
Nguyen Thai-Nghe
Year: 2023
Deep Biased Matrix Factorization for Student Performance Prediction
CASA
EAI
DOI: 10.4108/eetcasa.v9i1.3147
Abstract
In universities that use the academic credit system, selecting elective courses is a crucial task that can have a significant impact on a student's academic performance. Students who perform poorly in their courses may receive formal warnings or even face expulsion from the university. Thus, a well-designed study plan from a course recommendation system can play an essential role in achieving good academic performance. Additionally, early warnings regarding challenging courses can help students better prepare and improve their chances of success. Therefore, predicting student performance is a vital component of both the course recommendation system and the academic advisor's role. To this end, numerous studies have addressed the prediction of student performance using various approaches such as association rules, machine learning, and recommender systems. More recently, personalized machine learning approaches, particularly the matrix factorization technique, have been used in the course recommendation system. However, the accuracy of these approaches in predicting student performance still needs improvement. To address this issue, this study proposes an approach called Deep Biased Matrix Factorization, which carries out deep factorization via multi-layer to enhance prediction accuracy. Experimental results on an educational dataset have demonstrated that the proposed approach can significantly improve the accuracy of student performance prediction. By using this approach, universities can better recommend elective courses to their students as well as predict student performance, which can help them make informed decisions and achieve better academic outcomes.
Copyright © 2023 Thanh-Nhan Huynh-Ly et al., licensed to EAI. This is an open access article distributed under the terms of the CC BY-NC-SA 4.0, which permits copying, redistributing, remixing, transformation, and building upon the material in any medium so long as the original work is properly cited.