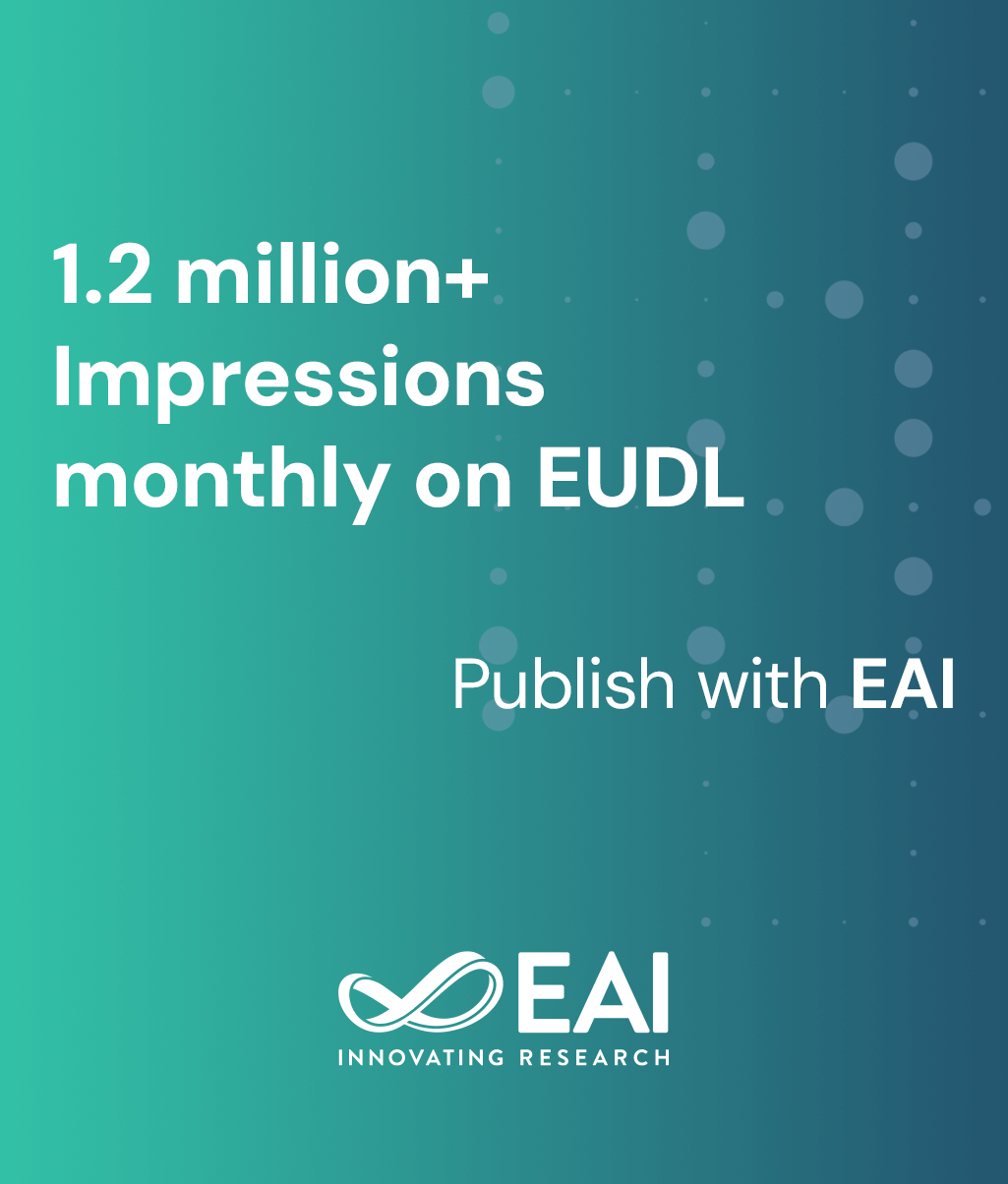
Research Article
Analysis and improvement of evaluation indexes for clustering results
@ARTICLE{10.4108/eai.9-10-2017.163211, author={Hao Zhong and Huibing Zhang and Fei Jia}, title={Analysis and improvement of evaluation indexes for clustering results}, journal={EAI Endorsed Transactions on Collaborative Computing}, volume={4}, number={13}, publisher={EAI}, journal_a={CC}, year={2020}, month={2}, keywords={evaluation indexes, Calinski-Harabasz Index, Davies-Bouldin Index, Silhouette Coefficient}, doi={10.4108/eai.9-10-2017.163211} }
- Hao Zhong
Huibing Zhang
Fei Jia
Year: 2020
Analysis and improvement of evaluation indexes for clustering results
CC
EAI
DOI: 10.4108/eai.9-10-2017.163211
Abstract
Clustering algorithm is the main field in collaborative computing of social network. How to evaluate clustering results accurately has become a hot spot in clustering algorithm research. Commonly used evaluation indexes are SC, DBI and CHI. There are two shortcomings in the calculation of three indexes. (1) Keep the number of clusters and the objects in the cluster unchanged. When transforming the feature vector, the three indexes will change greatly; (2) Keep the feature vector and the number of clusters unchanged. When changing the objects in the cluster, the three indexes will change tinily. This shows that the three indexes unable to evaluate the clustering results very well. Therefore, based on the calculation process of the three indexes, the paper proposes new three indexes - NSC, NDBI and NCHI. Through testing on standard data sets, three new indexes can better evaluate clustering results.
Copyright © 2020 Hao Zhong et al., licensed to EAI. This is an open access article distributed under the terms of the Creative Commons Attribution license (http://creativecommons.org/licenses/by/3.0/), which permits unlimited use, distribution and reproduction in any medium so long as the original work is properly cited.