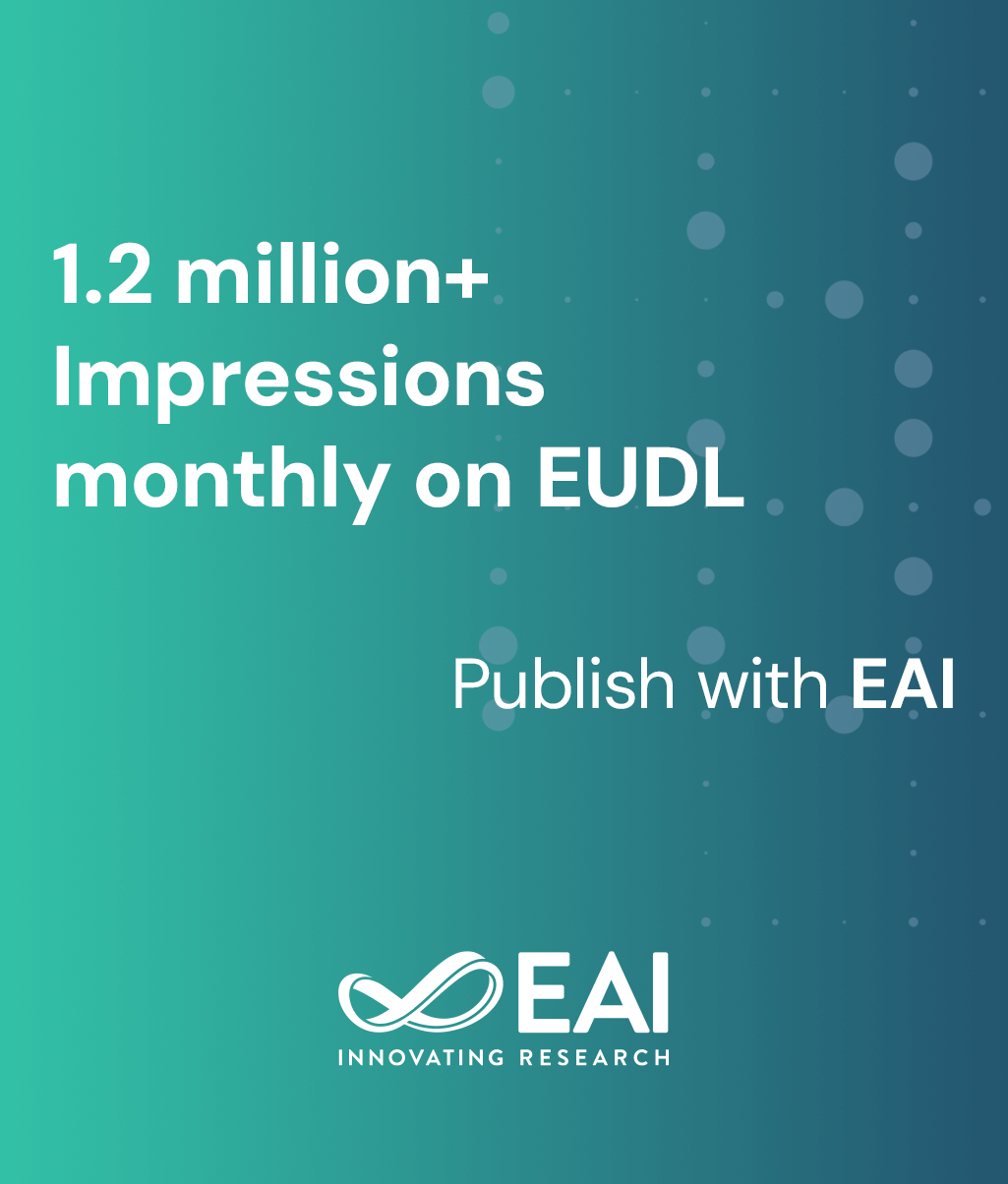
Research Article
UGGNet: Bridging U-Net and VGG for Advanced Breast Cancer Diagnosis
@ARTICLE{10.4108/eetcasa.v10i1.4681, author={Tran Cao Minh and Nguyen Kim Quoc and Phan Cong Vinh and Dang Nhu Phu and Vuong Xuan Chi and Ha Minh Tan}, title={UGGNet: Bridging U-Net and VGG for Advanced Breast Cancer Diagnosis}, journal={EAI Endorsed Transactions on Contex-aware Systems and Applications}, volume={10}, number={1}, publisher={EAI}, journal_a={CASA}, year={2024}, month={1}, keywords={Breast Cancer, Classification, Deep Learning, Segmentation, Ultrasonic Image}, doi={10.4108/eetcasa.v10i1.4681} }
- Tran Cao Minh
Nguyen Kim Quoc
Phan Cong Vinh
Dang Nhu Phu
Vuong Xuan Chi
Ha Minh Tan
Year: 2024
UGGNet: Bridging U-Net and VGG for Advanced Breast Cancer Diagnosis
CASA
EAI
DOI: 10.4108/eetcasa.v10i1.4681
Abstract
In the field of medical imaging, breast ultrasound has emerged as a crucial diagnostic tool for early detection of breast cancer. However, the accuracy of diagnosing the location of the affected area and the extent of the disease depends on the experience of the physician. In this paper, we propose a novel model called UGGNet, combining the power of the U-Net and VGG architectures to enhance the performance of breast ultrasound image analysis. The U-Net component of the model helps accurately segment the lesions, while the VGG component utilizes deep convolutional layers to extract features. The fusion of these two architectures in UGGNet aims to optimize both segmentation and feature representation, providing a comprehensive solution for accurate diagnosis in breast ultrasound images. Experimental results have demonstrated that the UGGNet model achieves a notable accuracy of 78.2\% on the "Breast Ultrasound Images Dataset."
Copyright © 2024 T. C. Minh et al., licensed to EAI. This is an open access article distributed under the terms of the CC BY-NC-SA 4.0, which permits copying, redistributing, remixing, transformation, and building upon the material in any medium so long as the original work is properly cited.