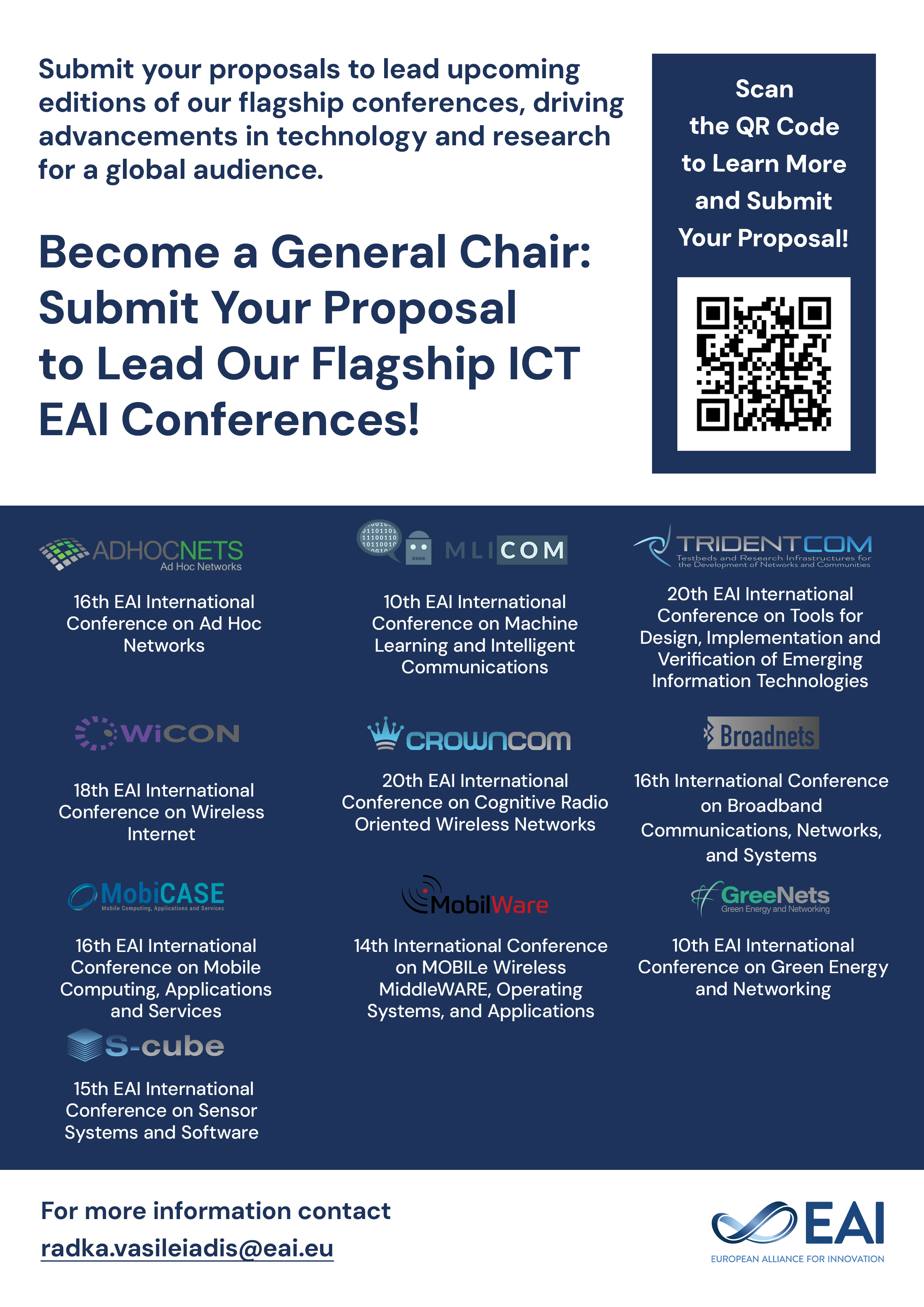
Research Article
Predicting Breast Cancer with Ensemble Methods on Cloud
@ARTICLE{10.4108/eetcasa.v8i2.2788, author={Au Pham and Tu Tran and Phuc Tran and Hiep Huynh}, title={Predicting Breast Cancer with Ensemble Methods on Cloud}, journal={EAI Endorsed Transactions on Context-aware Systems and Applications}, volume={9}, number={1}, publisher={EAI}, journal_a={CASA}, year={2023}, month={3}, keywords={Bagging, Boosting, Stacking, Random Forest, Ensemble methods}, doi={10.4108/eetcasa.v8i2.2788} }
- Au Pham
Tu Tran
Phuc Tran
Hiep Huynh
Year: 2023
Predicting Breast Cancer with Ensemble Methods on Cloud
CASA
EAI
DOI: 10.4108/eetcasa.v8i2.2788
Abstract
There are many dangerous diseases and high mortality rates for women (including breast cancer). If the disease is detected early, correctly diagnosed and treated at the right time, the likelihood of illness and death is reduced. Previous disease prediction models have mainly focused on methods for building individual models. However, these predictive models do not yet have high accuracy and high generalization performance. In this paper, we focus on combining these individual models together to create a combined model, which is more generalizable than the individual models. Three ensemble techniques used in the experiment are: Bagging; Boosting and Stacking (Stacking include three models: Gradient Boost, Random Forest, Logistic Regression) to deploy and apply to breast cancer prediction problem. The experimental results show the combined model with the ensemble methods based on the Breast Cancer Wisconsin dataset; this combined model has a higher predictive performance than the commonly used individual prediction models.
Copyright © 2023 Au Van Pham et al., licensed to EAI. This is an open access article distributed under the terms of the CC BY-NCSA 4.0, which permits copying, redistributing, remixing, transformation, and building upon the material in any medium so long as the original work is properly cited.