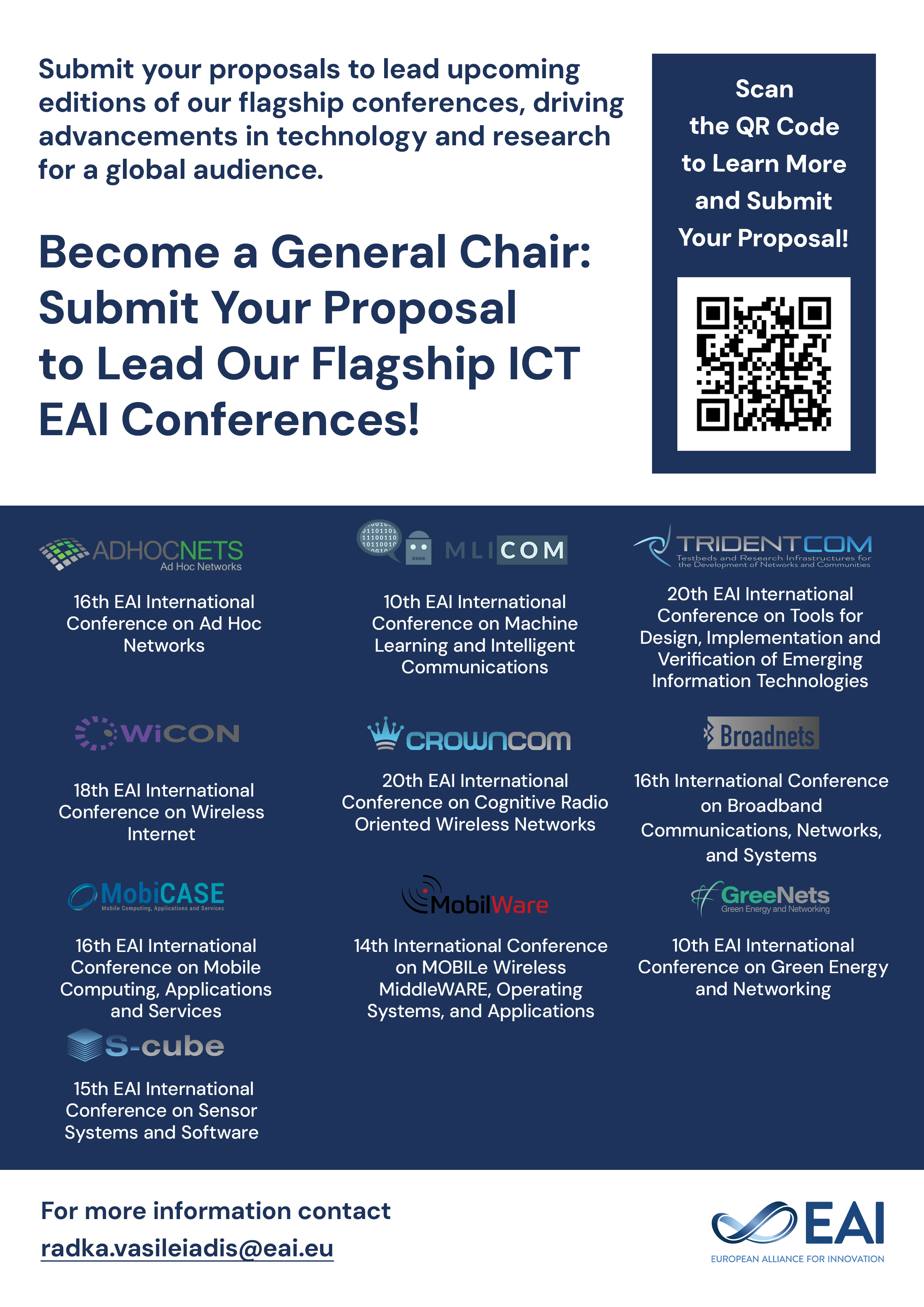
Research Article
Gradient Descent Machine Learning with Equivalency Testing for Non-Subject Dependent Applications in Human Activity Recognition
@ARTICLE{10.4108/eetcasa.v8i24.1996, author={T.A. Woolman and J.L. Pickard}, title={Gradient Descent Machine Learning with Equivalency Testing for Non-Subject Dependent Applications in Human Activity Recognition}, journal={EAI Endorsed Transactions on Context-aware Systems and Applications}, volume={8}, number={1}, publisher={EAI}, journal_a={CASA}, year={2022}, month={7}, keywords={Human activity recognition, digital sensor, telemetry, gradient boosting, gradient descent, machine learning, classification, statistical equivalence testing}, doi={10.4108/eetcasa.v8i24.1996} }
- T.A. Woolman
J.L. Pickard
Year: 2022
Gradient Descent Machine Learning with Equivalency Testing for Non-Subject Dependent Applications in Human Activity Recognition
CASA
EAI
DOI: 10.4108/eetcasa.v8i24.1996
Abstract
INTRODUCTION: A solution to subject-independent HAR prediction through machine learning classification algorithms using statistical equivalency for comparative analysis between independent groups with non-subject training dependencies. OBJECTIVES: To indicate that the multinomial predictive classification model that was trained and optimized on the one-subject control group is at least partially extensible to multiple independent experiment groups for at least one activity class. METHODS: Gradient boosted machine multinomial classification algorithm is trained on a single individual with the classifier trained on all activity classes as a multinomial classification problem. RESULTS: Levene-Wellek-Welch (LWW) Statistic calculated as 0.021, with a Critical Value for LWW of 0.026, using an alpha of 0.05. CONCLUSION: Confirmed falsifiability that incorporates reproducible methods into the quasi-experiment design applied to the field of machine learning for human activity recognition.
Copyright © 2022 T.A. Woolman et al., licensed to EAI. This is an open access article distributed under the terms of the Creative Commons Attribution license, which permits unlimited use, distribution and reproduction in any medium so long as the original work is properly cited.